5 GPTs for Hardware Testing Powered by AI for Free of 2025
AI GPTs for Hardware Testing refer to specialized applications of Generative Pre-trained Transformers that are optimized for tasks in the hardware testing domain. These tools leverage the advanced capabilities of AI to simulate, predict, and analyze hardware performance under various conditions, thus aiding in the development, troubleshooting, and optimization of hardware products. The integration of AI into hardware testing allows for more precise diagnostics, predictive maintenance, and efficient quality control.
Top 5 GPTs for Hardware Testing are: AVR Assembly Master,MicroPython Copilot,Isaac Sim Guide,Circuit SketcherDraw,Electrical and Computer Engineering
AVR Assembly Master
Optimize and debug AVR code efficiently.
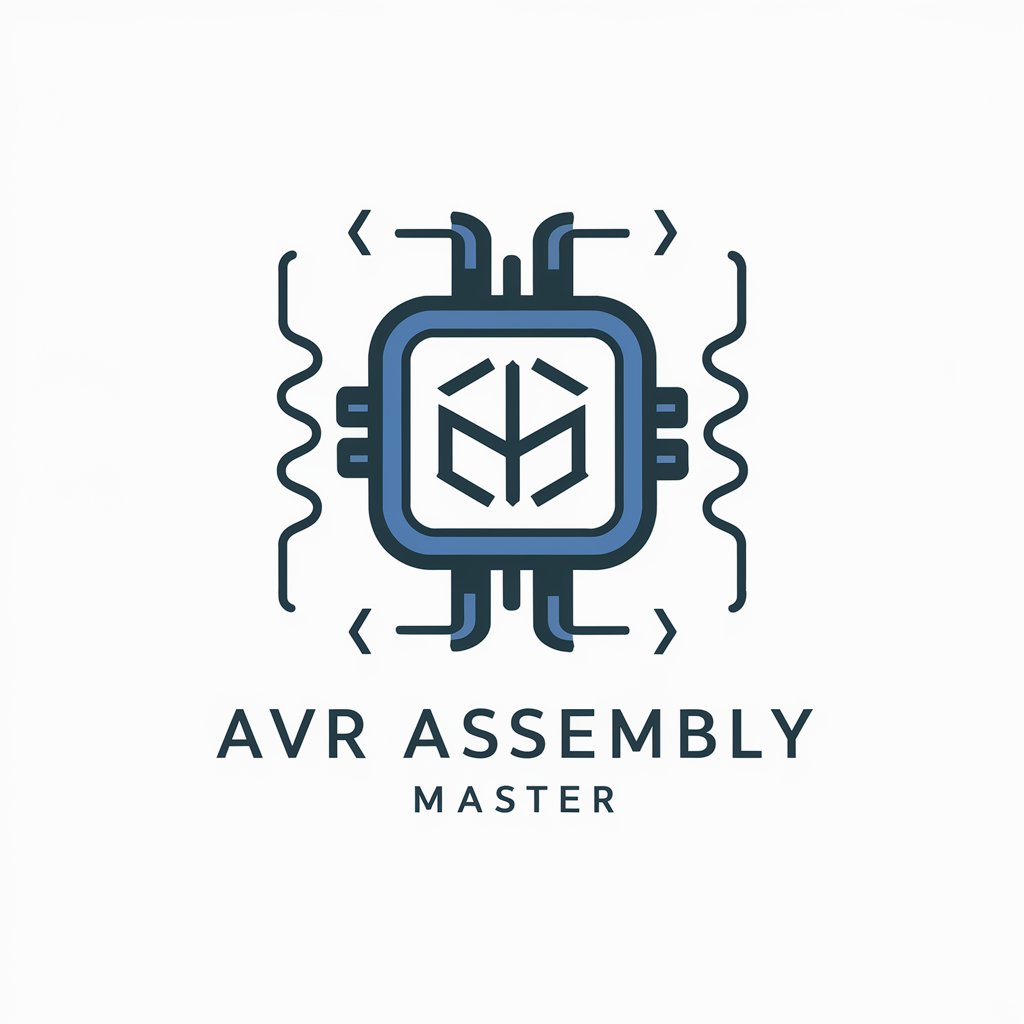
MicroPython Copilot
Streamline Embedded Projects with AI
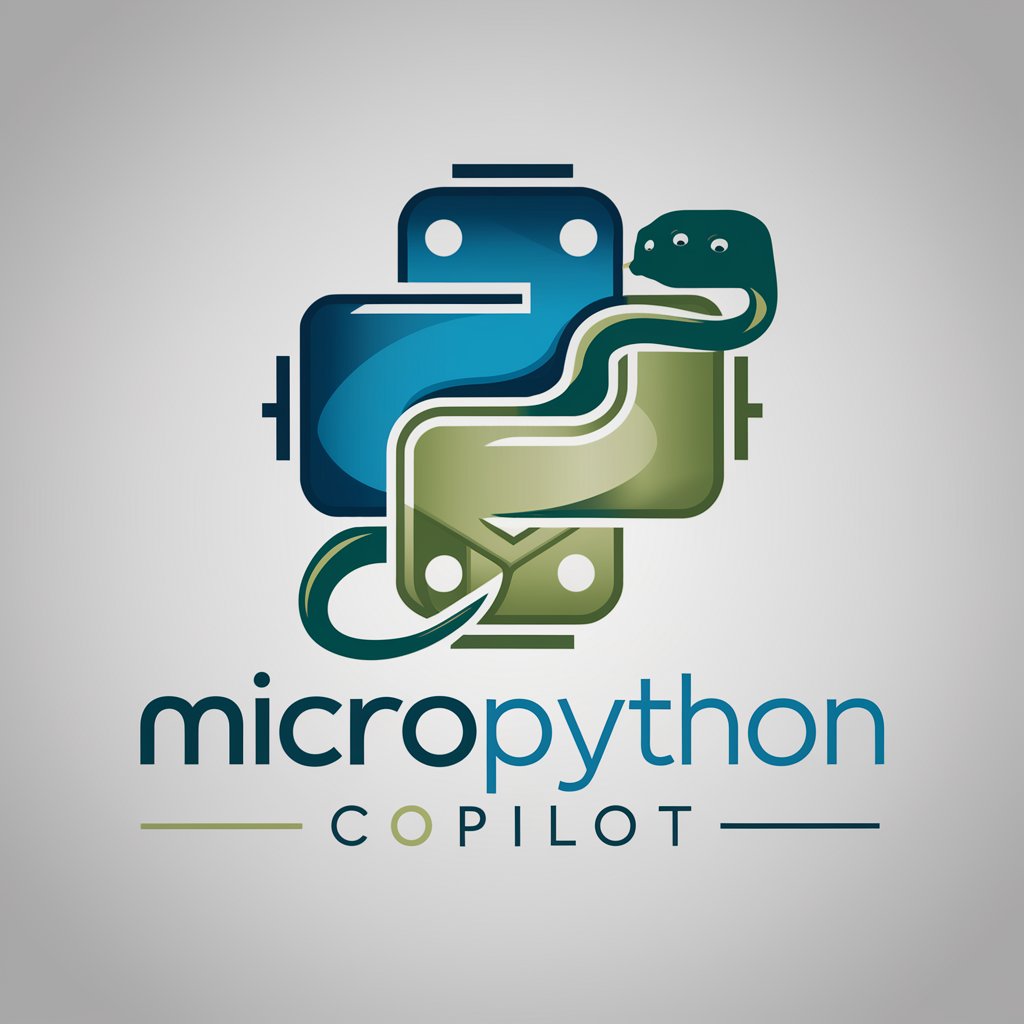
Isaac Sim Guide
Simulate. Integrate. Innovate.
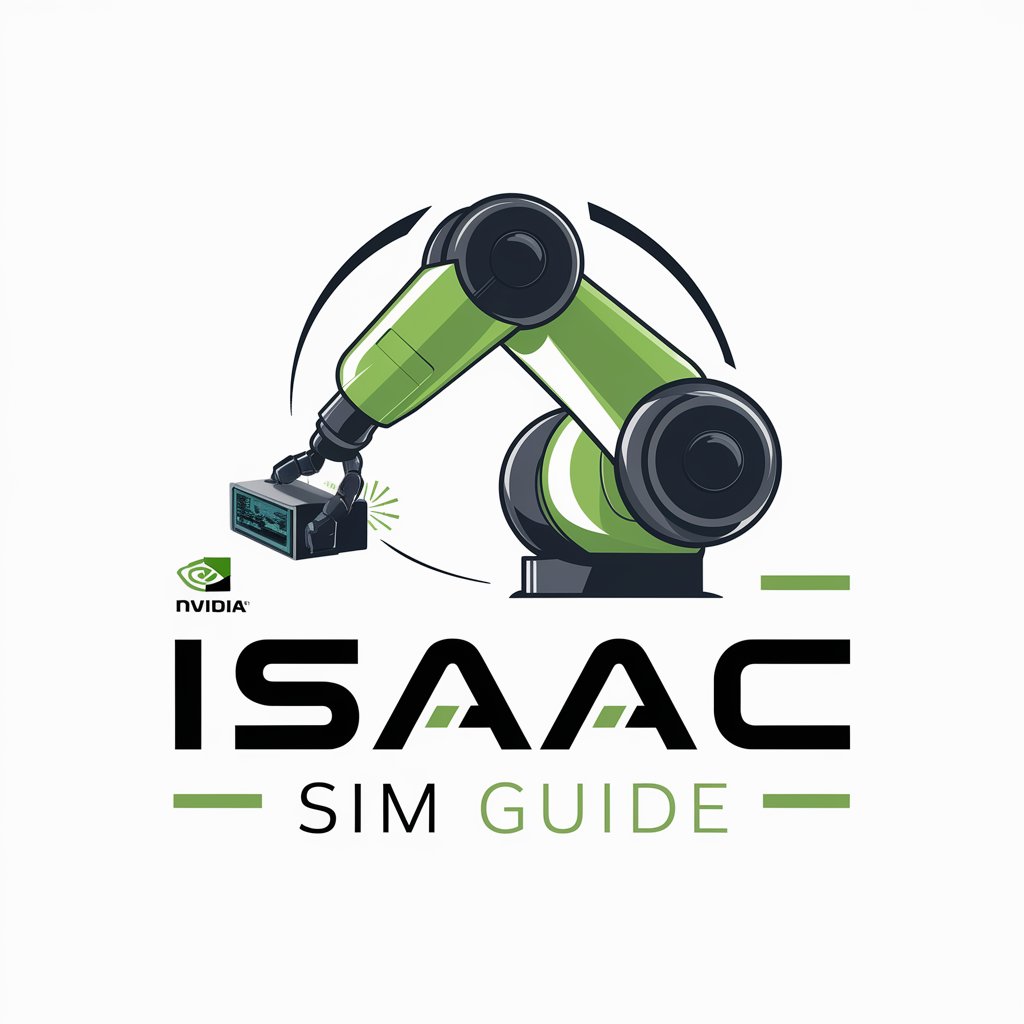
Circuit SketcherDraw
Visualize circuits from code, powered by AI.
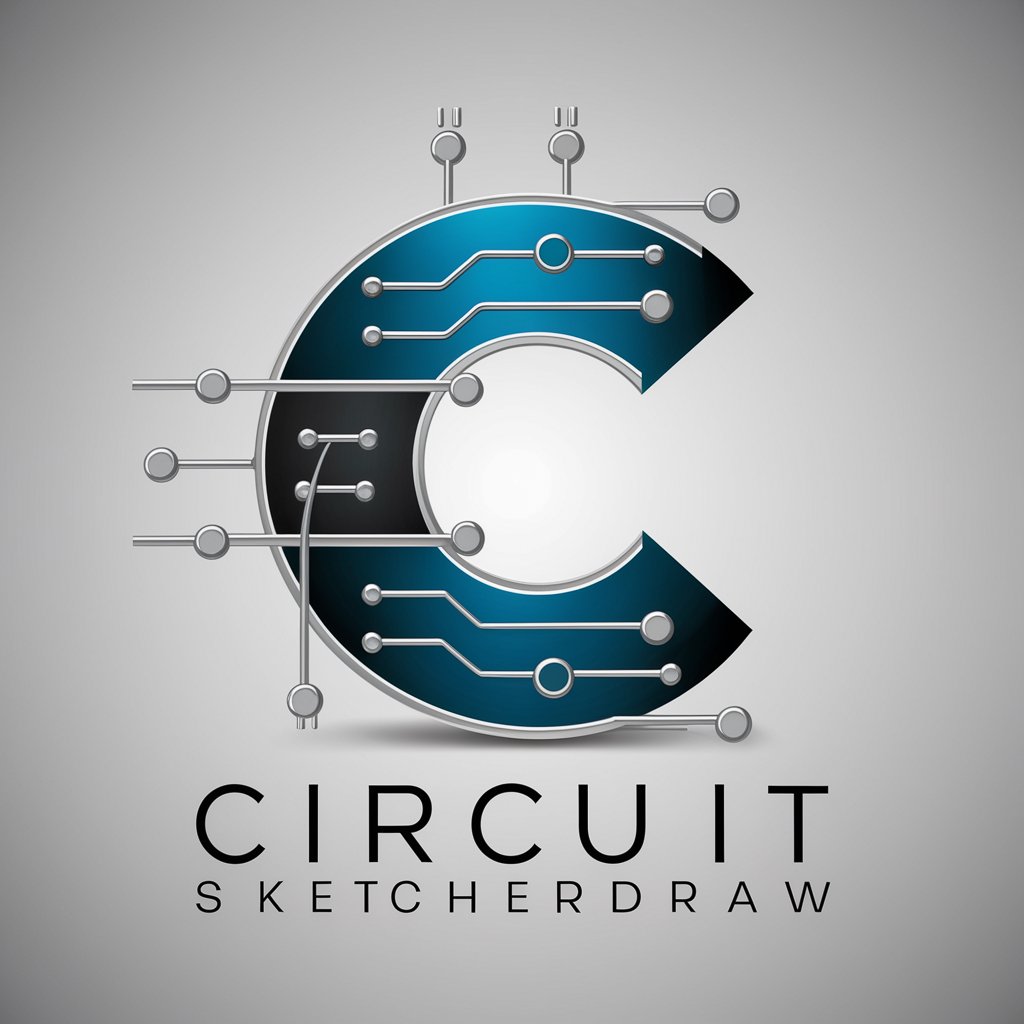
Electrical and Computer Engineering
Empowering Innovation with AI-Driven Engineering
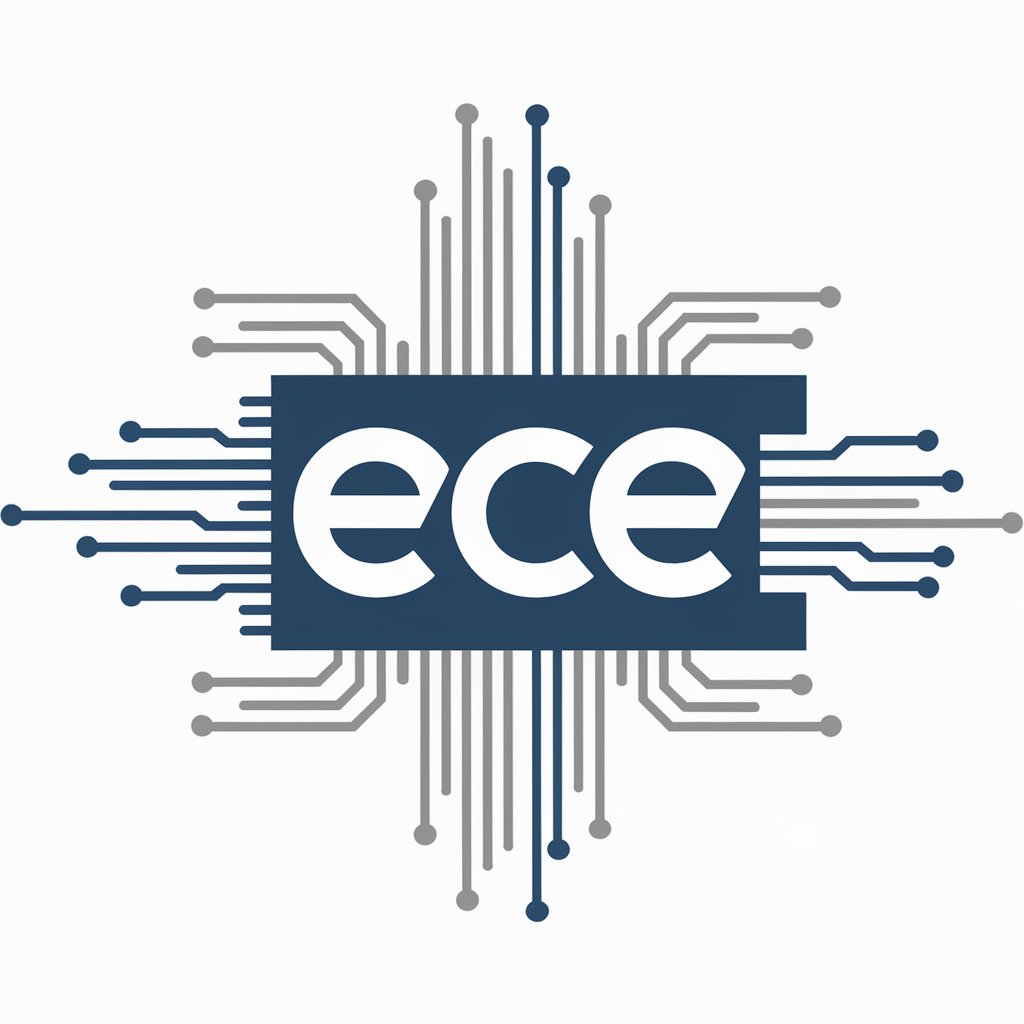
Essential Traits and Functions
AI GPTs for Hardware Testing are notable for their adaptability across different testing scenarios, from component-level evaluation to system-wide integration tests. Key features include automated test generation, real-time data analysis, fault detection, and predictive insights. These tools can adapt their functions from basic to advanced levels, catering to different aspects of hardware testing such as stress testing, performance benchmarking, and environmental impact simulation.
Who Benefits from AI GPTs in Hardware Testing
The primary users of these AI GPTs tools include hardware developers, quality assurance professionals, and maintenance engineers. They are also immensely beneficial for educational purposes, helping students and novices understand complex hardware interactions. Advanced users, like seasoned engineers, can leverage these tools for deep customization and integration into bespoke testing environments.
Try Our other AI GPTs tools for Free
Tech Tutoring
Explore AI-driven tech tutoring with GPTs: Tailored, interactive learning tools designed to enhance your technology education, regardless of your expertise level.
Document Proofing
Explore AI GPTs for Document Proofing: your essential tools for enhancing document quality with advanced editing, grammar checks, and style consistency. Perfect for professionals and novices alike.
Data Prediction
Explore AI GPT tools for Data Prediction, designed to transform data analysis with adaptable, user-friendly solutions for accurate forecasting and trend insights.
Professional Sabotage
Explore AI GPTs tailored for Professional Sabotage, designed to anticipate, analyze, and prevent disruptive actions in professional settings with cutting-edge technology.
Market Communication
Discover how AI GPT tools revolutionize Market Communication with adaptive AI technology for enhanced interaction and strategic content creation.
Photography Training
Explore the transformative potential of AI GPTs in Photography Training. These tools offer personalized learning experiences, technical guidance, and integration with professional tools, perfect for both novices and experts.
Further Reflections on AI GPTs
AI GPTs are transforming hardware testing by enabling more comprehensive and less intrusive testing procedures. Their integration into hardware testing not only streamlines workflows but also enhances the reliability and innovativeness of hardware solutions. With user-friendly interfaces, these tools are designed to be easily adopted and integrated into various testing scenarios.
Frequently Asked Questions
What are AI GPTs for Hardware Testing?
AI GPTs for Hardware Testing are AI-driven tools designed to assist in the evaluation, analysis, and optimization of hardware devices through advanced data processing and predictive modeling capabilities.
How can AI GPTs improve hardware testing processes?
By automating routine tests, predicting hardware failures before they occur, and optimizing test parameters based on real-time data, AI GPTs enhance both the efficiency and accuracy of hardware testing.
Who can use AI GPTs for Hardware Testing?
These tools are accessible to a wide range of users from hardware testing novices to experienced engineers and developers, providing both simple interfaces for beginners and advanced options for professionals.
Can AI GPTs be integrated with existing testing frameworks?
Yes, AI GPTs can be seamlessly integrated with existing hardware testing frameworks to enhance their capabilities and introduce new functionalities like predictive maintenance and automated fault detection.
Are there customization options available for AI GPTs in Hardware Testing?
Absolutely, users can customize these tools to suit specific hardware configurations, testing requirements, and performance benchmarks, making them a versatile addition to any hardware development and testing lab.
What makes AI GPTs unique in hardware testing?
AI GPTs stand out due to their ability to learn from data, predict outcomes, and provide actionable insights, which are crucial for making informed decisions during the hardware development lifecycle.
Is programming knowledge required to use AI GPTs in Hardware Testing?
Basic usage does not require programming skills, though having them can help users take full advantage of the tool's advanced features and customization options.
How does AI GPTs ensure the reliability of hardware testing?
AI GPTs employ sophisticated algorithms to analyze test results and historical data, thereby increasing the reliability of predictions and ensuring higher standards of hardware performance and safety.