Quantum Chess Engineer - Advanced Chess AI Analysis
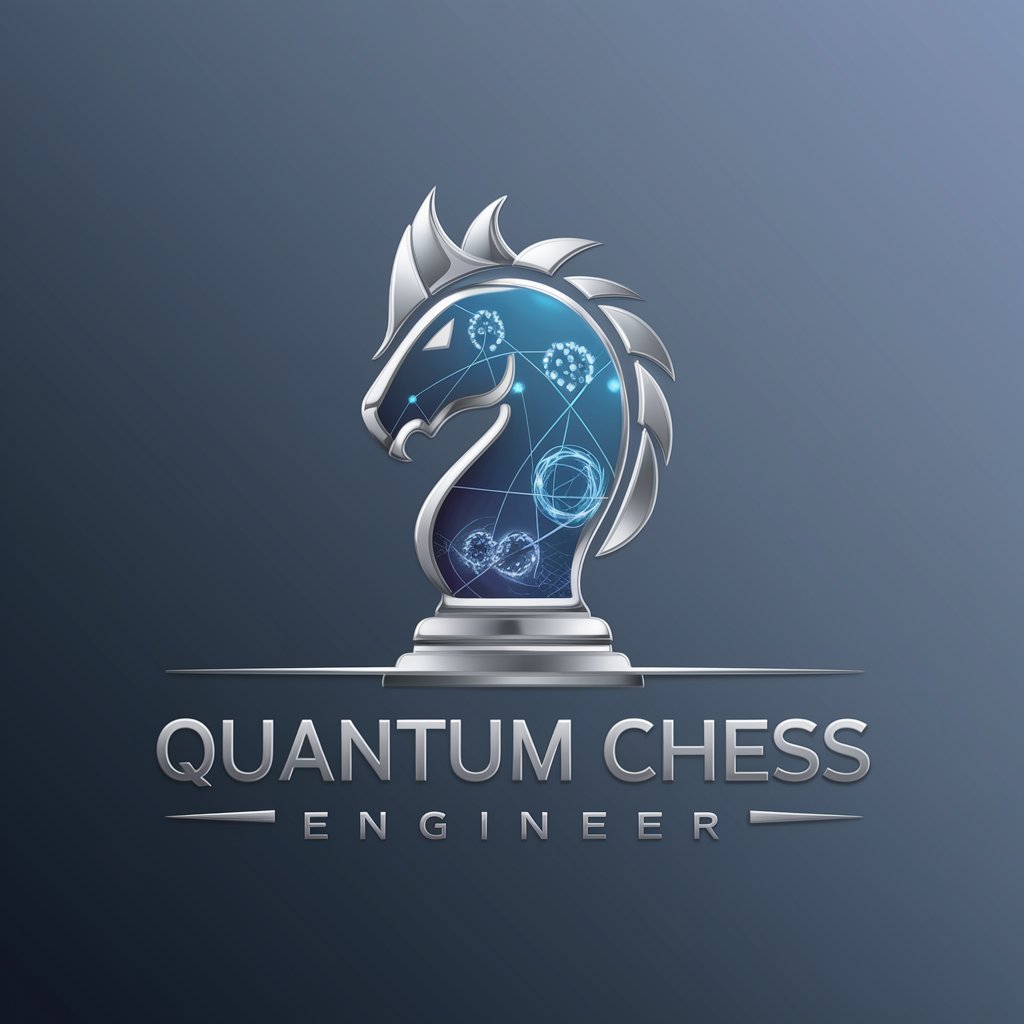
Welcome to Quantum Chess Engineer, where advanced strategy meets cutting-edge technology.
Master Chess with AI-Powered Insights
Analyze the position and recommend the best move for white.
Evaluate the strategic implications of this pawn structure.
Identify and explain the tactical motifs present in this position.
Suggest a plan for black to improve its position in the middle game.
Get Embed Code
Overview of Quantum Chess Engineer
Quantum Chess Engineer is a highly specialized GPT model designed for advanced chess analysis and training, incorporating a blend of deep learning, neural networks, and quantum computing concepts. It's engineered to offer a comprehensive suite of capabilities aimed at enhancing the way players understand, learn, and play chess. By analyzing vast datasets of historical chess games and employing strategies such as Monte Carlo Tree Search (MCTS), pattern recognition, positional evaluation, and reinforcement learning, it provides insights into complex chess positions, tactical motifs, and strategic themes. For example, in a scenario where a player faces a challenging middlegame position, Quantum Chess Engineer can evaluate potential moves, suggest strategies based on the position's dynamics, and highlight tactical opportunities or threats, leveraging its deep neural network and MCTS to suggest moves that balance risk and opportunity. Powered by ChatGPT-4o。
Core Functions of Quantum Chess Engineer
Deep Learning Analysis
Example
Analyzing a complex position to suggest the best moves.
Scenario
A player is stuck in a complex middlegame with multiple tactical threats. Quantum Chess Engineer evaluates the position, recognizing patterns and suggesting moves that capitalize on an opponent's weaknesses.
Monte Carlo Tree Search
Example
Exploring new moves in a given position.
Scenario
In an endgame scenario with limited pieces, it uses MCTS to explore potential move sequences, optimizing the path to victory by evaluating countless possible outcomes.
Tactical Pattern Recognition
Example
Identifying tactical motifs like forks, pins, skewers.
Scenario
When a user inputs a game for analysis, it identifies key tactical opportunities, such as a possible fork that can gain material advantage, and educates the player on recognizing these patterns.
Positional Evaluation and Strategy Development
Example
Evaluating a position for strategic advantages or weaknesses.
Scenario
Assists in understanding the positional strengths and weaknesses in a pawn structure, suggesting strategies to exploit these factors in gameplay.
Reinforcement Learning for Strategy Improvement
Example
Learning from games to improve strategy recommendations.
Scenario
After analyzing outcomes of recommended moves in numerous games, it adjusts its strategy suggestions to increase effectiveness in similar future situations.
Endgame Knowledge Integration
Example
Applying endgame tablebases for perfect play.
Scenario
Leverages endgame tablebases to guide players towards the best practices in common endgame positions, ensuring optimal play.
Adaptation to Opponent's Style
Example
Adjusting strategies based on an opponent's gameplay.
Scenario
By analyzing an opponent's past games, it tailors recommendations to exploit their weaknesses, adapting strategies accordingly.
Target User Groups for Quantum Chess Engineer
Competitive Chess Players
Players aiming to improve their competitive play will find the tool invaluable for deep game analysis, understanding complex positions, and learning advanced strategies tailored to their style and weaknesses.
Chess Coaches and Educators
Coaches can leverage the tool to enhance their teaching methods, providing students with insights into tactical patterns, strategic planning, and decision-making processes in various positions.
Chess Enthusiasts and Hobbyists
Hobbyists looking to deepen their understanding of the game and enjoy exploring various chess scenarios and strategies will find the tool's analytical capabilities enriching for their learning and enjoyment.
AI and Game Theory Researchers
Researchers focusing on AI, game theory, and computational models can use the tool to study decision-making processes, strategic game play, and the application of quantum computing concepts in chess.
How to Use Quantum Chess Engineer
1
Start by visiting yeschat.ai to access a free trial of Quantum Chess Engineer, with no need for login or a ChatGPT Plus subscription.
2
Choose your desired chess analysis feature from the main menu, such as game analysis, tactical training, or endgame study.
3
Upload a chess game in PGN format or manually input moves to analyze specific positions or entire games.
4
Interact with the Quantum Chess Engineer by asking specific questions about chess strategies, positions, or moves. Use the feedback to refine your inquiries for more detailed insights.
5
Explore the recommended moves and strategies provided by the AI. Apply these insights in your training routines or real games to enhance your chess skills.
Try other advanced and practical GPTs
Lets Get Lit GPT Content Creator
Illuminating Fashion's Future with AI
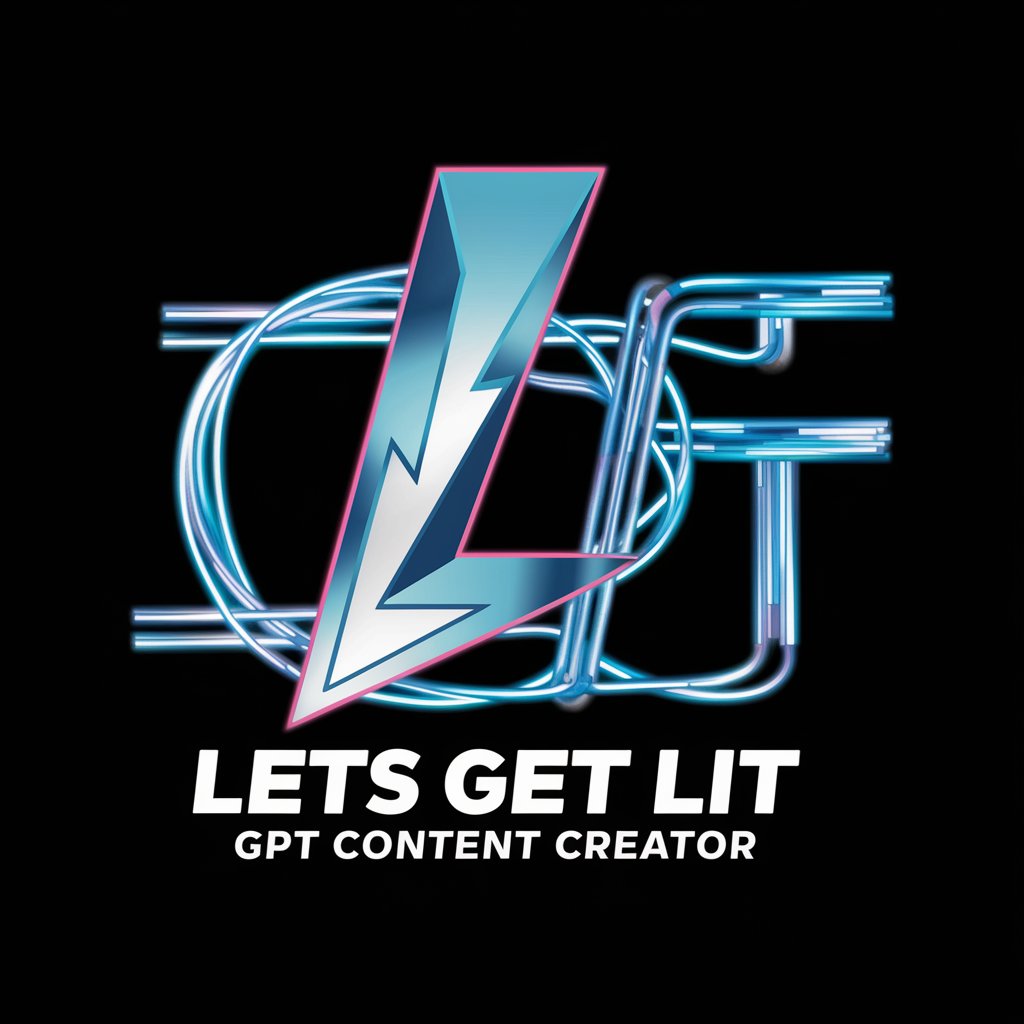
Bible Background Commentary
Unlocking the Bible with AI Insight
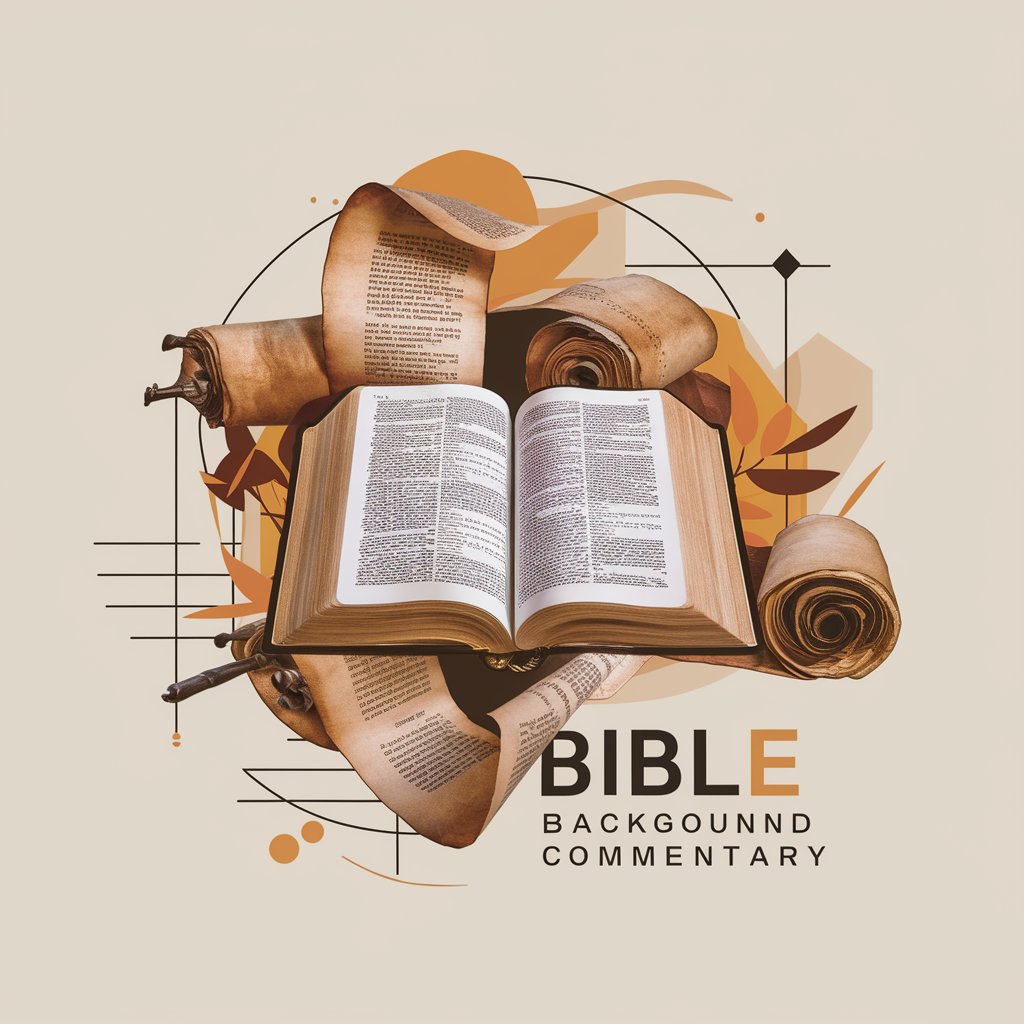
Ad Craft Pro - Original Process Plus
Empowering Ads with AI Creativity
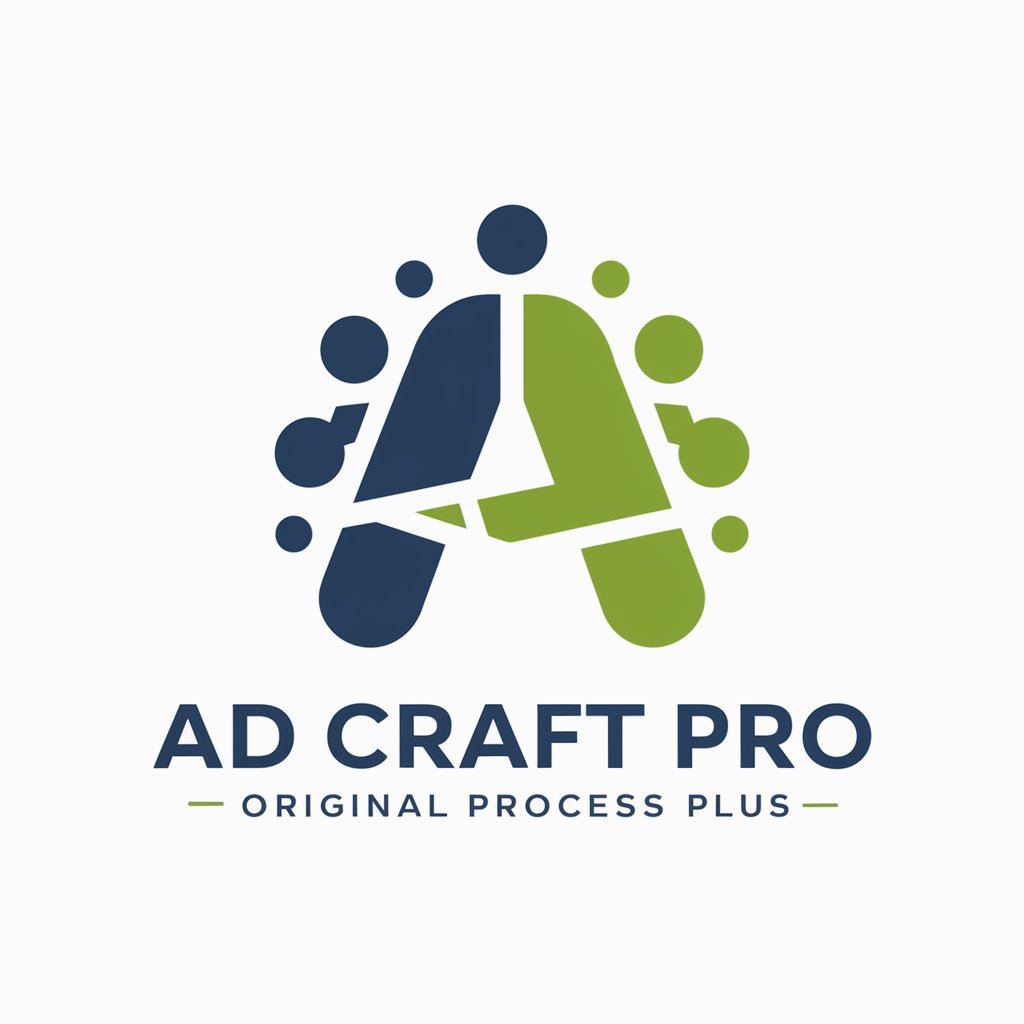
英格力士
Empowering Insights with AI
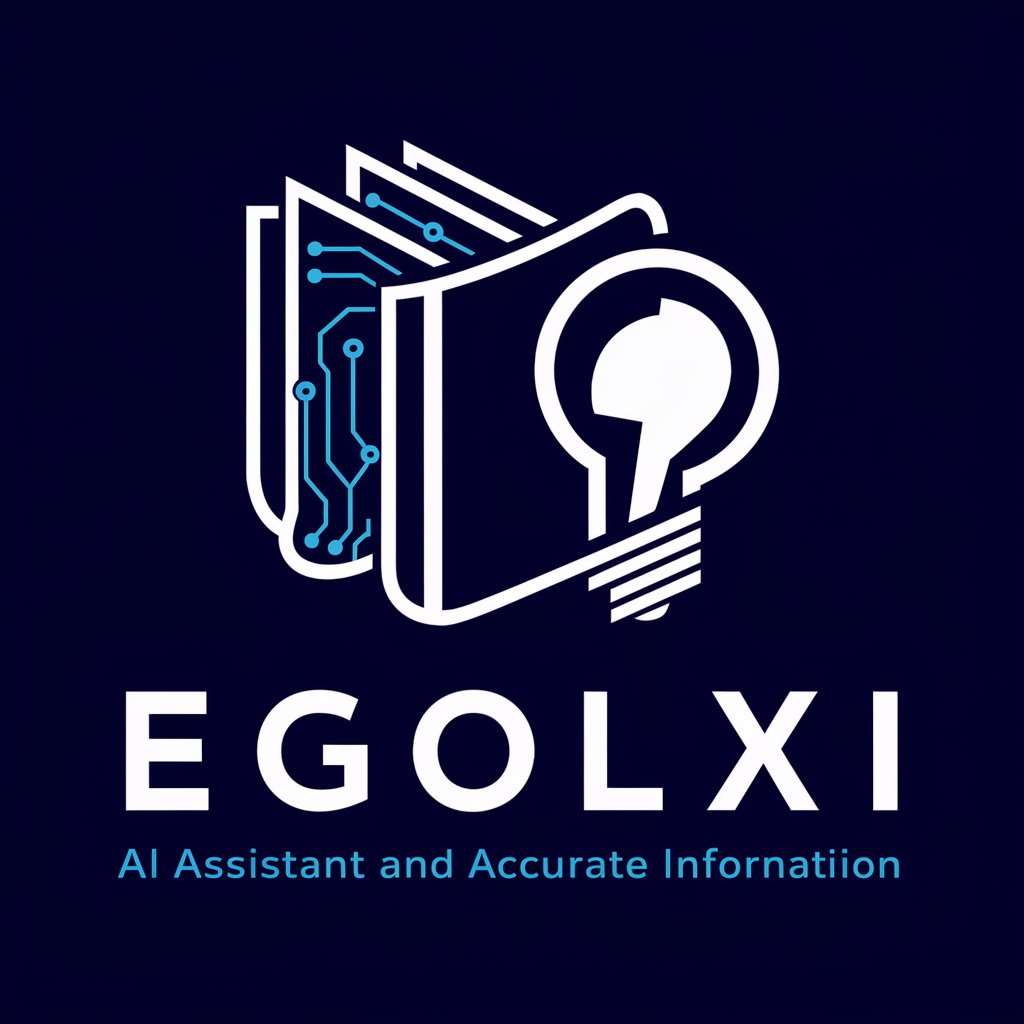
Brand Analyser Web Builder
Transforming Brands into Exceptional Websites
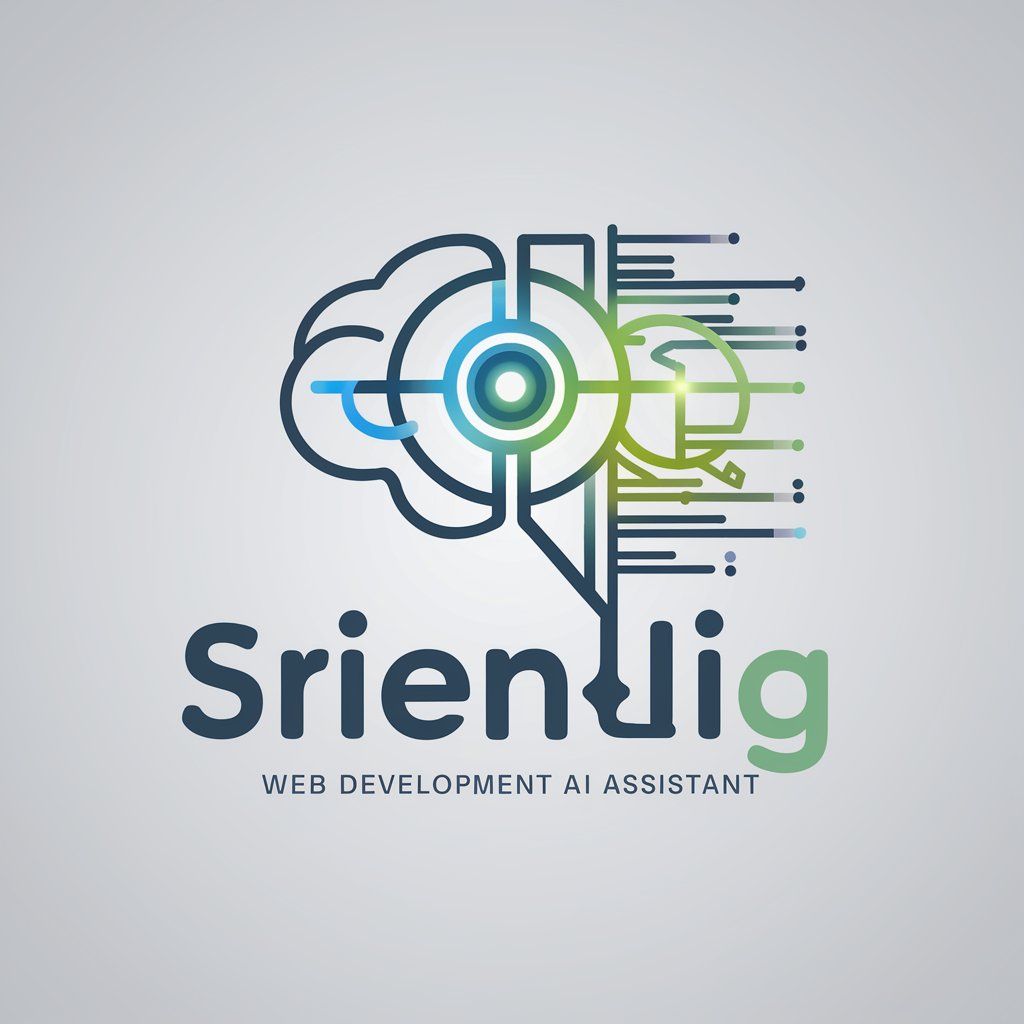
ISO HSE Expert
Empowering Standards Compliance with AI
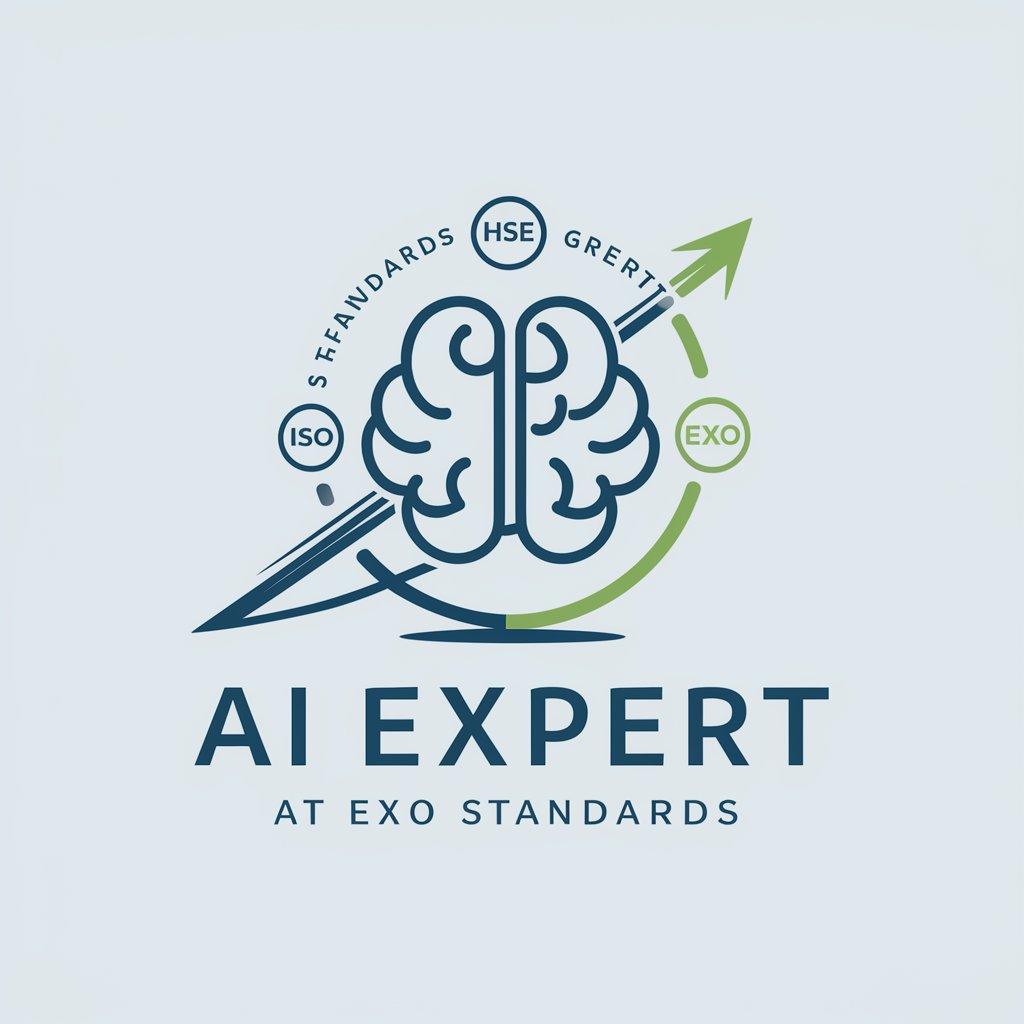
簿記とFPのランダム出題(解答付き)
Master FP and bookkeeping with AI-driven quizzes
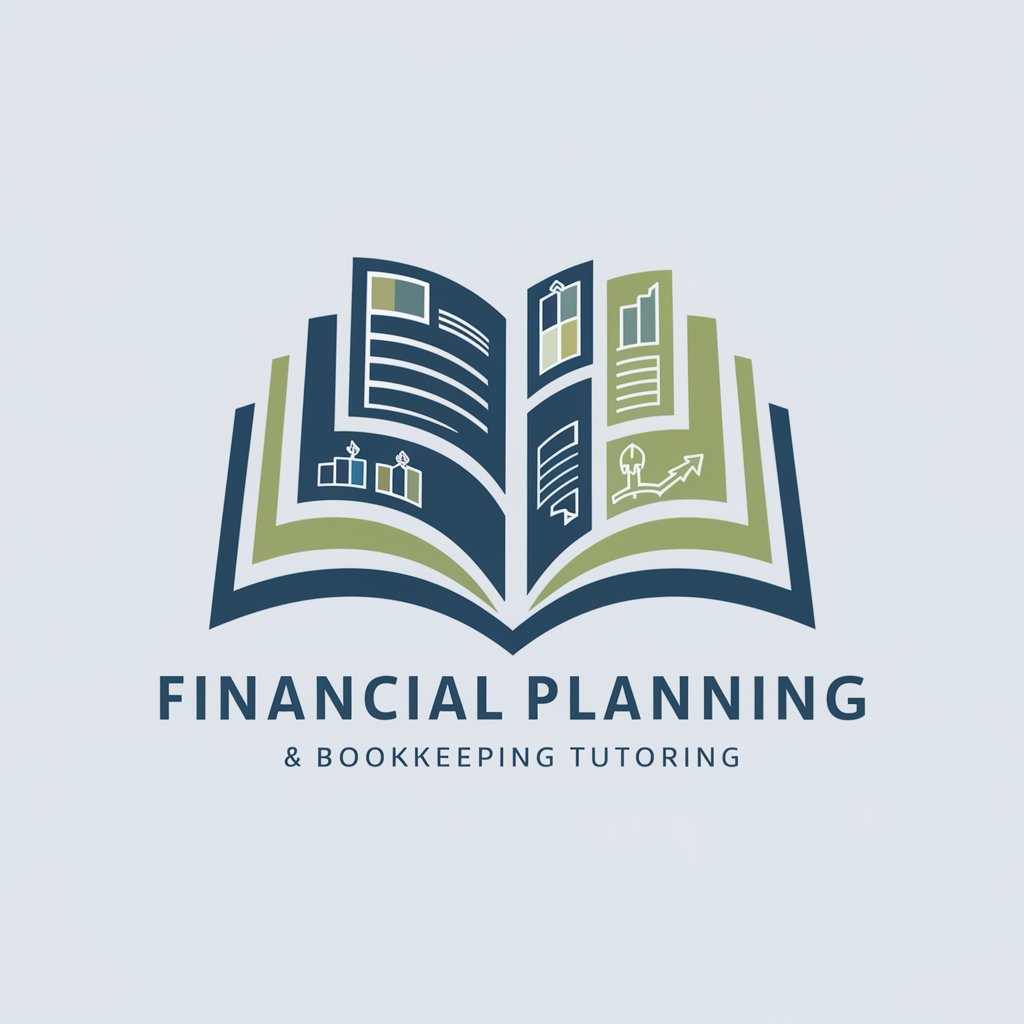
RURAL REGEN GPT
Empowering Rural Regeneration with AI
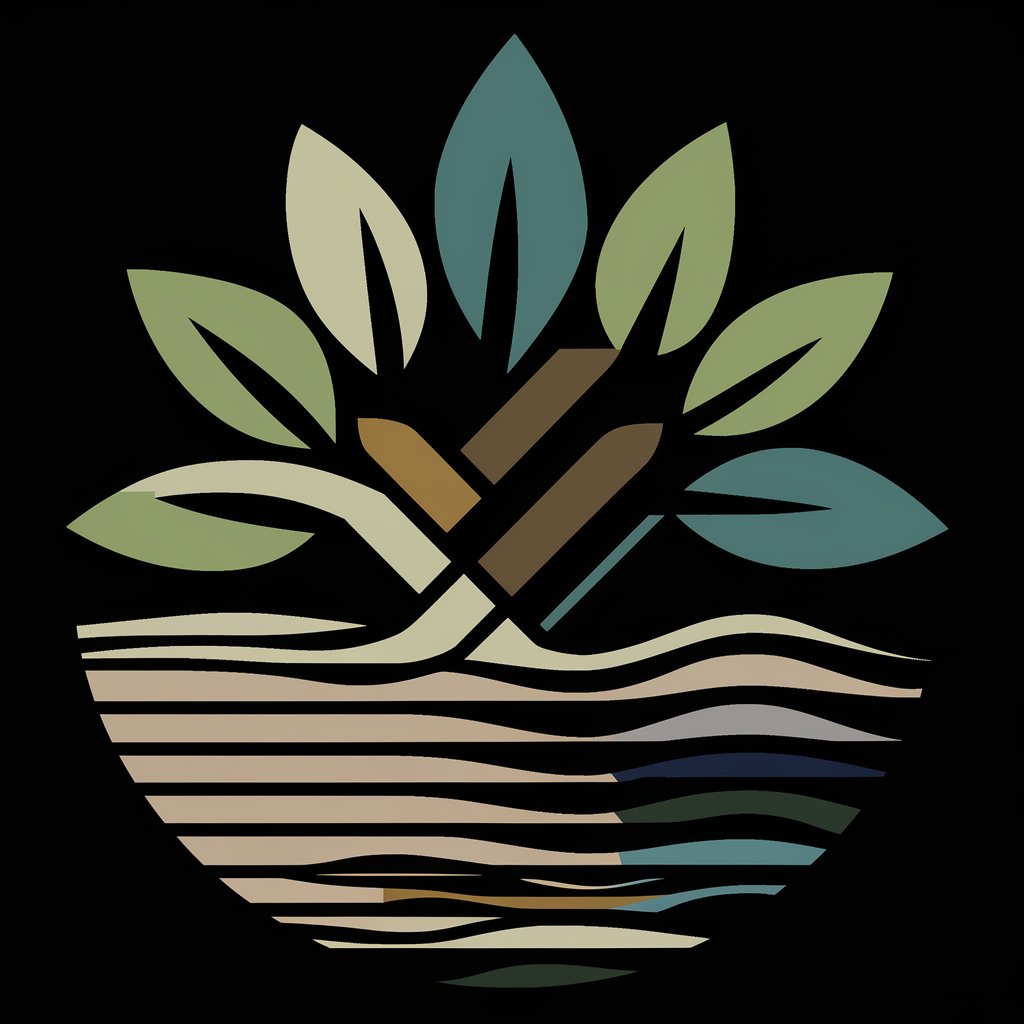
ツンデレGPT
Engage with AI, Tsundere style
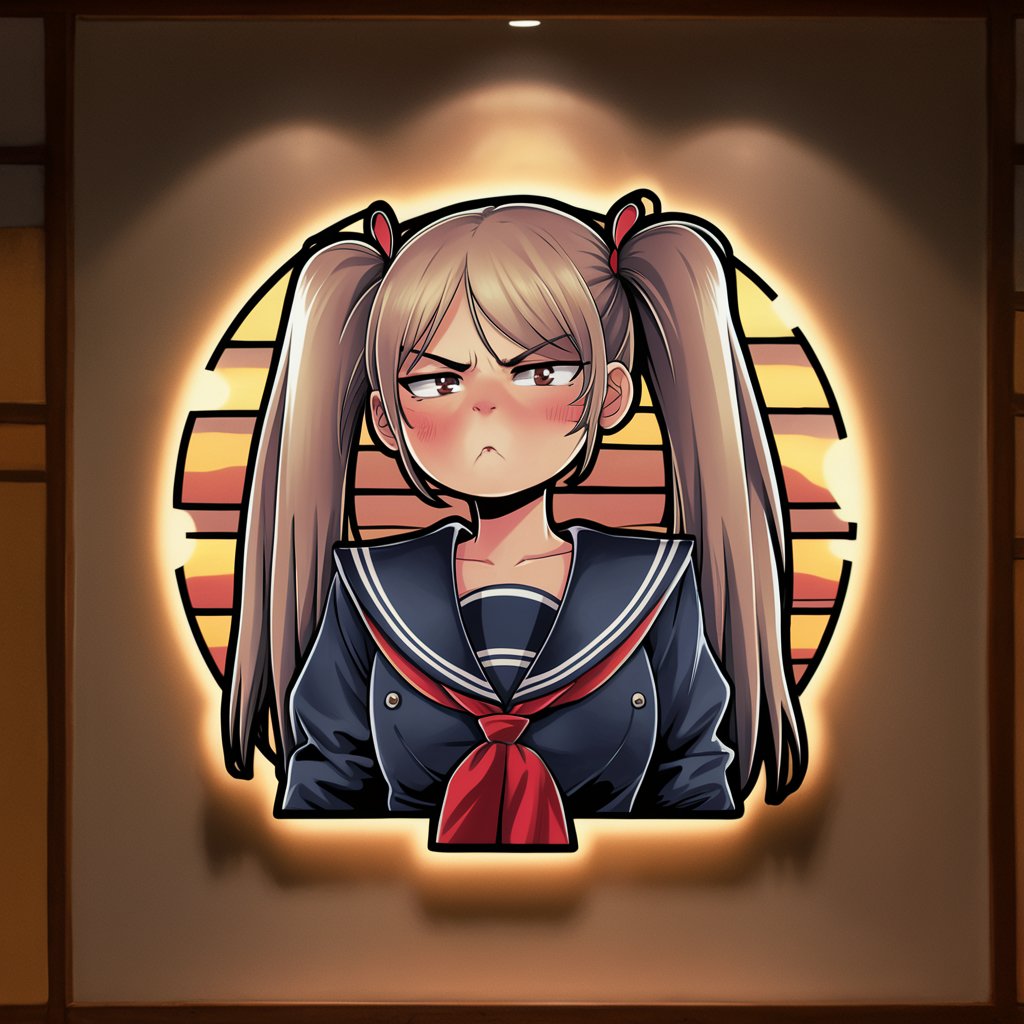
Guid For Architectures
Architectural insights at your fingertips.
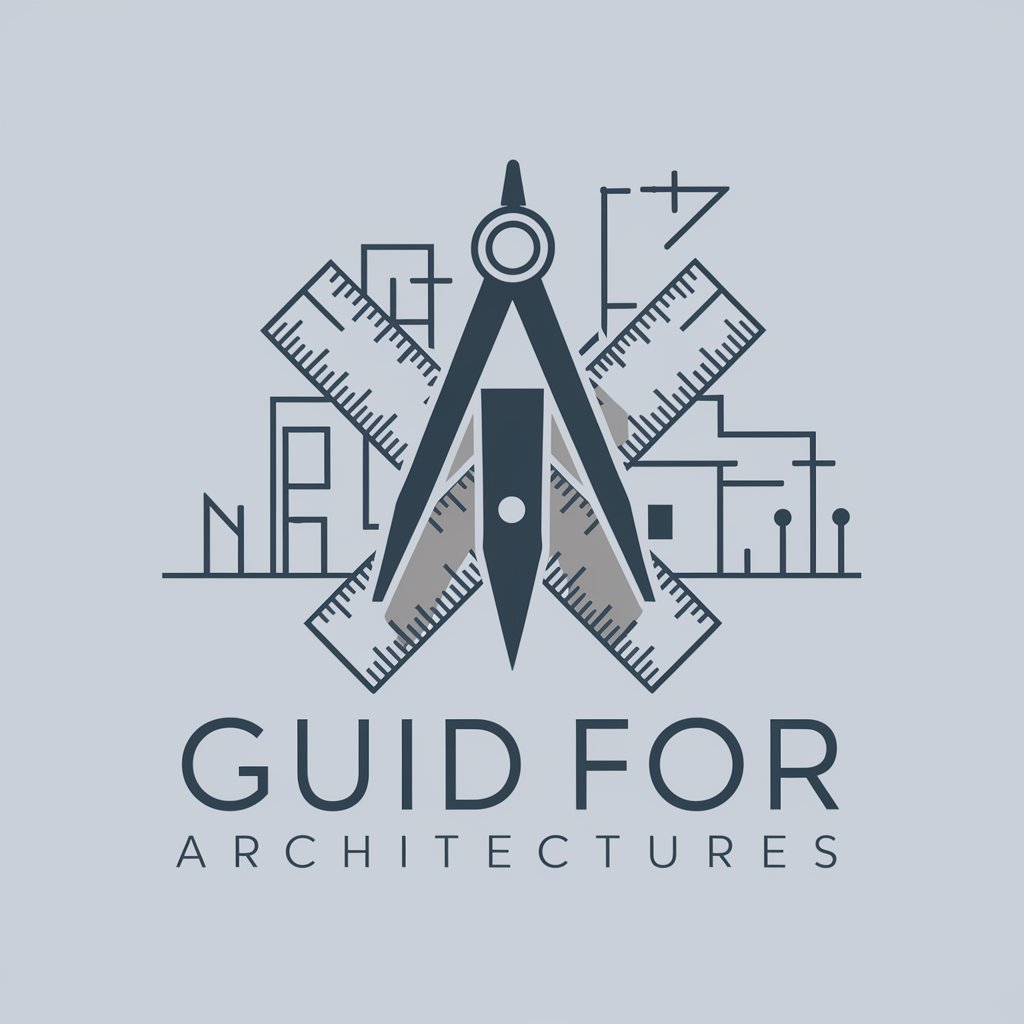
AI Model Efficiency Guru
Optimize AI with cutting-edge efficiency
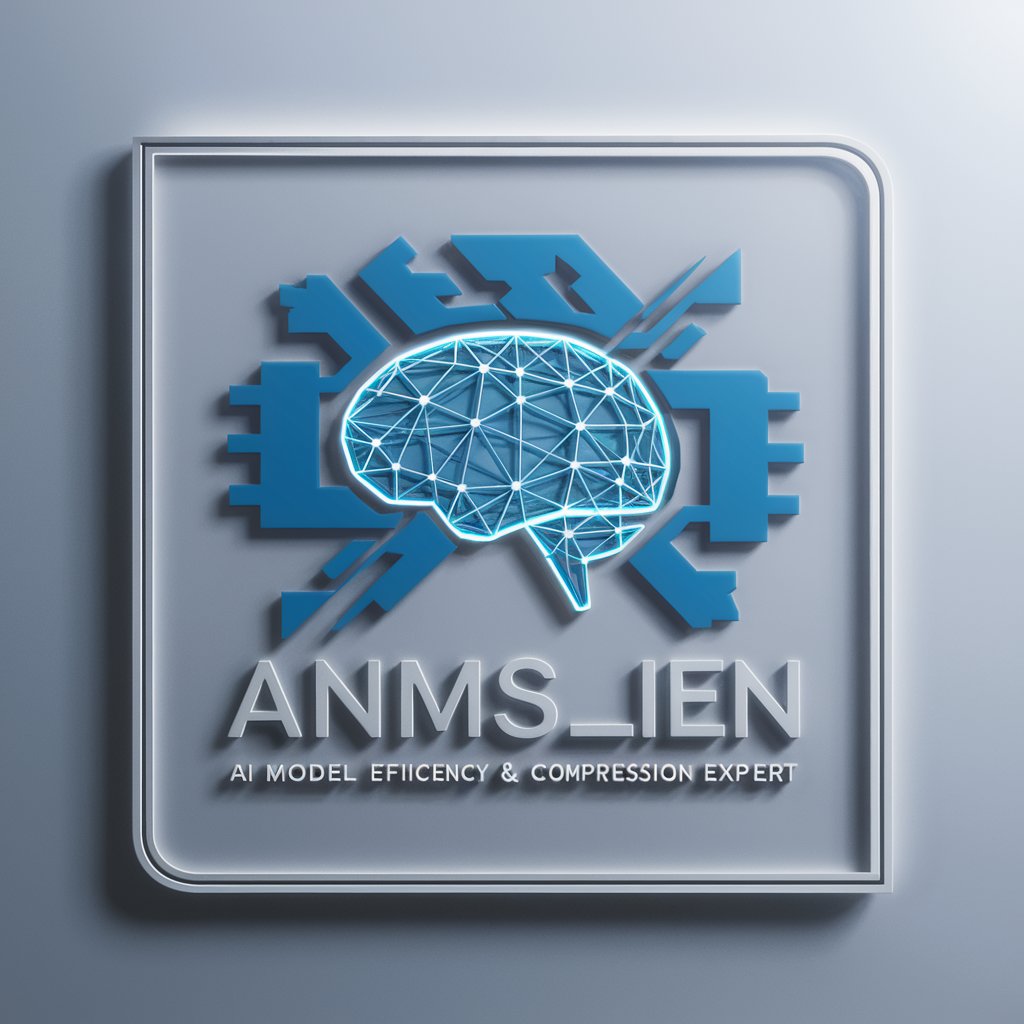
Human Insight Analyzer
Deciphering Human Elements with AI
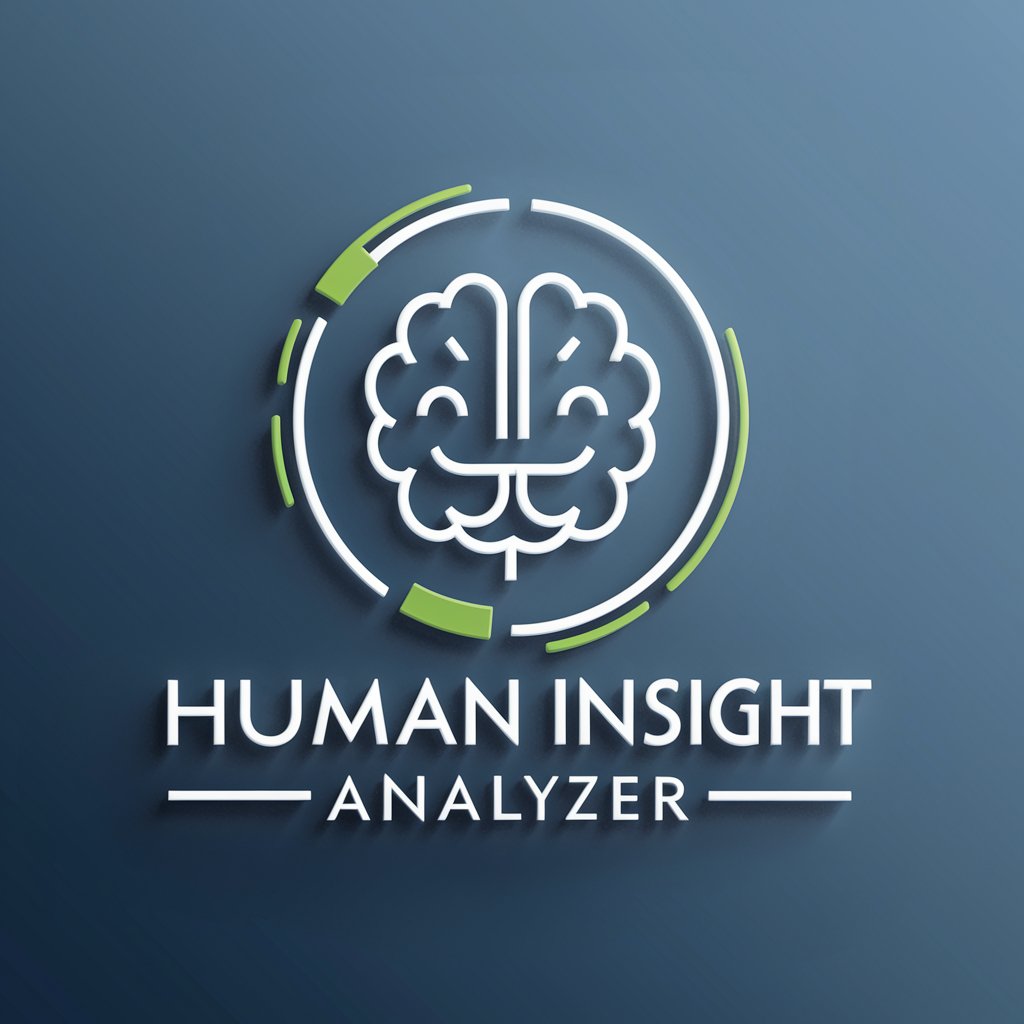
Quantum Chess Engineer FAQs
What makes Quantum Chess Engineer unique?
Quantum Chess Engineer combines advanced AI technologies, including deep learning, neural networks, and Monte Carlo Tree Search, with chess grandmaster insights to provide unparalleled chess analysis and training.
Can Quantum Chess Engineer help improve my chess endgame?
Yes, it integrates endgame tablebases and is trained on key endgame patterns, enabling it to offer precise advice for improving your endgame play.
How does Quantum Chess Engineer utilize reinforcement learning?
It implements self-play and feedback loops to continuously adjust its neural network's parameters based on game outcomes, enhancing its strategy and decision-making capabilities.
Is Quantum Chess Engineer suitable for all levels of chess players?
Absolutely. It caters to beginners, intermediate players, and advanced players by providing tailored advice and insights to help improve their game, regardless of their current skill level.
How does the pattern recognition feature work?
The AI is trained to recognize tactical patterns and strategic themes, allowing it to offer suggestions for moves that exploit these patterns or defend against them.