Semantic Scene Explorer - Textual and Image Analysis

Hello! Need help with Semantic and Instance Segmentation? Ask away!
Uncover Insights with AI-Powered Analysis
What is instance segmentation?
How can I categorize these objects?
Explain hierarchical clustering in segmentation.
Describe the scene in this text.
Get Embed Code
Introduction to Semantic Scene Explorer
Semantic Scene Explorer is designed to assist with Semantic and Instance Segmentation in textual data. Its primary goal is to analyze and categorize text-based information regarding objects and scenes, enabling a more organized and accessible way to handle data. Through hierarchical clustering, Semantic Scene Explorer can group similar objects or scenes together, facilitating efficient data analysis and retrieval. For instance, in a dataset containing detailed descriptions of urban environments, Semantic Scene Explorer can identify and categorize different elements like buildings, vehicles, and pedestrians, highlighting their relationships and characteristics. This capability is invaluable for tasks requiring detailed scene understanding and object identification, ranging from content creation to academic research. Powered by ChatGPT-4o。
Main Functions of Semantic Scene Explorer
Semantic Segmentation
Example
Analyzing a textual description of a street scene to identify and label each element, such as 'sidewalk', 'tree', 'car', and 'pedestrian'.
Scenario
In urban planning research, extracting specific features from textual descriptions of cityscapes to study the distribution of green spaces and vehicles.
Instance Segmentation
Example
Distinguishing between different instances of the same category, like different 'cars' in a parking lot description, labeling them as 'Car 1', 'Car 2', etc.
Scenario
For automotive industry analysis, identifying and comparing mentions of different car models in customer reviews.
Hierarchical Clustering
Example
Grouping similar objects or scene descriptions based on their characteristics, such as grouping all 'electric vehicles' or 'parks' together from a dataset.
Scenario
In environmental studies, clustering descriptions of natural habitats to identify common characteristics and differences, aiding in biodiversity research.
Ideal Users of Semantic Scene Explorer Services
Academic Researchers
Researchers in fields like urban planning, environmental science, and linguistics can utilize Semantic Scene Explorer to analyze textual data, extract and categorize relevant information, facilitating their studies on various topics.
Content Creators
Writers and journalists can use Semantic Scene Explorer to organize and analyze extensive descriptions or narratives, identifying key elements and themes for more focused content creation.
Data Analysts
Professionals in data analysis and information retrieval can leverage Semantic Scene Explorer to process large volumes of text data, enhancing data organization and insight generation for business intelligence.
How to Use Semantic Scene Explorer
Start for Free
Visit yeschat.ai to access Semantic Scene Explorer for a free trial, no login or ChatGPT Plus subscription required.
Upload Your Data
Upload text documents or images to analyze. Ensure your data is in a supported format for optimal processing.
Choose Analysis Type
Select between Semantic or Instance Segmentation, depending on your needs for object labels or scene descriptions.
Review and Refine
Examine the initial segmentation results. Use the tool's features to refine and adjust the analysis parameters as needed.
Export and Apply
Export the analyzed data for your use. Apply the insights to your project, whether for academic, professional, or personal purposes.
Try other advanced and practical GPTs
HISS Projekt Bot
Empowering IT support with AI

bpy
Powering Blender with AI-driven Python scripting
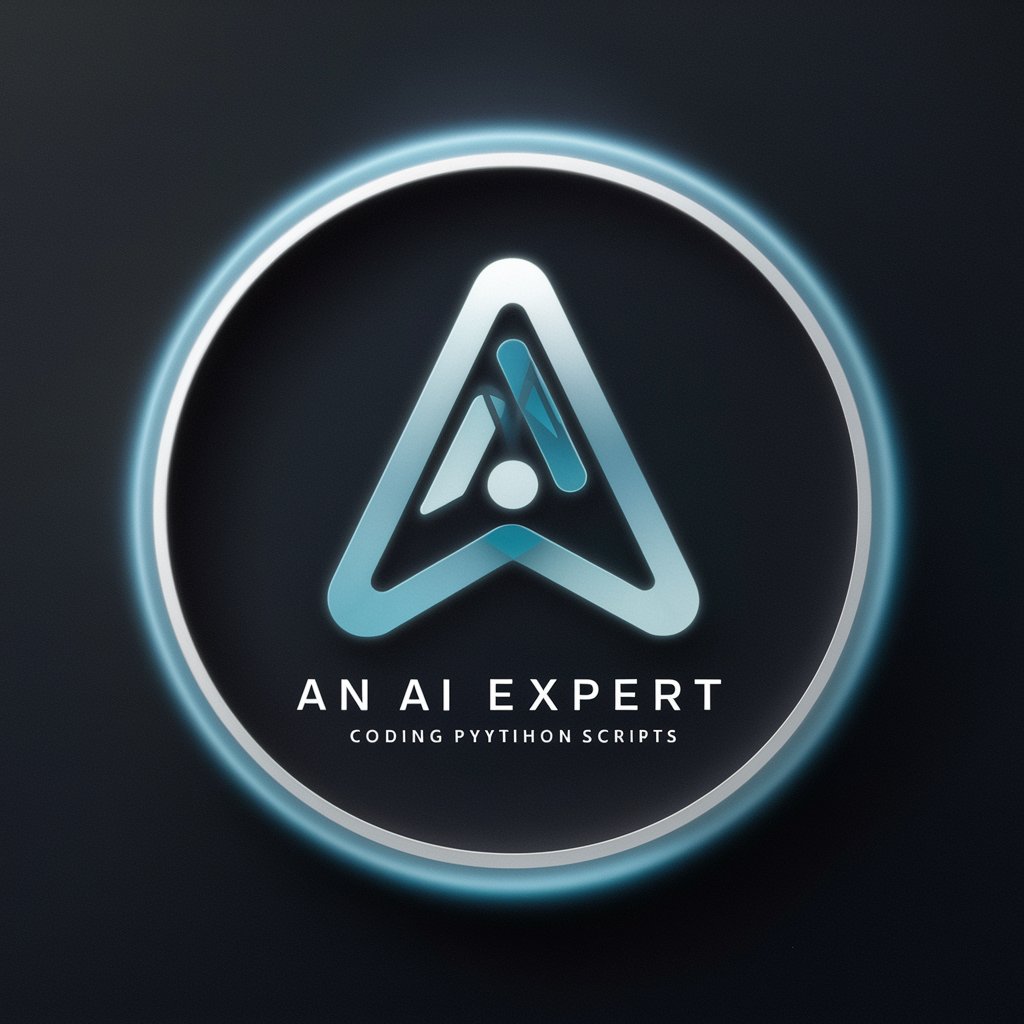
Canyon Mysteries: Kincaid's Legacy
Unravel history with AI-powered adventures

Android Ассистент
Empowering Android Users with AI

Price Scout
Smart Shopping with AI-Driven Insights
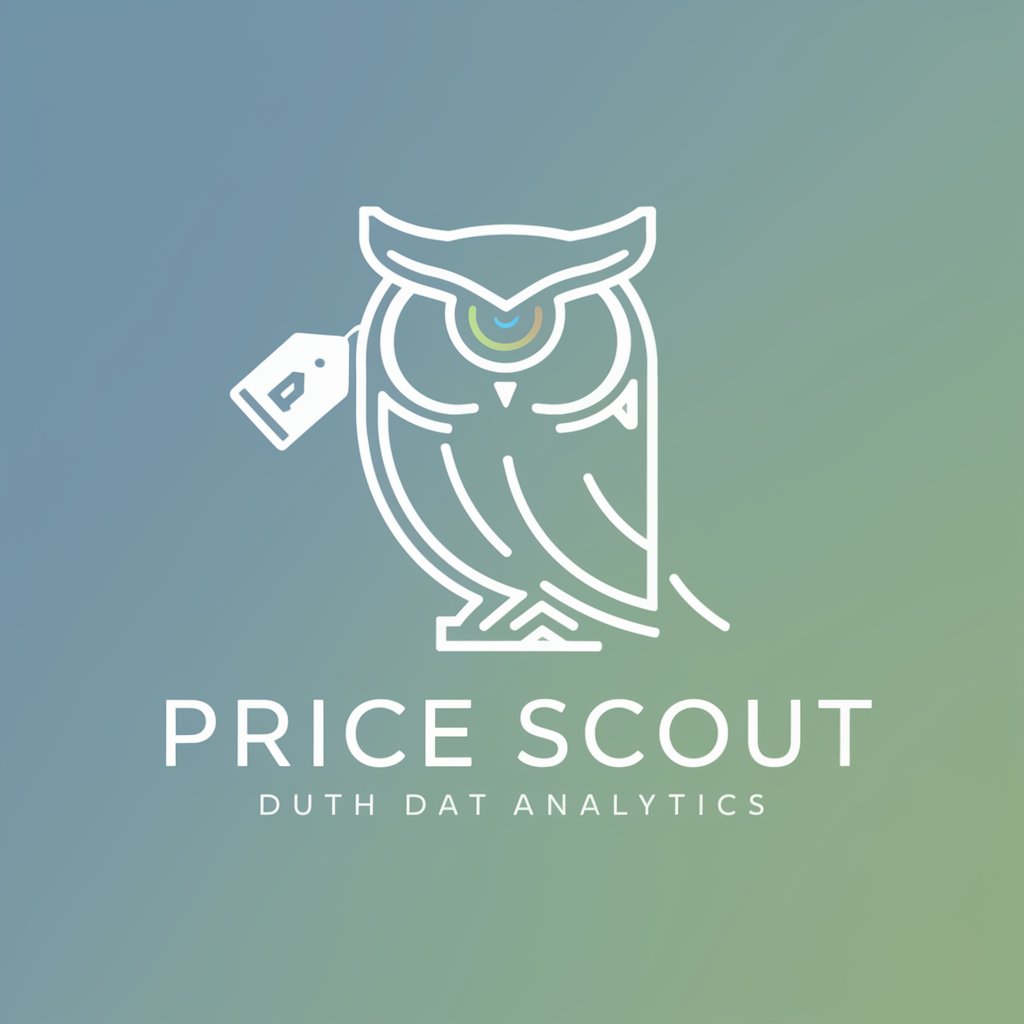
Eco Transport Sage
Driving Green Mobility Forward with AI

The REAL Santa Claus
Spreading cheer through AI-powered wisdom

InsightGraphicsPro
Turning Complex Data into Insightful Graphics
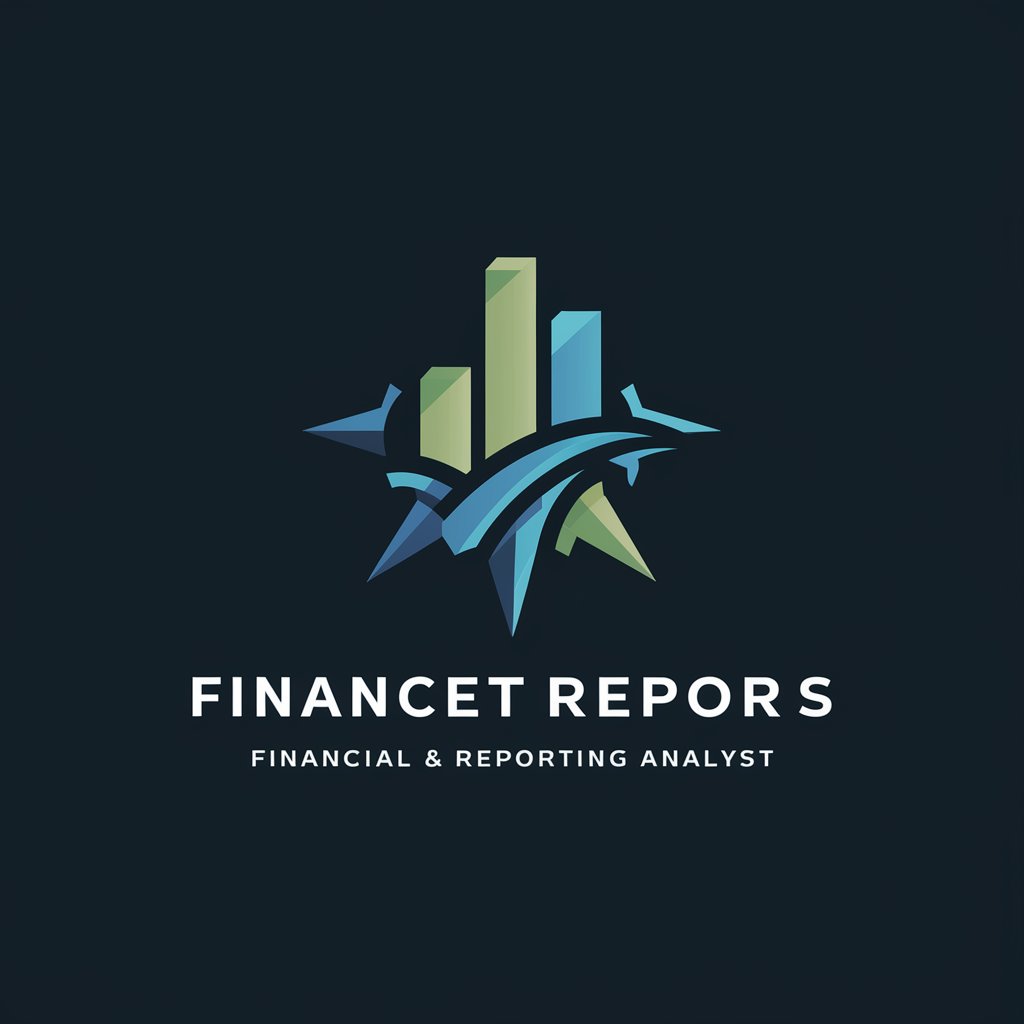
ハリウッドの悪役デザイナー
Craft Unique Villains with AI-Powered Creativity

Assistente de Aprendizagem para Autistas
Empowering Autistic Learners with AI

Vestle Jõuluvanaga
Chat with Santa, keep magic alive

Tuteur d'Humour
Elevate Your Humor with AI

Semantic Scene Explorer Q&A
What is Semantic Scene Explorer?
Semantic Scene Explorer is an AI-powered tool designed to perform detailed semantic and instance segmentation on textual data, helping users categorize and understand complex scenes and objects within their data.
Can Semantic Scene Explorer analyze images?
Yes, besides textual data, Semantic Scene Explorer can analyze images, providing detailed object labels and scene descriptions through advanced AI processing.
Who can benefit from using Semantic Scene Explorer?
Researchers, writers, data analysts, and developers, among others, can benefit from using Semantic Scene Explorer to organize, analyze, and derive insights from large volumes of text or image data.
How does Semantic Scene Explorer handle different data formats?
Semantic Scene Explorer is equipped to handle various data formats, including plain text, PDFs, and common image formats, allowing for versatile analysis capabilities across different types of content.
What makes Semantic Scene Explorer unique?
Its ability to provide detailed semantic and instance segmentation with high accuracy, supported by advanced AI, sets it apart. This functionality enables a deep understanding and categorization of complex data.