5 GPTs for Cluster Optimization Powered by AI for Free of 2024
AI GPTs for Cluster Optimization refer to advanced Generative Pre-trained Transformer models specifically engineered for enhancing efficiency and performance in cluster computing environments. These tools leverage the power of AI to analyze, predict, and optimize the distribution of workloads across a computing cluster. By doing so, they significantly improve resource utilization, reduce latency, and enhance the overall performance of distributed computing tasks. Their relevance lies in their ability to provide tailored solutions that adapt to the varying demands of cluster computing, making them indispensable in scenarios where computational resources are distributed across multiple nodes.
Top 5 GPTs for Cluster Optimization are: Kubernetes Navigator,K8s Assistant,Missing Cluster Identification Agent,Kubernetes Assistant,Hadoop Helper
Kubernetes Navigator
AI-powered Kubernetes Guidance
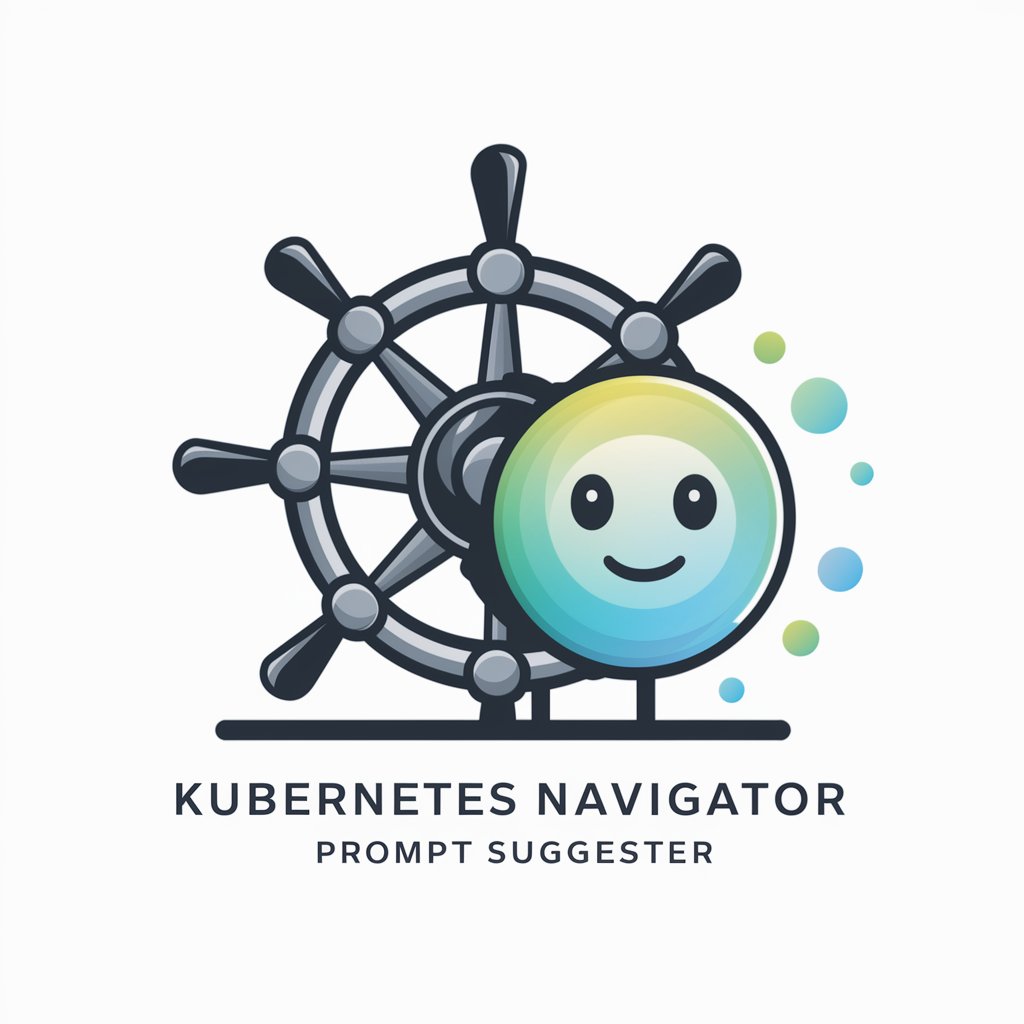
K8s Assistant
Unlocking Kubernetes Potential with AI
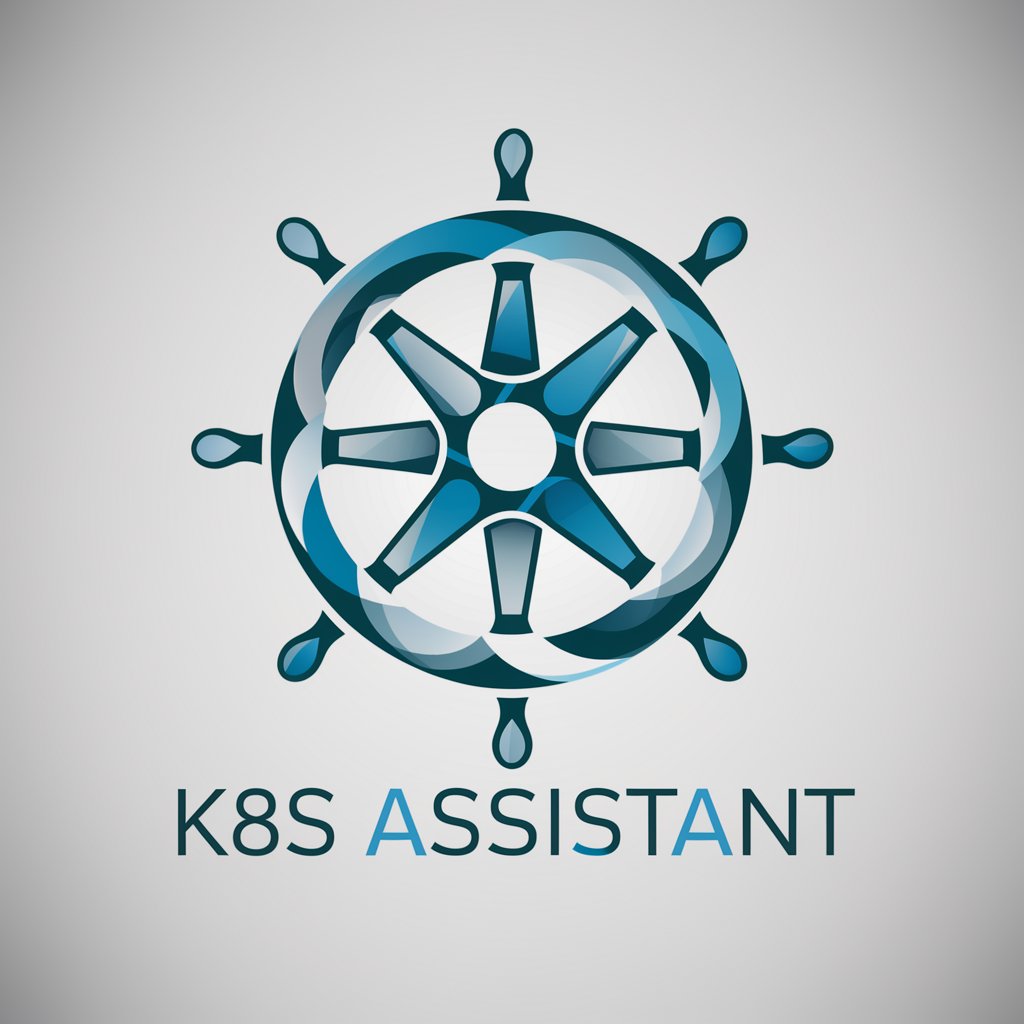
Missing Cluster Identification Agent
AI-powered Cluster Refinement
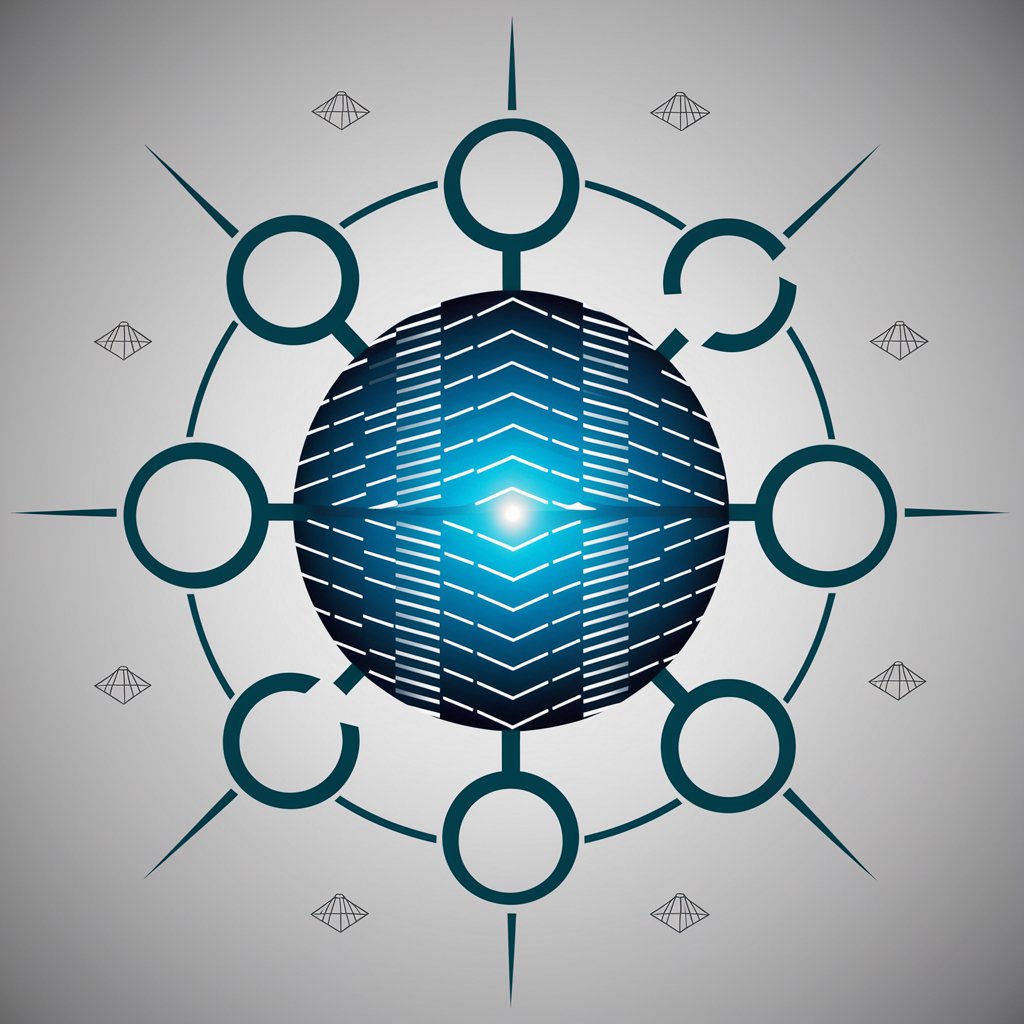
Kubernetes Assistant
Streamlining Kubernetes with AI
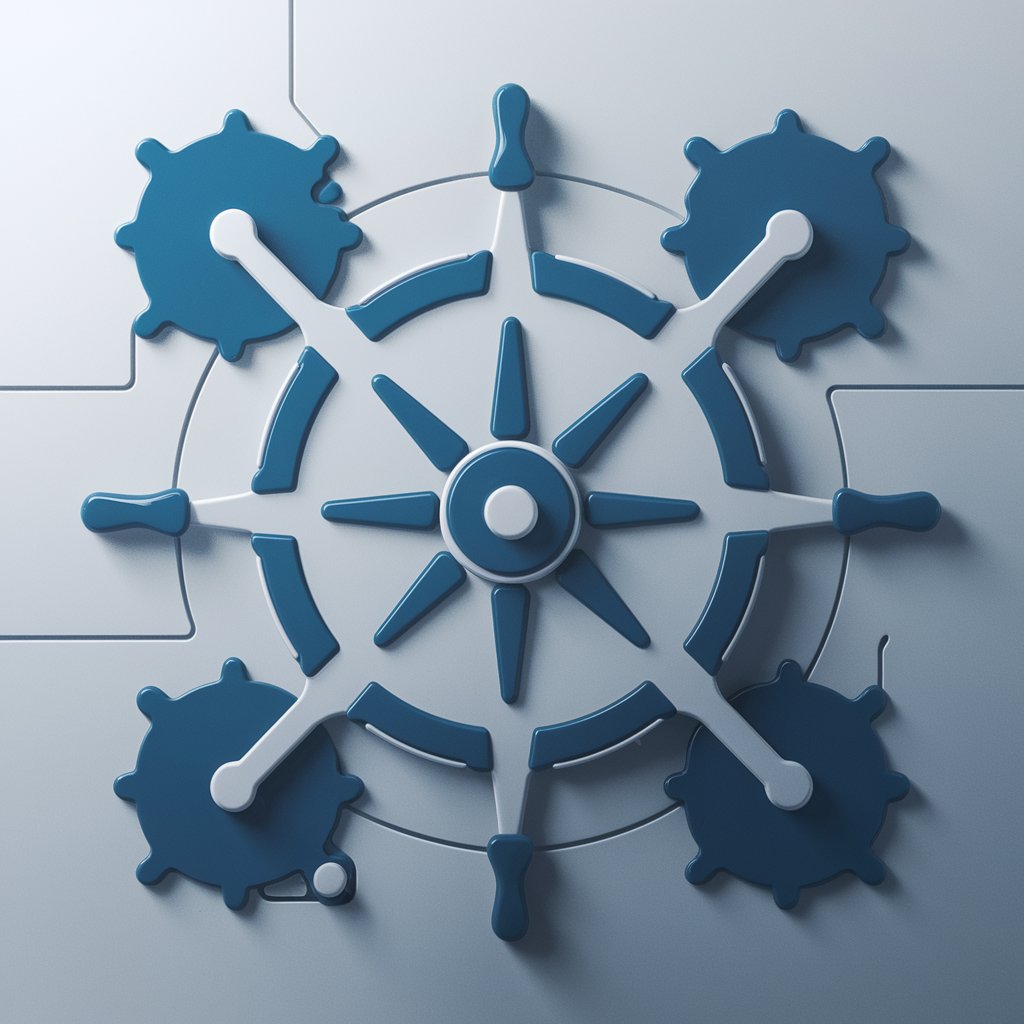
Hadoop Helper
AI-powered Hadoop expertise at your fingertips

Distinctive Capabilities of AI GPTs in Cluster Optimization
These AI GPTs tools stand out for their adaptability and the breadth of functions they cover, from real-time monitoring to predictive analytics for workload distribution. Key features include dynamic workload balancing, predictive resource allocation based on machine learning models, automated anomaly detection, and root cause analysis for system inefficiencies. Moreover, they can be customized to suit various cluster configurations and computing needs, from simple batch processing to complex data analytics workflows. Their advanced language models also support natural language processing for intuitive interaction, offering both technical and non-technical users a powerful tool for cluster management.
Who Can Benefit from AI GPTs in Cluster Optimization
The primary beneficiaries of AI GPTs for Cluster Optimization include IT professionals, system administrators, data scientists, and developers working with large-scale distributed computing environments. These tools are also highly accessible to novices in the field, providing user-friendly interfaces that simplify complex optimization tasks. For those with programming skills, these GPTs offer extensive customization options, allowing users to tailor the AI's capabilities to their specific needs and integrate them seamlessly into existing workflows.
Try Our other AI GPTs tools for Free
Archaeological Exploration
Discover how AI GPTs are revolutionizing Archaeological Exploration, offering advanced tools for site prediction, text analysis, and artifact recognition.
Jewelry Design
Discover how AI GPTs for Jewelry Design are revolutionizing the industry, offering creative solutions, streamlined processes, and unparalleled customization options for designers.
Gem Identification
Discover AI-powered Gem Identification tools, leveraging advanced GPT technology for accurate, efficient gemstone analysis. Ideal for professionals and enthusiasts alike.
Gemology Education
Discover the future of gemology education with AI GPTs, your gateway to interactive learning, advanced analysis, and personalized educational journeys in the fascinating world of gemstones.
Hobbyist Exploration
Discover how AI GPTs for Hobbyist Exploration can transform your hobbies with customized solutions, from art and coding to gaming and more.
Newsletter Engagement
Elevate your newsletter strategy with AI GPTs. Tailor content, analyze engagement, and optimize your outreach for maximum reader interaction.
Expanding the Capabilities of Cluster Computing with AI GPTs
AI GPTs for Cluster Optimization represent a paradigm shift in managing distributed computing resources. With their advanced analytics, predictive capabilities, and intuitive interfaces, these tools not only streamline cluster management but also pave the way for innovative applications in data analysis, machine learning, and beyond. Their integration flexibility further enhances their value, allowing for seamless adoption in diverse computing environments and sectors.
Frequently Asked Questions
What are AI GPTs for Cluster Optimization?
AI GPTs for Cluster Optimization are specialized AI models designed to improve the efficiency and performance of distributed computing environments through advanced analytics and machine learning.
How do these tools improve cluster performance?
They analyze and optimize workload distribution, predict resource requirements, and automatically adjust to changing demands, ensuring optimal resource utilization and reduced latency.
Can non-technical users utilize these GPTs effectively?
Yes, with user-friendly interfaces and natural language processing capabilities, these tools are accessible to users without coding skills, simplifying complex optimization tasks.
What customization options are available for developers?
Developers can tailor the AI's functionalities to specific cluster configurations and integrate the tools with existing systems, leveraging APIs and custom scripting.
Are these tools applicable to any cluster configuration?
Yes, they are designed to be adaptable to various configurations, from small clusters to large distributed computing environments.
How do AI GPTs handle dynamic changes in workload?
Through real-time monitoring and predictive analytics, they dynamically allocate resources and adjust workloads to maintain efficiency under changing conditions.
What are the benefits of predictive resource allocation?
Predictive allocation prevents resource bottlenecks, ensures balanced workloads, and reduces the risk of system overloads, leading to smoother and more efficient operations.
Can these tools integrate with existing monitoring systems?
Yes, they can be integrated with existing monitoring and management systems to provide a comprehensive view of cluster performance and optimization opportunities.