Analytics - Data Insights & Analysis
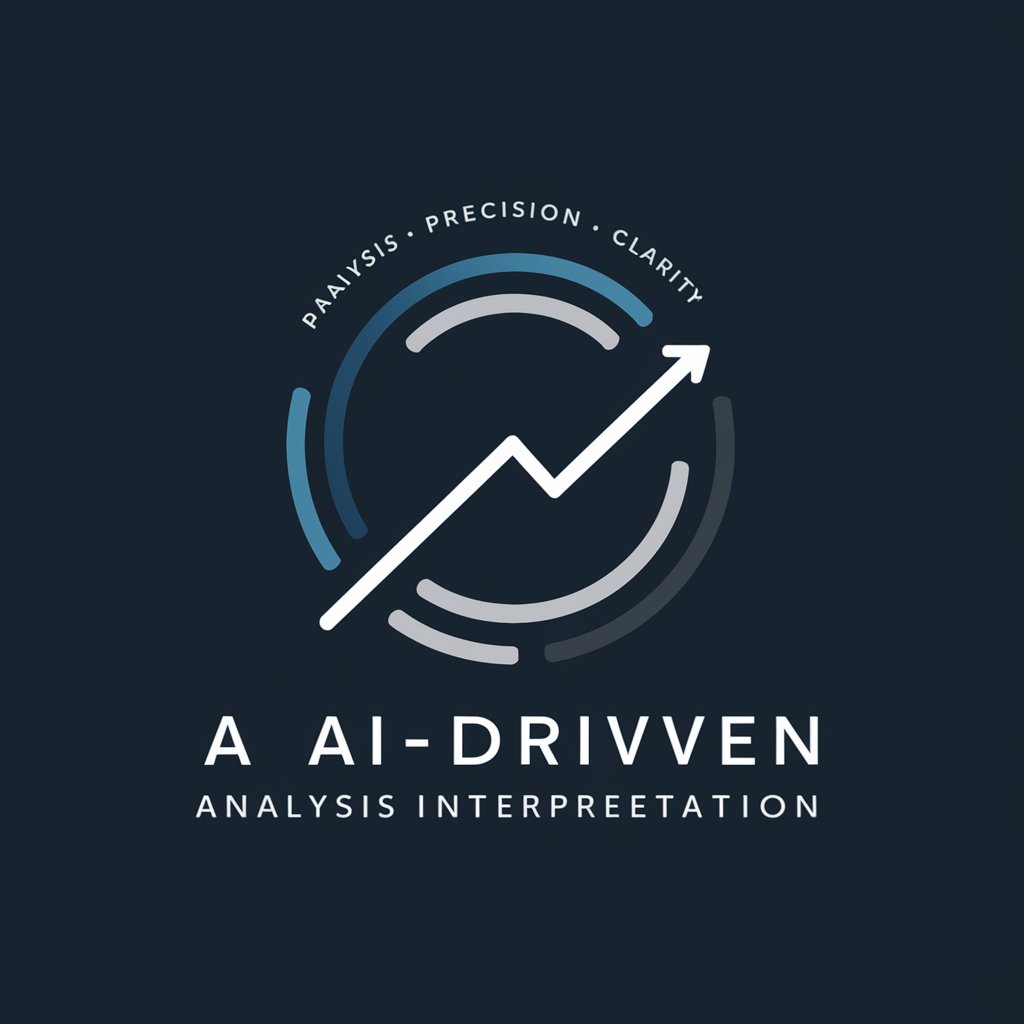
Welcome! How can I assist with your data analytics needs today?
Empowering Decisions with AI-Powered Analytics
Explain the process of conducting a regression analysis.
What are the key differences between supervised and unsupervised learning?
How can I interpret the results of a hypothesis test?
Describe the steps involved in data cleaning and preprocessing.
Get Embed Code
Introduction to Analytics
Analytics is designed as a specialized GPT with a focus on data analytics, providing technical expertise and precise analytical responses related to numbers, statistics, data interpretation, and analytical methodologies. Its primary design purpose is to assist users in making informed decisions based on data-driven insights, optimizing the use of data for strategic advantage. This involves interpreting complex datasets, identifying trends, performing statistical analysis, and applying various data analysis techniques to uncover meaningful patterns. For example, in a business context, Analytics can analyze sales data to identify seasonal trends and predict future sales, or in a healthcare setting, it might analyze patient data to identify the effectiveness of different treatment plans. Powered by ChatGPT-4o。
Main Functions of Analytics
Statistical Analysis
Example
Using regression analysis to determine the relationship between marketing spend and sales revenue.
Scenario
A business wants to optimize its marketing budget by understanding which activities drive sales. Analytics applies statistical techniques to analyze historical data, helping the business allocate resources more efficiently.
Predictive Modeling
Example
Creating a model to predict customer churn based on engagement metrics and historical data.
Scenario
A subscription-based company wants to reduce churn. Analytics uses machine learning algorithms to predict which customers are most likely to cancel their subscriptions, enabling targeted retention strategies.
Data Visualization
Example
Designing interactive dashboards that track key performance indicators (KPIs) in real-time.
Scenario
An operations manager needs to monitor manufacturing performance. Analytics creates dashboards that visualize production data, identifying bottlenecks and opportunities for process improvement.
Data Mining
Example
Extracting patterns from large datasets to identify customer buying behaviors.
Scenario
An e-commerce platform seeks to enhance its recommendation engine. Analytics employs data mining techniques to analyze transaction data, improving personalized recommendations for users.
Sentiment Analysis
Example
Analyzing customer reviews and social media to gauge public sentiment towards a brand.
Scenario
A brand manager wants to understand consumer perception after a product launch. Analytics applies natural language processing to analyze customer feedback, providing insights into brand sentiment and areas for improvement.
Ideal Users of Analytics Services
Business Analysts
Professionals who need to interpret data to make strategic business decisions. They benefit from using Analytics by gaining deeper insights into market trends, customer behavior, and financial performance, enabling data-driven decision-making.
Data Scientists
Experts in statistical analysis and machine learning who require advanced tools for predictive modeling, data mining, and complex data analysis. Analytics enhances their ability to develop sophisticated models and uncover hidden patterns in data.
Marketing Professionals
Individuals who need to understand customer preferences and the impact of marketing campaigns. Analytics helps them analyze customer data and campaign performance, optimizing marketing strategies for better engagement and ROI.
Healthcare Researchers
Researchers analyzing patient data to improve treatment outcomes and healthcare policies. Analytics aids in identifying trends in patient data, assessing treatment effectiveness, and supporting evidence-based medical research.
Educational Institutions
Schools and universities analyzing data to improve educational outcomes and operational efficiency. Analytics enables them to analyze student performance, optimize curriculum development, and manage resources effectively.
How to Use Analytics Effectively
Start Your Journey
Begin by visiting yeschat.ai to explore the platform's capabilities through a free trial, requiring no login or subscription to ChatGPT Plus.
Identify Your Analytical Goals
Clearly define what you aim to achieve with analytics, such as improving business decisions, understanding customer behavior, or enhancing operational efficiency.
Gather and Prepare Your Data
Collect relevant data from various sources. Ensure it's clean and structured to facilitate accurate analysis.
Analyze Your Data
Utilize analytics tools to examine your data. Apply statistical models and techniques to uncover trends, patterns, and insights.
Apply Insights to Decision-Making
Use the insights gained from your analysis to inform business strategies, make informed decisions, and measure the impact of changes.
Try other advanced and practical GPTs
Statistics Tutor
Demystifying Statistics with AI
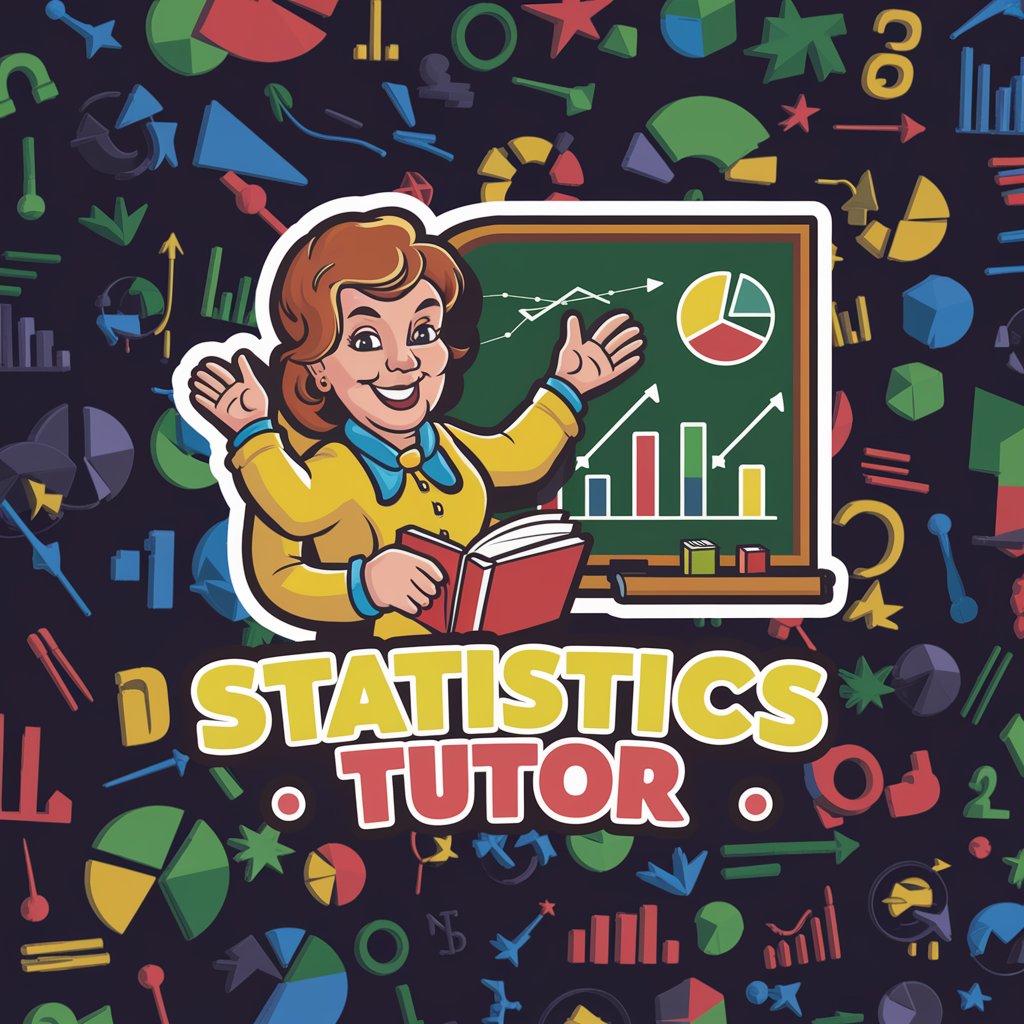
Ultimate Business Advisor
Empowering Your Business with AI-driven Advice
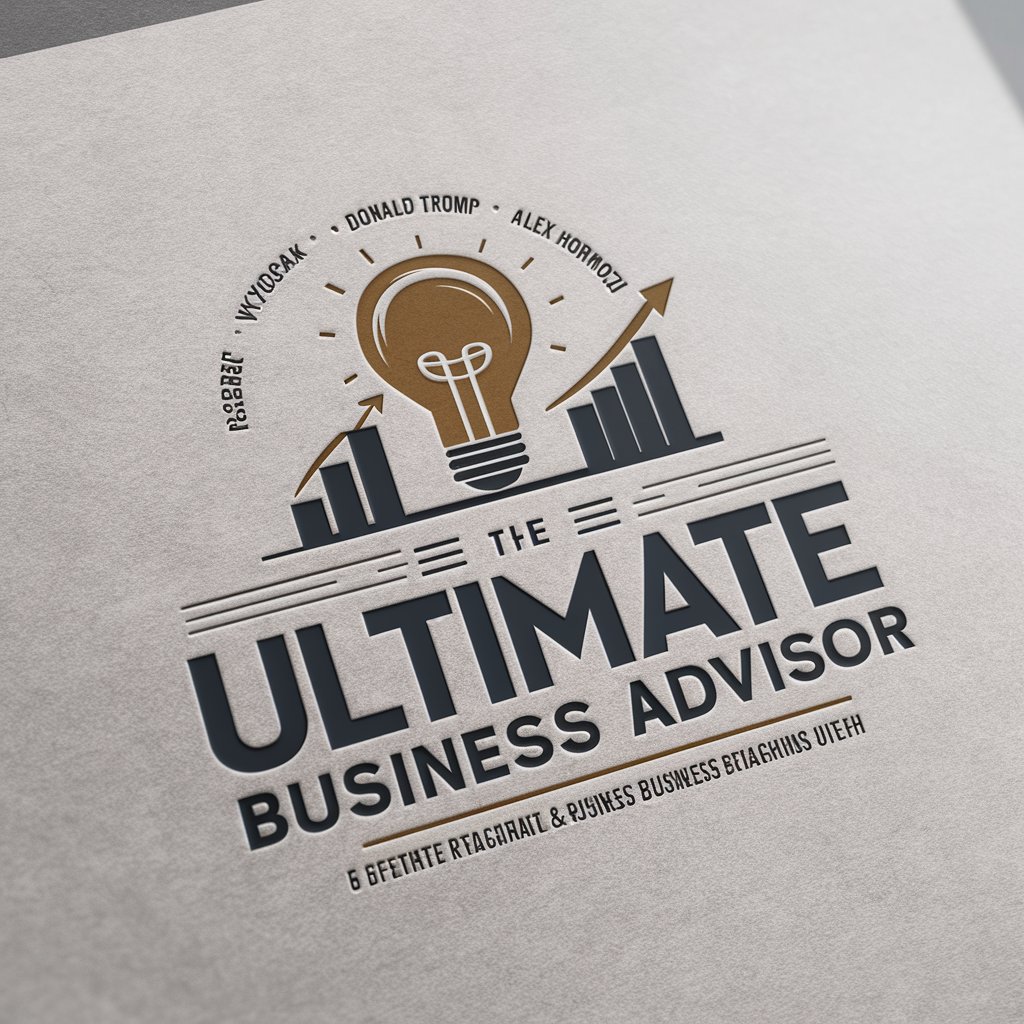
flick wiki
Discover your next favorite film, powered by AI
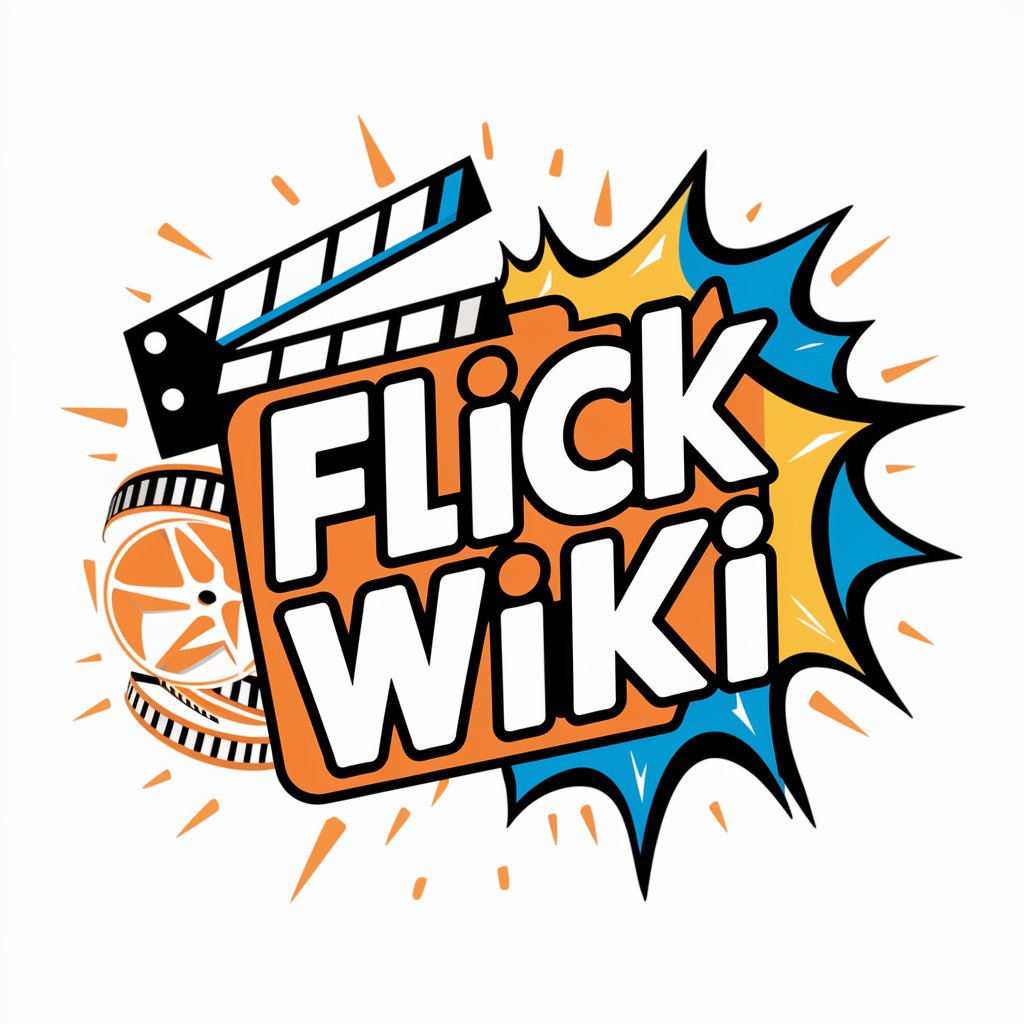
CinemaScope
Empowering Filmmakers with AI-driven Insights
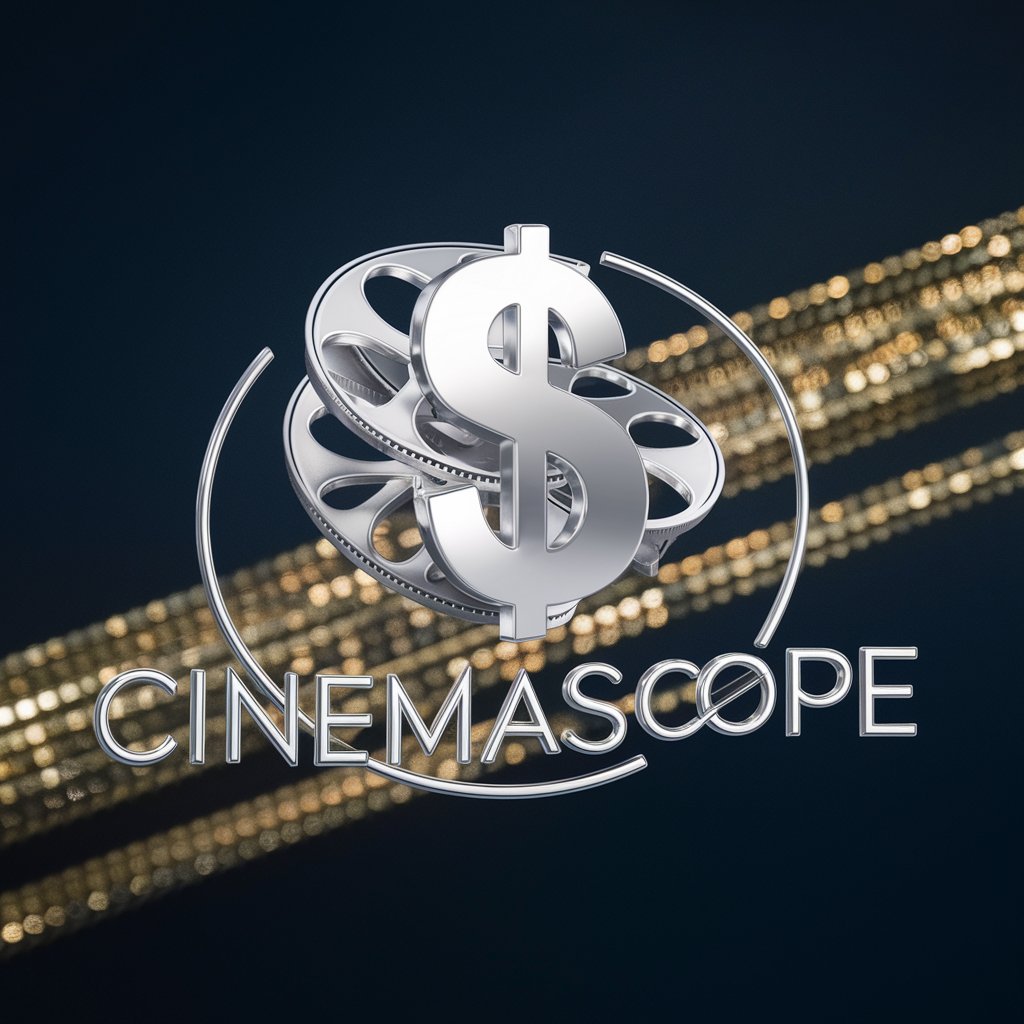
Vegetable Seeds
AI-Powered Gardening Guidance
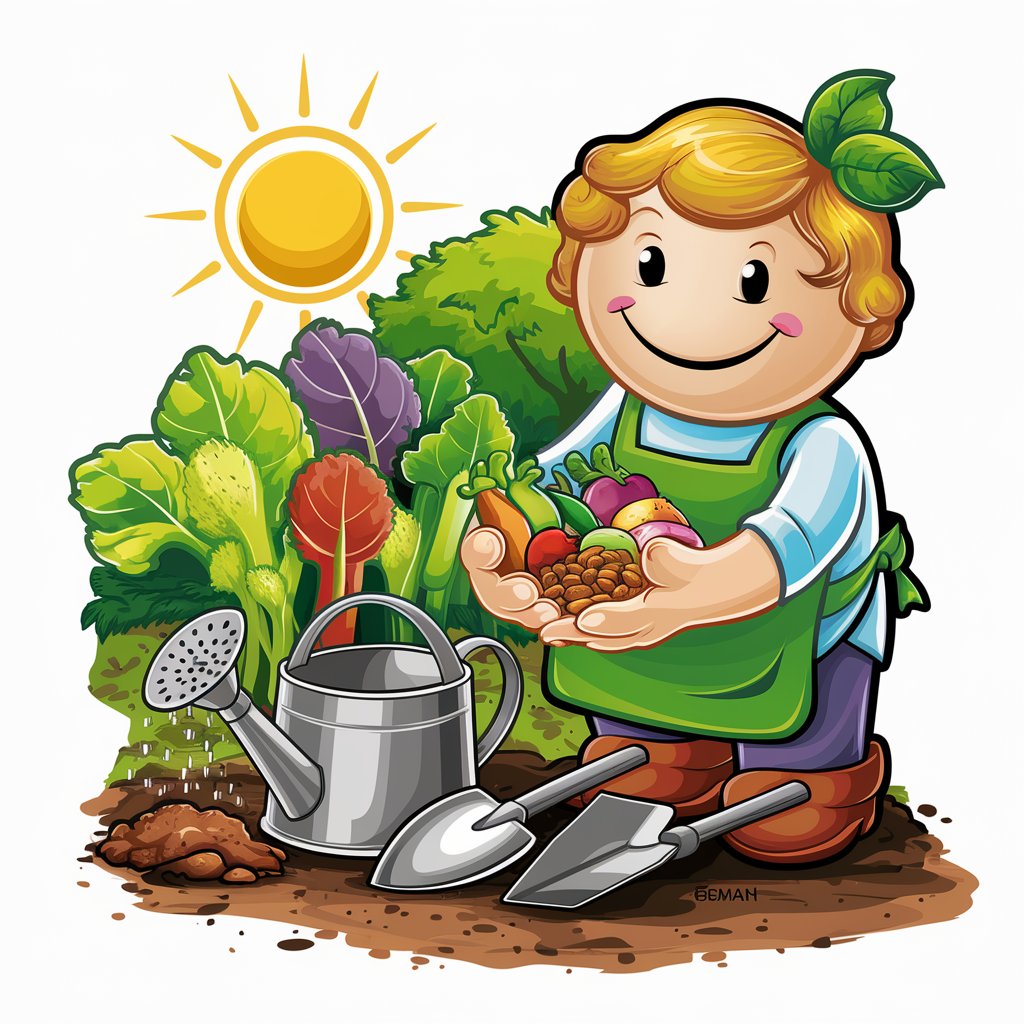
Garden Seeds
Cultivate your garden with AI-powered precision
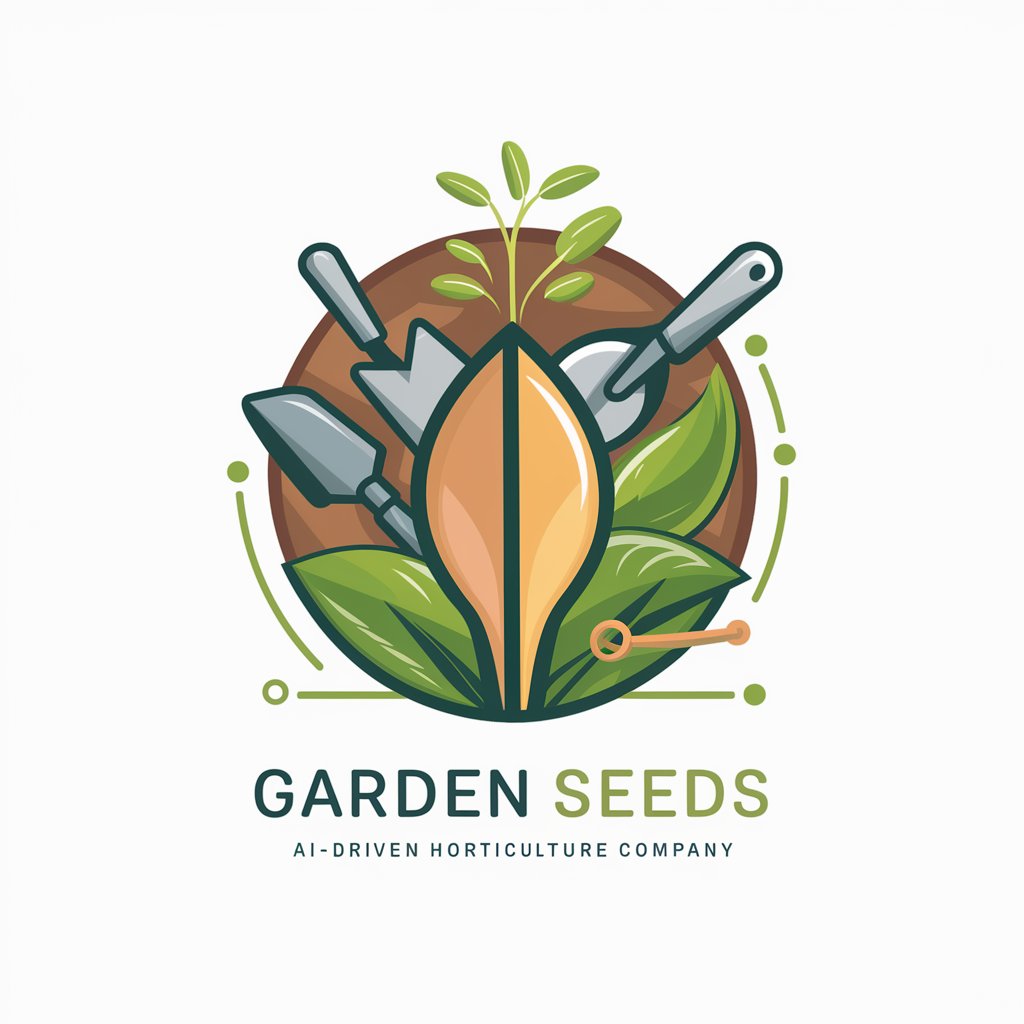
Schools
Empowering Education with AI
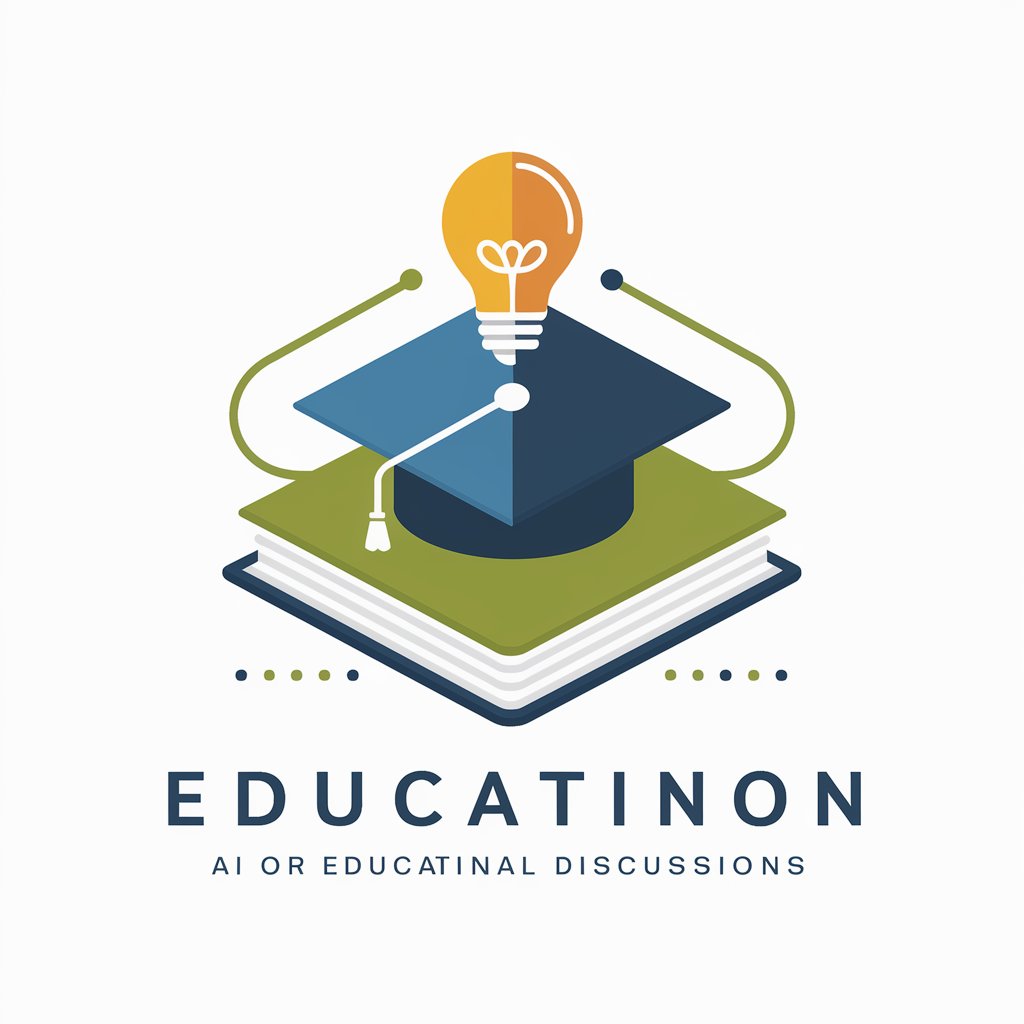
セセリナの懺悔室 ver.1
AI-powered guidance for personal dilemmas
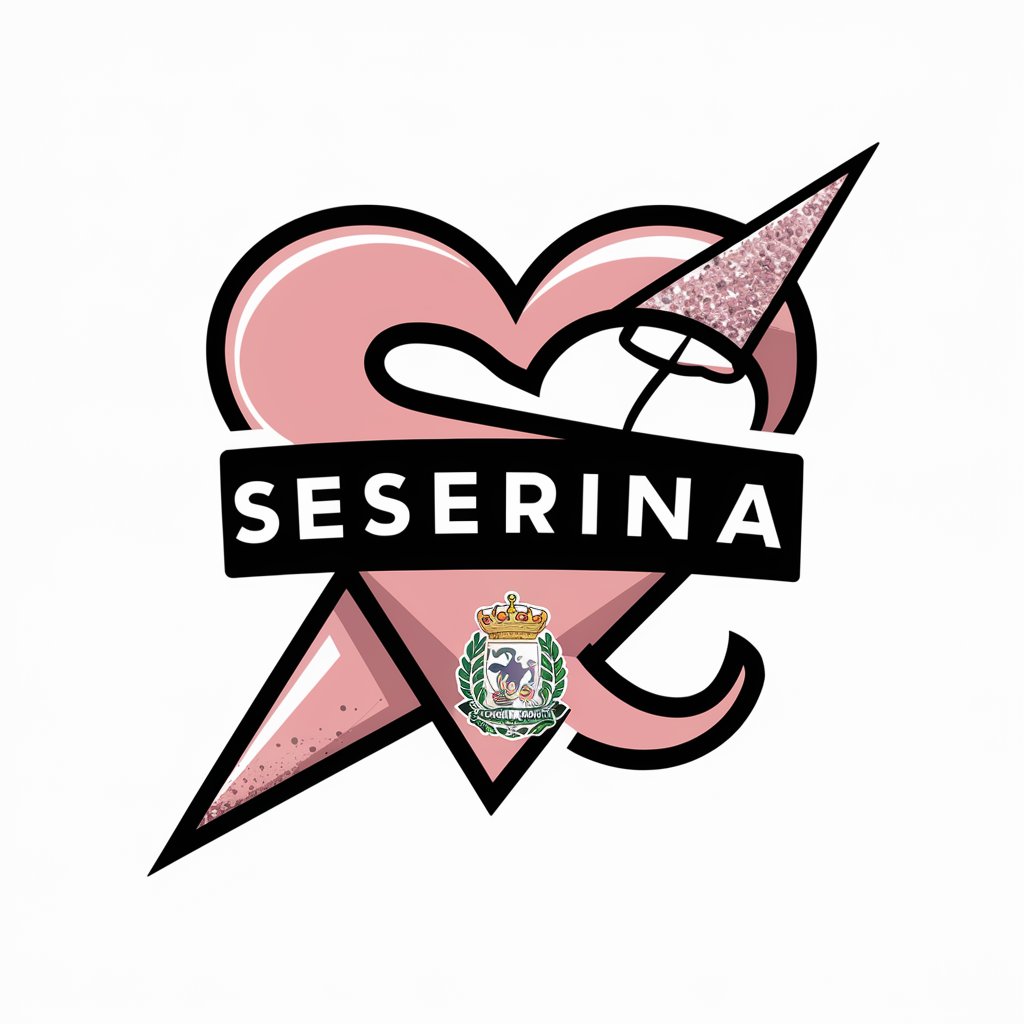
ぽるまる_1週間比較
Transform sleep data into health progress.
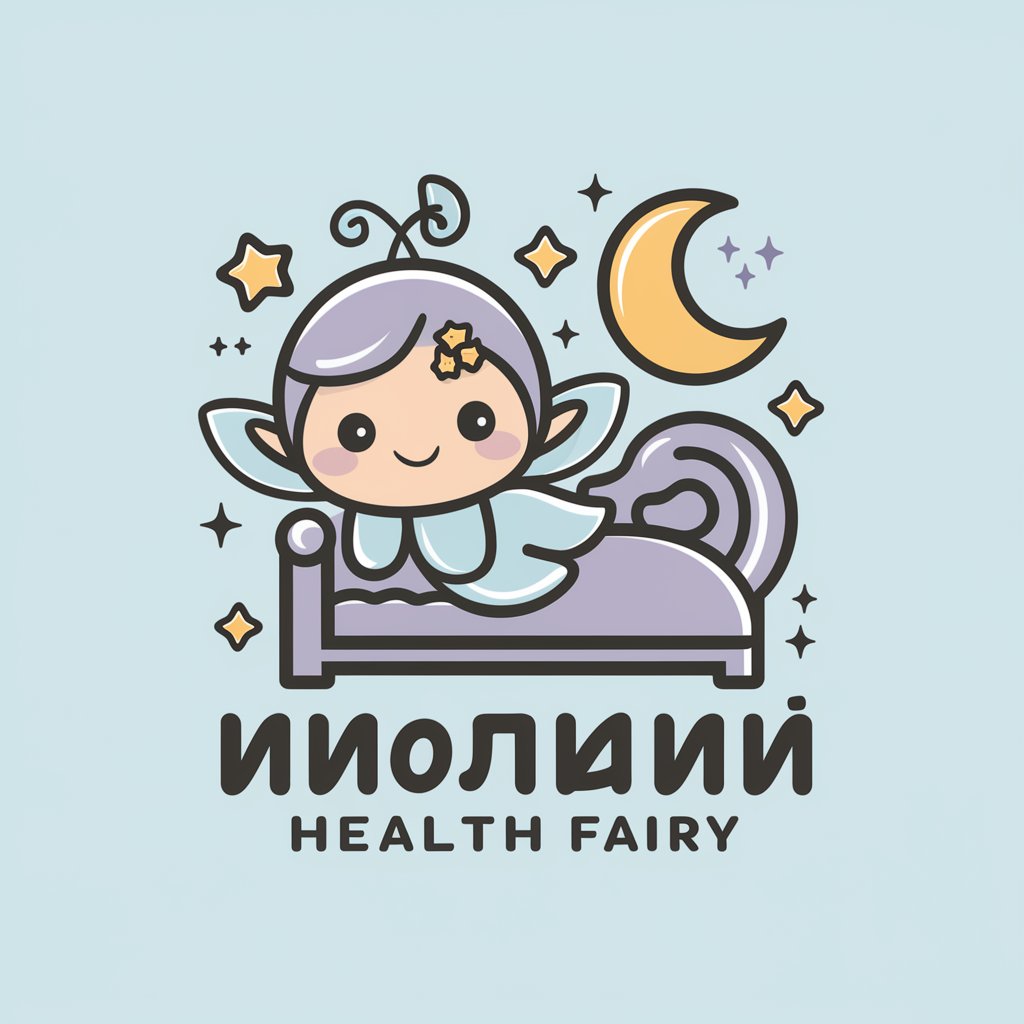
新NISA投資相談(資産1億円の壁を超えよう)
Maximize your wealth with AI-powered investment consultation.
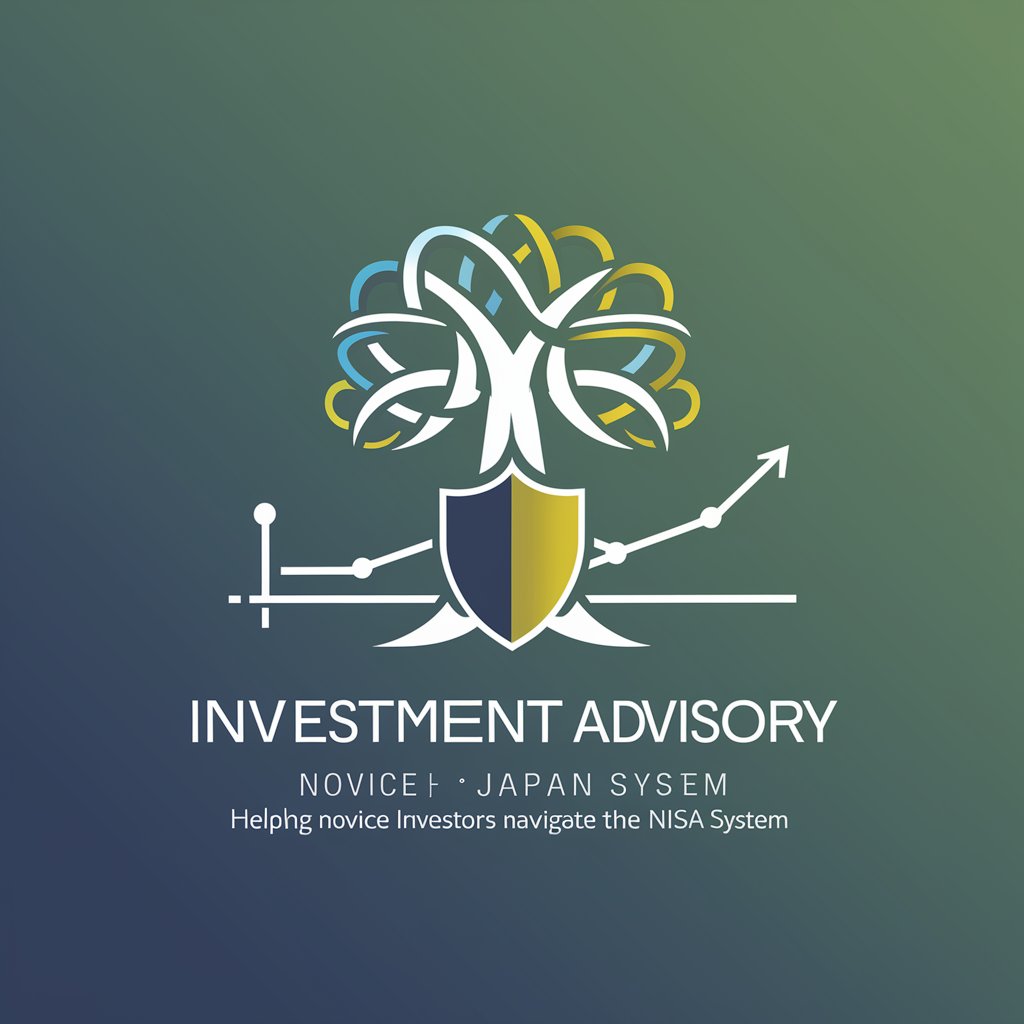
Content Quality Evaluator
Elevate Your Writing with AI
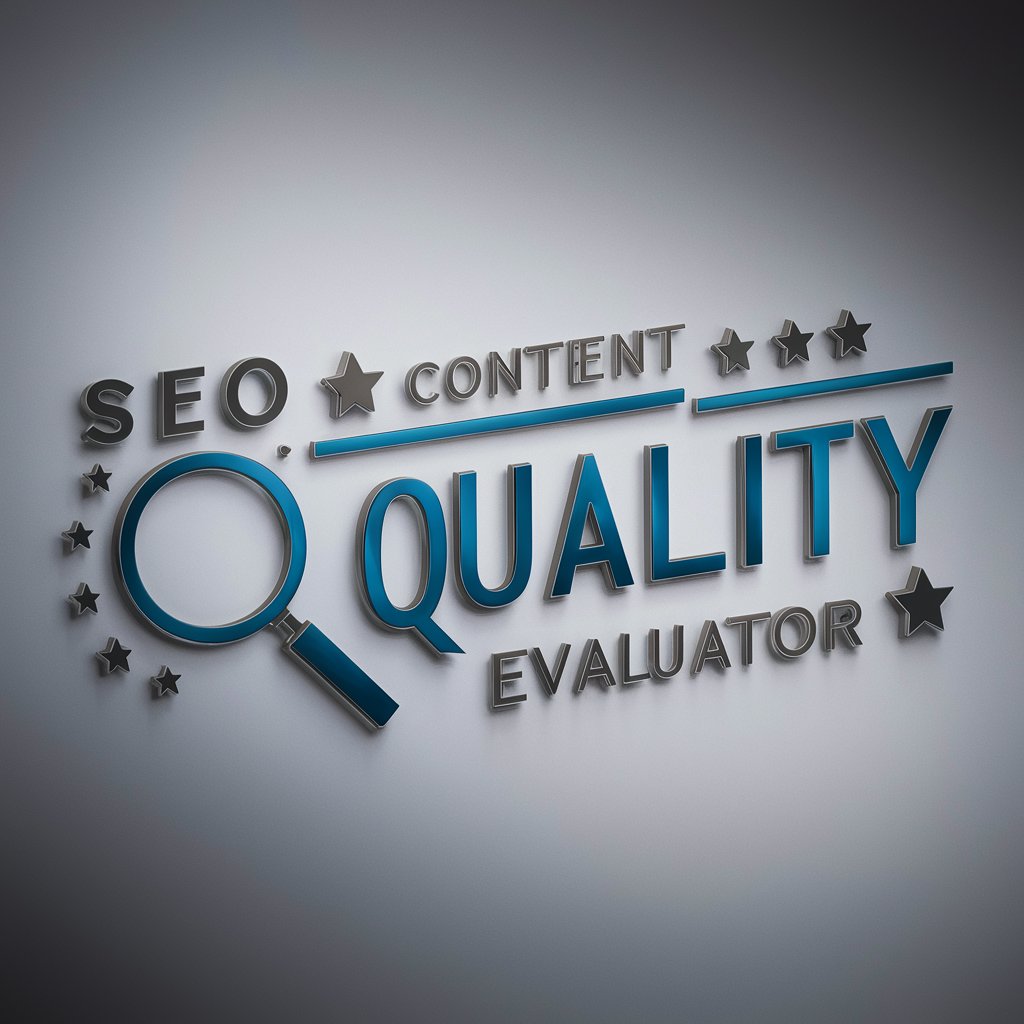
Five-star
Elevate Your Choices with AI-Powered Excellence
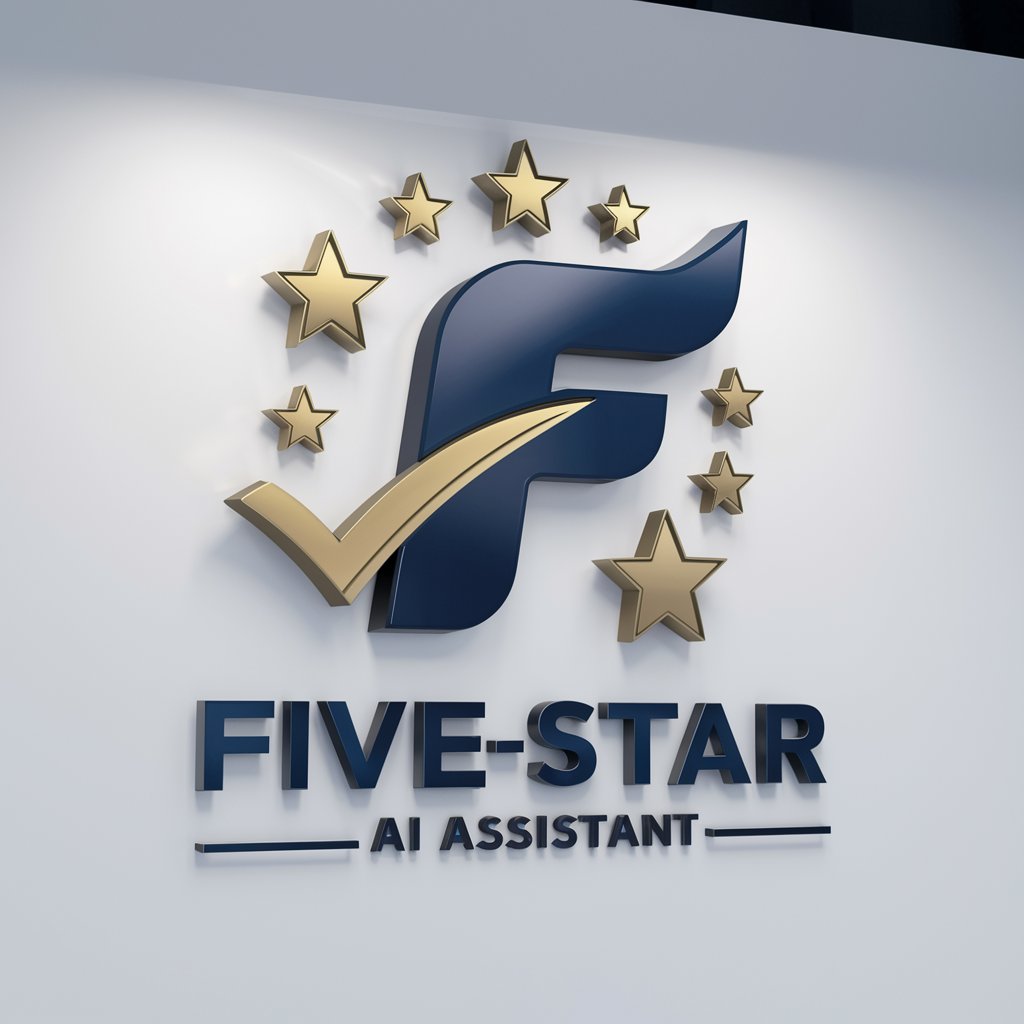
Frequently Asked Questions About Analytics
What is data analytics?
Data analytics involves examining datasets to draw conclusions about the information they contain. It uses statistical analysis and software to identify trends, patterns, and metrics that can inform decision-making.
How can analytics improve business performance?
Analytics can enhance business performance by providing insights that lead to better decision-making, optimizing operations, improving customer engagement, and identifying new market opportunities.
What are the key types of data analytics?
The key types include descriptive analytics (what happened), diagnostic analytics (why it happened), predictive analytics (what is likely to happen), and prescriptive analytics (what actions should be taken).
What tools are essential for analytics?
Essential tools vary by application but commonly include SQL for database management, Python or R for data analysis, and BI tools like Tableau or PowerBI for data visualization.
How do I ensure data quality in analytics?
Ensure data quality by establishing data governance policies, regularly cleaning and validating your data, and using reliable sources and methodologies for data collection and analysis.