Deep Learning - advanced AI chatbot service

🔵 The most advanced, sophisticated, intelligent, and complete Deep Learning expert.
AI-powered conversations at your fingertips
⚡️ Explain me the basics of Deep Learning
⏪ How does back propagation work?
👨🏽💻 Design an AI model for my use case
🧠 Teach me something about Deep Learning
Get Embed Code
Understanding Deep Learning
Deep learning is a subset of machine learning that mimics the workings of the human brain in processing data for tasks such as image recognition, speech recognition, and decision making. The basic function of deep learning models is to automatically learn and improve from experience by leveraging large neural networks with multiple layers (hence 'deep'). These models work by analyzing large amounts of data and creating complex representations through hierarchical learning, where each layer extracts features from the output of the previous one. For example, in image recognition, lower layers might detect edges, while higher layers might recognize more complex shapes, leading to the final classification (e.g., identifying a cat in a picture). Deep learning's design purpose is to automate and optimize tasks involving large, complex datasets, especially in areas where traditional machine learning struggles with feature extraction and scalability. Powered by ChatGPT-4o。
Core Functions of Deep Learning
Image and Video Recognition
Example
Deep learning models like convolutional neural networks (CNNs) are used for facial recognition, object detection, and medical imaging analysis.
Scenario
In healthcare, deep learning is applied to analyze medical images such as X-rays or MRIs to detect early signs of diseases like cancer. The model is trained on large datasets of medical images and can recognize patterns that may be difficult for humans to spot.
Natural Language Processing (NLP)
Example
Models like transformers (e.g., GPT, BERT) enable machines to understand and generate human language.
Scenario
Deep learning is used in chatbots, virtual assistants like Siri or Alexa, and for machine translation services like Google Translate. These systems process and generate human language, providing automated responses in real time.
Speech Recognition
Example
Recurrent Neural Networks (RNNs) and Long Short-Term Memory networks (LSTMs) are used to convert speech into text.
Scenario
Deep learning is behind voice-to-text applications and virtual assistants, allowing users to interact with devices through voice commands. For example, dictating a message to a smartphone.
Autonomous Vehicles
Example
Deep reinforcement learning is employed to train models that control self-driving cars.
Scenario
In autonomous vehicles, deep learning models process data from cameras, radar, and sensors to make real-time decisions about navigation, obstacle avoidance, and lane detection, ensuring safe driving.
Anomaly Detection
Example
Autoencoders or deep learning-based classifiers are used to detect unusual patterns in data.
Scenario
In cybersecurity, deep learning models are applied to detect anomalies in network traffic, helping identify potential cyber-attacks before they cause damage.
Recommendation Systems
Example
Deep learning models analyze user behavior to predict preferences and suggest content.
Scenario
Streaming platforms like Netflix and Spotify use deep learning to recommend movies, shows, or songs based on users' watching or listening history.
Target Users of Deep Learning Services
Data Scientists and Machine Learning Engineers
These professionals use deep learning to create predictive models and solve complex problems across various industries. They benefit from deep learning's ability to automatically extract features from raw data, reducing manual preprocessing work.
Healthcare Professionals
Doctors and radiologists use deep learning to assist in diagnosing diseases through the analysis of medical images and patient data. It enhances the accuracy and speed of diagnosis, particularly in areas like radiology and genomics.
Business Analysts
In sectors like finance and e-commerce, business analysts apply deep learning models to customer behavior analysis, fraud detection, and market trend prediction, which helps drive decision-making and optimize business processes.
Software Developers
Developers integrate deep learning into applications such as chatbots, virtual assistants, and personalized recommendation systems. They use frameworks like TensorFlow and PyTorch to implement models that offer smart features in their apps.
Autonomous System Developers
Engineers working on self-driving cars, drones, and robotics use deep learning to enable machines to perceive their environment and make decisions autonomously. They benefit from deep learning's ability to process real-time data efficiently.
Researchers and Academics
Researchers in fields like artificial intelligence, neuroscience, and cognitive science use deep learning to advance the understanding of complex phenomena, explore theoretical aspects of learning, and innovate in AI-driven technologies.
How to Use Deep Learning
Visit yeschat.ai for a free trial
Access yeschat.ai where you can try out advanced AI-powered chat solutions for free, without the need to log in or subscribe to ChatGPT Plus.
Set your objectives
Identify the specific problem you're addressing, whether it’s text generation, predictive modeling, or language understanding. This will determine the architecture and data you'll need.
Choose your framework
Pick a suitable Deep Learning framework like TensorFlow, PyTorch, or Keras. These libraries simplify neural network creation, backpropagation, and gradient descent.
Prepare and preprocess data
Gather the necessary dataset and preprocess it (normalization, encoding, etc.) to ensure it fits the input requirements of your chosen Deep Learning model.
Train, evaluate, and deploy
Set up your model, split data into training/validation sets, and train the model. Post-training, evaluate its performance using metrics like accuracy, precision, and loss. Deploy the model when satisfied.
Try other advanced and practical GPTs
Creador de Landing Pages Ganadoras
AI-driven content for winning landing pages

のInstagram Big Writer
Create Engaging Instagram Content with AI

Link Reader
AI-powered tool for reading and extracting key data

数据分析大师 - 可生成中文图表
AI-powered charts with Chinese text support

最全数学建模赛题助手
AI-powered solutions for mathematical modeling competitions

Nuxt
AI-Powered Web Development Simplified

GPTea
AI-powered celebrity news, games, and stories

PlusAI
AI-Powered Solutions for Your Needs

NEWS TRADE ASSISTANT V3
AI-powered trading insights for better decisions

رسم صورة حقيقية باستخدام الذكاء الاصطناعي
AI-powered image recreation tool for everyone
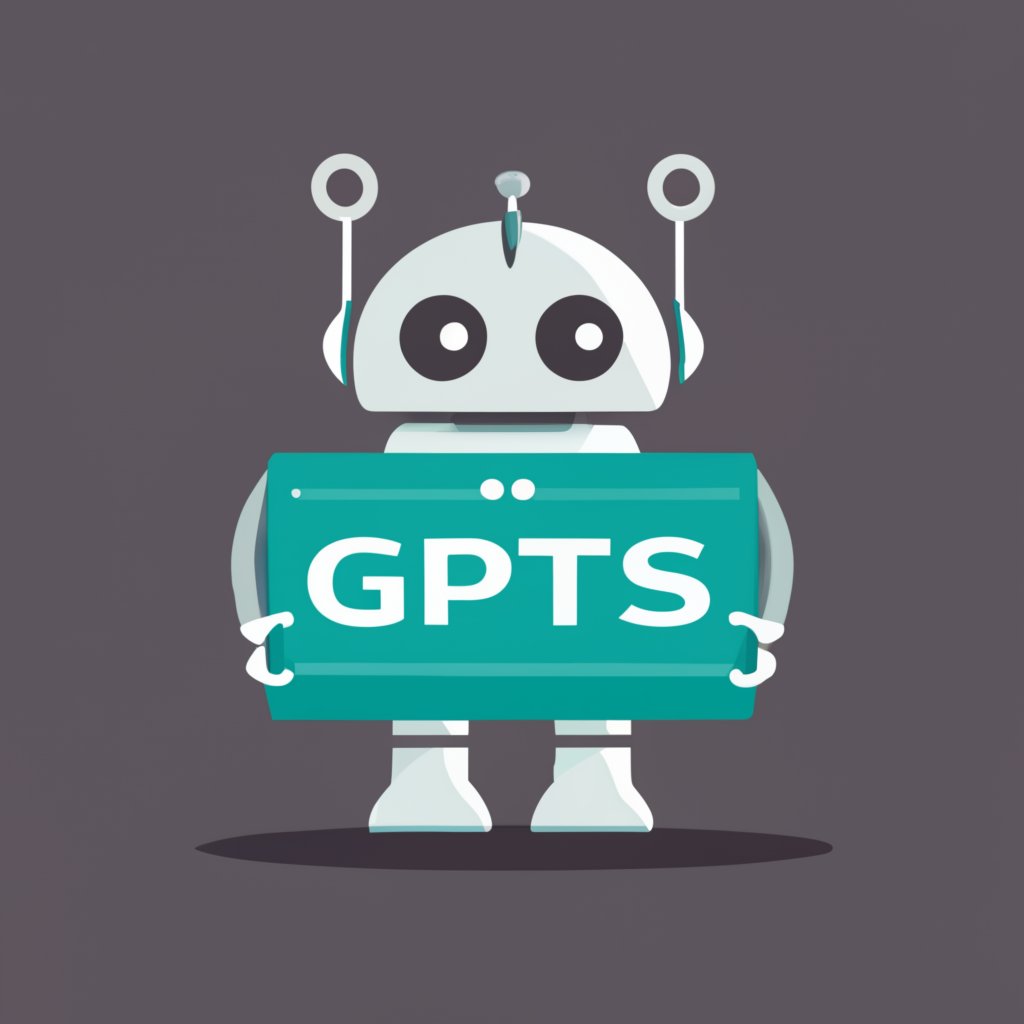
Math & Physics 👉🏼 Algebra Calculus Stats
AI-powered solutions for math & physics problems.

【占い】生年月日占い
AI-powered birthdate astrology predictions

Deep Learning Q&A
What is Deep Learning?
Deep Learning is a subset of machine learning where models learn from large amounts of data using neural networks. It excels in tasks like image recognition, natural language processing, and autonomous systems.
What frameworks are used for Deep Learning?
Popular Deep Learning frameworks include TensorFlow, PyTorch, and Keras. These libraries help simplify tasks like neural network construction, automatic differentiation, and data processing.
How is Deep Learning used in Natural Language Processing (NLP)?
In NLP, Deep Learning models such as LSTMs, GRUs, and Transformers are used for tasks like text generation, translation, sentiment analysis, and question answering, delivering human-like language capabilities.
What types of neural networks are there in Deep Learning?
Common types include Convolutional Neural Networks (CNNs) for image processing, Recurrent Neural Networks (RNNs) for sequence data, and Transformers, used for NLP and attention-based models.
What are the challenges of Deep Learning?
Challenges include the need for vast datasets, high computational resources, fine-tuning hyperparameters, and dealing with model interpretability and generalization issues.