4 GPTs for Environmental Forecasting Powered by AI for Free of 2025
AI GPTs for Environmental Forecasting are advanced tools that leverage the power of Generative Pre-trained Transformers to analyze, predict, and provide insights into various environmental conditions. These AI models are trained on vast datasets to recognize patterns and predict outcomes, making them invaluable for tasks such as weather forecasting, climate change analysis, and environmental risk assessment. Their relevance lies in their ability to process complex environmental data and offer predictions or solutions, tailored to support sustainable development and disaster mitigation efforts.
Top 4 GPTs for Environmental Forecasting are: Global Construction Oracle,Manoa Ideator,未来スコープ,Immo Urbain by ImmoSaas
Global Construction Oracle
Revolutionizing Construction with AI-Powered Innovation
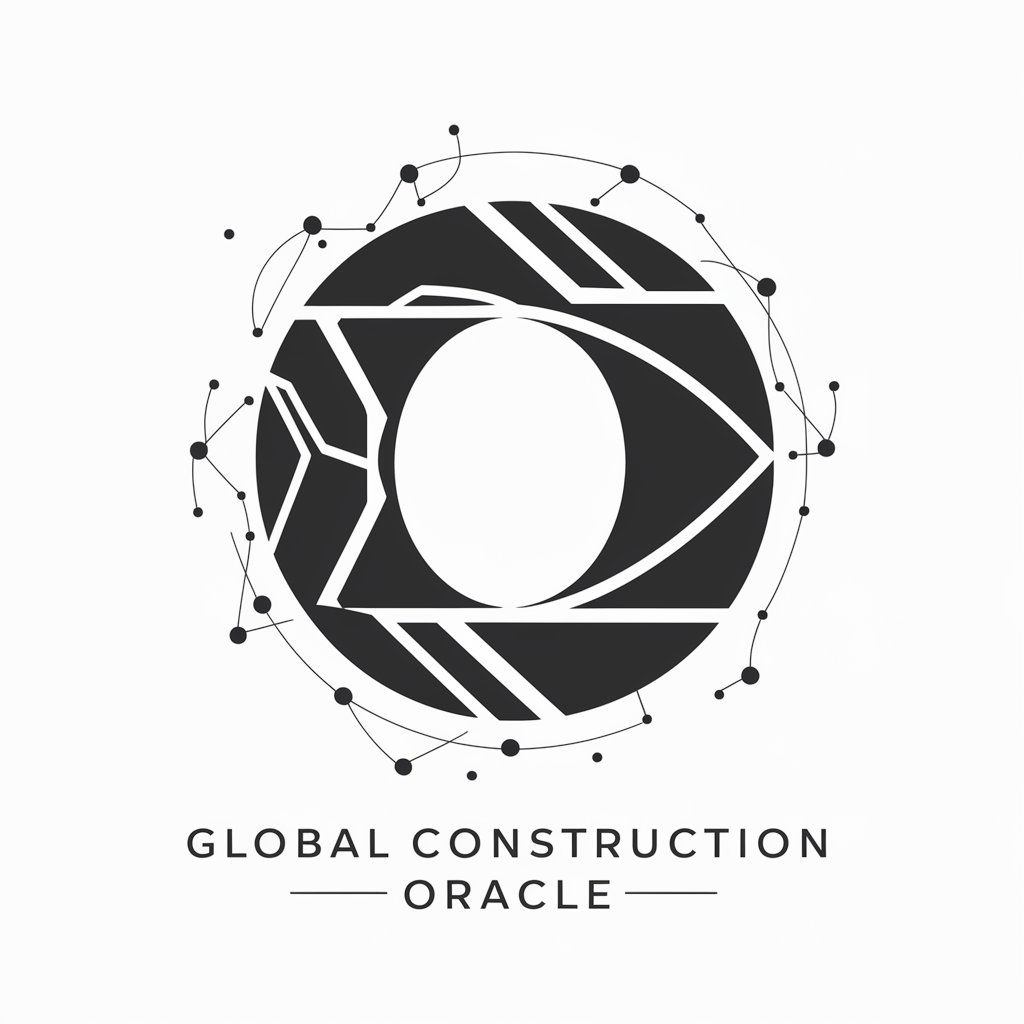
Manoa Ideator
Envisioning Tomorrow, Empowering Today
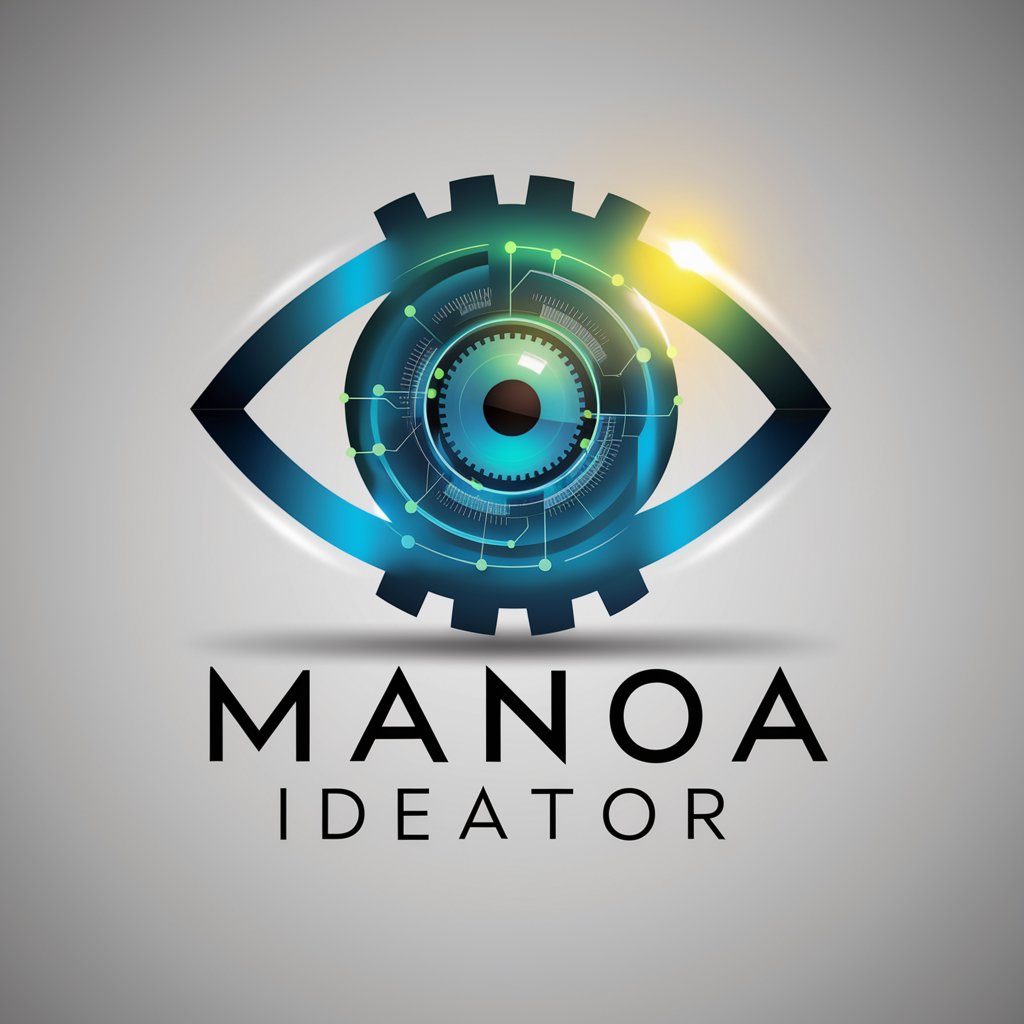
未来スコープ
Predicting tomorrow with AI today.
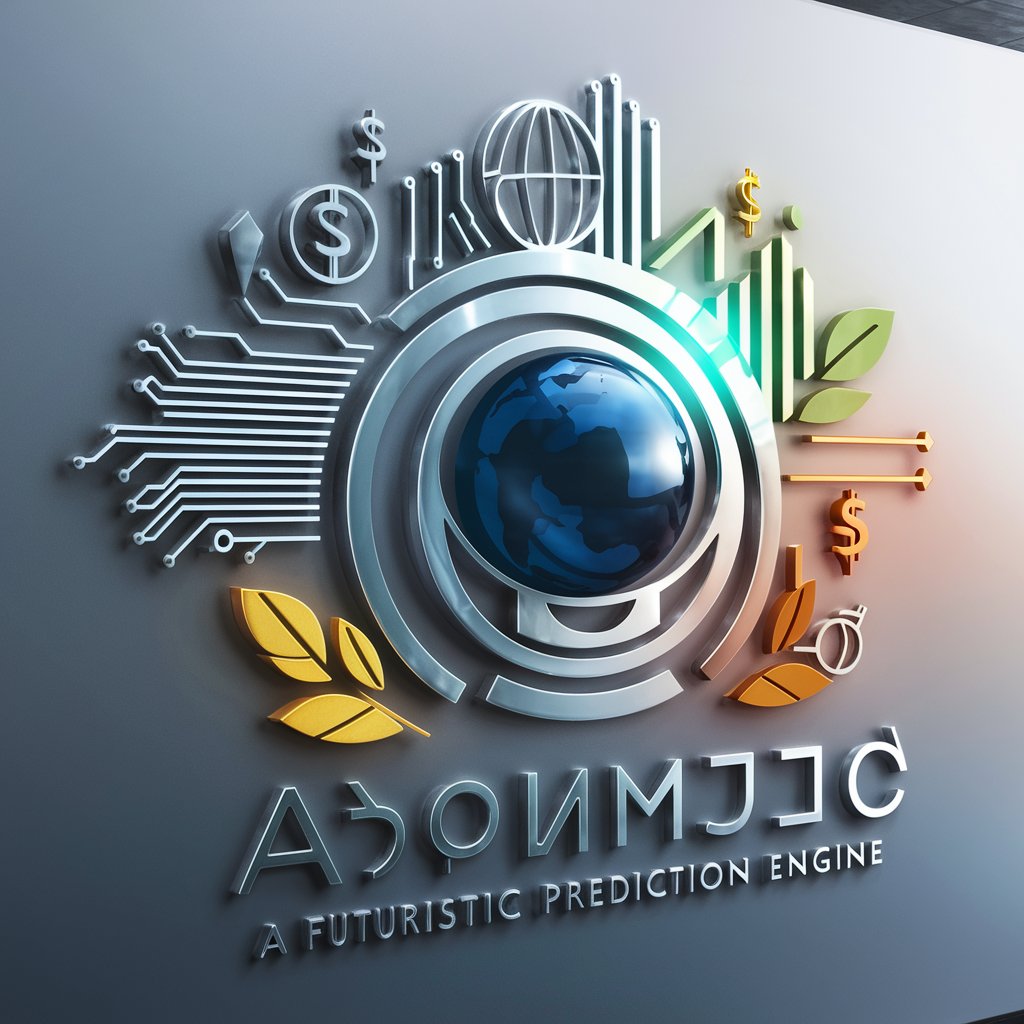
Immo Urbain by ImmoSaas
Empowering Urban Evolution with AI

Key Attributes and Functionalities
AI GPTs designed for Environmental Forecasting boast a range of unique features that set them apart. These include the ability to process and analyze large datasets, predict environmental trends, and generate reports or recommendations. They can adapt from simple forecasting models to complex simulations of environmental scenarios. Special features might include natural language processing for generating readable reports, technical support for integrating with existing environmental databases, and data analysis capabilities for identifying long-term trends.
Who Can Benefit
These tools are ideal for a wide range of users, from environmental science novices to experienced professionals. They offer an accessible entry point for those new to environmental forecasting, with intuitive interfaces and guidance. For developers and environmental scientists, they provide powerful customization options, allowing users to tailor models to specific forecasting needs. This accessibility opens up possibilities for educators, researchers, and policy-makers, facilitating informed decision-making in environmental planning and conservation.
Try Our other AI GPTs tools for Free
Lead Management
Discover AI GPTs for Lead Management: revolutionary tools designed to automate, optimize, and personalize your lead management process, enhancing efficiency and engagement.
DesignScript Development
Unlock the potential of computational design with AI GPTs for DesignScript Development, your intelligent assistant for automating tasks, optimizing workflows, and enhancing creativity in the design process.
Graph Interpretation
Explore AI GPTs for Graph Interpretation: Unleash the power of AI to analyze and understand complex graphs and networks with ease, making sophisticated data analysis accessible to all.
Learning React
Explore AI GPT tools for Learning React - your gateway to mastering React with personalized learning experiences, technical support, and real-world application guidance.
Art Inspiration
Discover how AI GPTs for Art Inspiration can transform your creative process with innovative ideas and visual concepts. Ideal for artists and designers of all skill levels.
Listening Practice
Discover AI GPTs for Listening Practice: innovative tools designed to enhance your listening skills through tailored exercises, real-time feedback, and a personalized learning journey.
Enhanced Perspectives on AI Solutions
AI GPTs for Environmental Forecasting redefine how we approach environmental analysis and planning. Their ability to digest complex data sets and offer precise forecasts presents a significant advantage. The user-friendly interfaces of these tools democratize access to advanced forecasting, while integration capabilities ensure that they can enhance existing workflows. This marks a shift towards more data-driven, accurate environmental management strategies, empowering stakeholders across various sectors.
Frequently Asked Questions
What exactly are AI GPTs for Environmental Forecasting?
They are AI models trained to predict environmental conditions, analyze climate data, and assist in sustainable planning and disaster response, using vast amounts of data to make accurate forecasts.
Who can use these tools?
Anyone from novices in environmental studies to seasoned professionals can use these tools. They're designed to be accessible yet offer deep customization for expert users.
Can these tools predict all types of environmental changes?
While highly effective at analyzing trends and making forecasts, their accuracy depends on the quality of data and the specific environmental variables involved.
Do I need programming skills to use these tools?
No, many GPTs for Environmental Forecasting are designed with user-friendly interfaces for those without coding experience, though programming skills can enhance customization.
How do GPTs process environmental data?
They use machine learning algorithms to analyze historical and real-time data, identifying patterns and predicting future conditions based on those patterns.
Can these tools integrate with existing environmental systems?
Yes, many of these tools are designed to be compatible with existing databases and systems, allowing for seamless integration and data analysis.
What makes AI GPTs different from traditional forecasting models?
AI GPTs can process vast amounts of data more efficiently and make predictions not just based on historical trends but also by understanding complex relationships within the data.
Are the forecasts provided by these tools reliable?
Forecasts are based on current and historical data and are generally reliable, but users should consider potential variables and uncertainties in environmental conditions.