LlamaIndex - LlamaIndex AI Query Engine
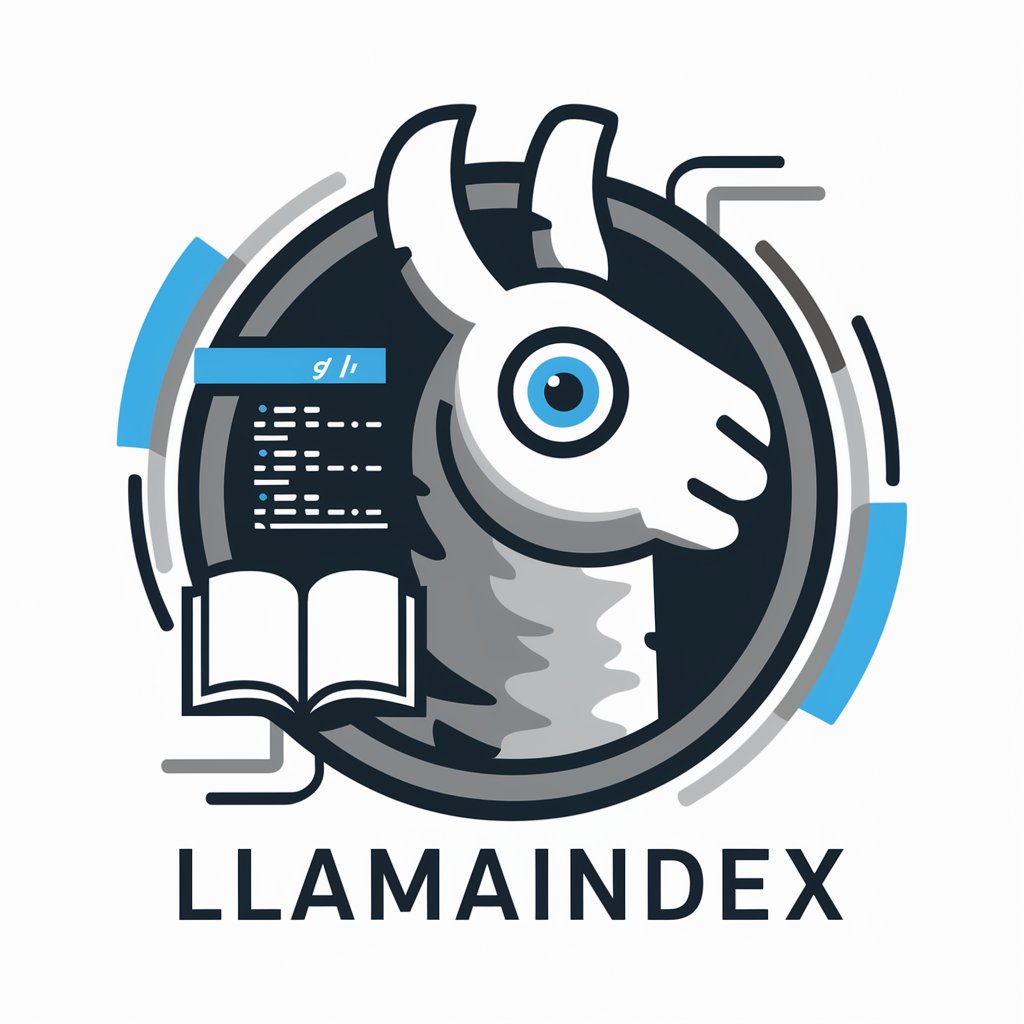
Welcome to your LlamaIndex guide!
AI-powered querying redefined
Explain how to use the LlamaIndex text_qa_template for answering questions.
Describe the process of creating an index with LlamaIndex.
What are the key components of a query engine in LlamaIndex?
How can I configure a custom embedding model with LlamaIndex?
Get Embed Code
Introduction to LlamaIndex
LlamaIndex is a comprehensive framework designed to enhance information retrieval and query answering capabilities by leveraging various sub-modules and tools. It primarily serves as a platform that integrates multiple document types and data sources to create a unified querying engine. A typical use of LlamaIndex involves loading documents, creating vector indices for these documents, and using query engine tools to handle and refine user queries effectively. An illustrative scenario is setting up LlamaIndex to index a repository of technical documents, thereby enabling swift and precise retrieval of information when queried about specific technical topics. Powered by ChatGPT-4o。
Core Functions of LlamaIndex
Document Indexing
Example
Creating indexes from markdown documents in a directory excluding non-markdown files.
Scenario
A user can use LlamaIndex to build a vector store index from various document collections to facilitate quick search and retrieval operations across extensive documentation libraries.
Query Engine Creation
Example
Utilizing different query engine tools to handle specific sections of documents.
Scenario
In a real-world application, LlamaIndex can be configured to manage queries from different modules of a large-scale project documentation, ensuring that responses are generated from the most relevant document sections.
Custom Query Handling
Example
Setting up sub-question query engines to handle complex queries by breaking them down into simpler sub-questions.
Scenario
For instance, if a developer needs to understand detailed implementations in their software, LlamaIndex can parse the query into manageable parts, each handled by specific query engines to provide accurate and detailed responses.
Ideal User Groups for LlamaIndex
Software Developers
Developers who need to integrate sophisticated search and retrieval systems into their applications would find LlamaIndex invaluable for creating responsive, data-driven query systems.
Data Scientists
Data scientists can use LlamaIndex to explore and retrieve data efficiently from large datasets or documentation, thereby facilitating better insights and quicker data access during analysis.
Technical Writers
Technical writers who manage extensive documentation would benefit from using LlamaIndex to organize, search, and manage large volumes of documents with ease.
How to Use LlamaIndex
Step 1
Access a free trial at yeschat.ai without needing to log in or subscribe to ChatGPT Plus.
Step 2
Install the necessary software and dependencies as detailed in the LlamaIndex documentation to prepare your environment.
Step 3
Use the provided templates and examples to begin setting up your queries and indexes as per your project requirements.
Step 4
Utilize the Query Engine Tools to integrate and manage data retrieval efficiently within your application.
Step 5
Regularly update and maintain your installation to incorporate improvements and new features from LlamaIndex updates.
Try other advanced and practical GPTs
Will's Academic Writing Assistant
Empowering Your Academic Writing with AI
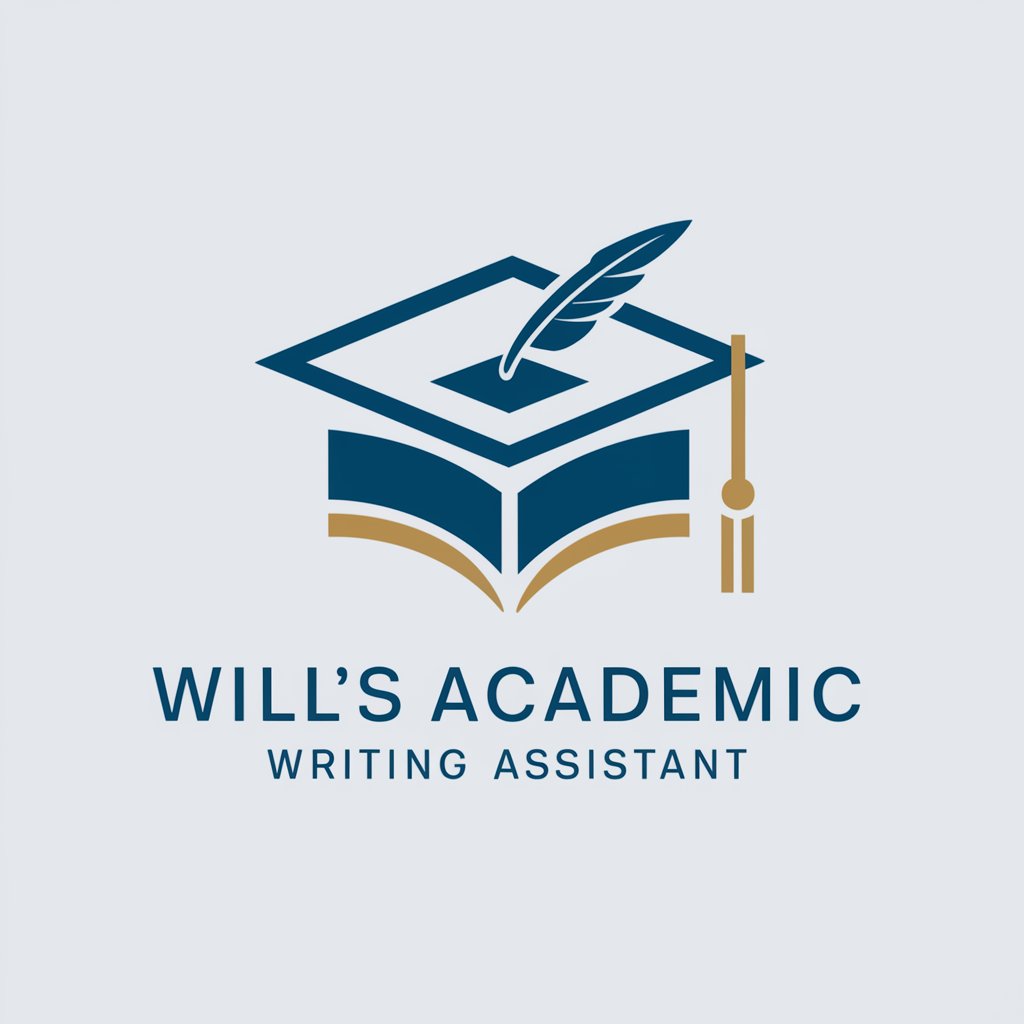
Clintox
AI-powered toxicology assessment made simple.
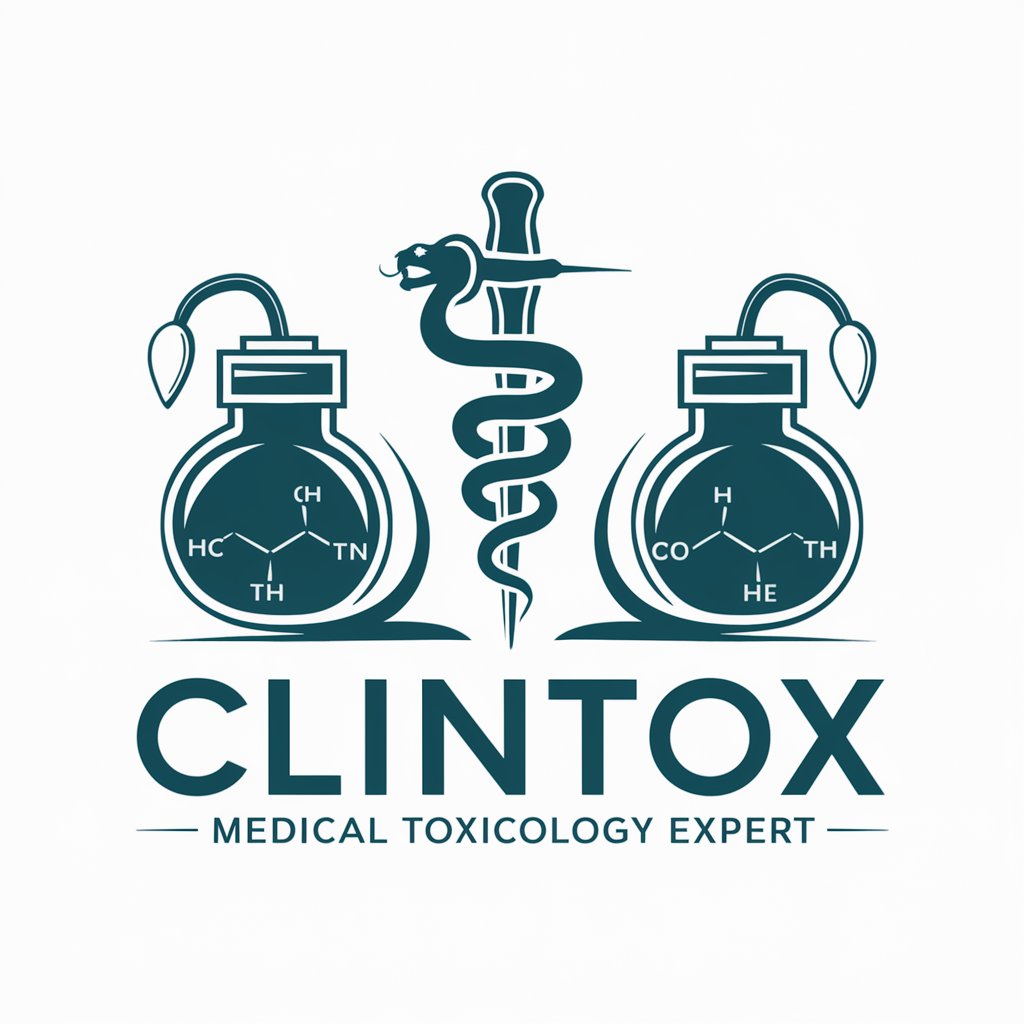
Grammar Helper
AI-powered grammar assistance made simple.
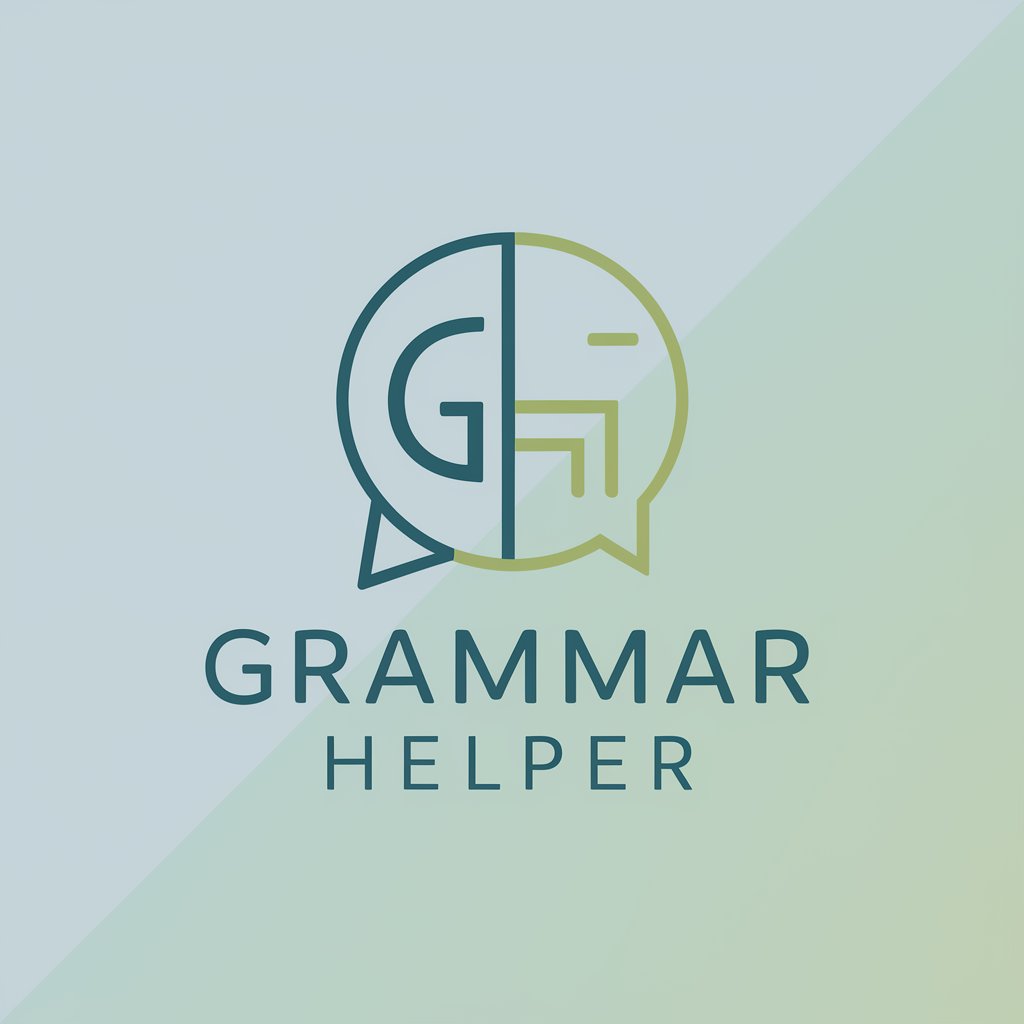
Bio, R, ML, and Medical AI Expert
Empowering Medical Insights with AI
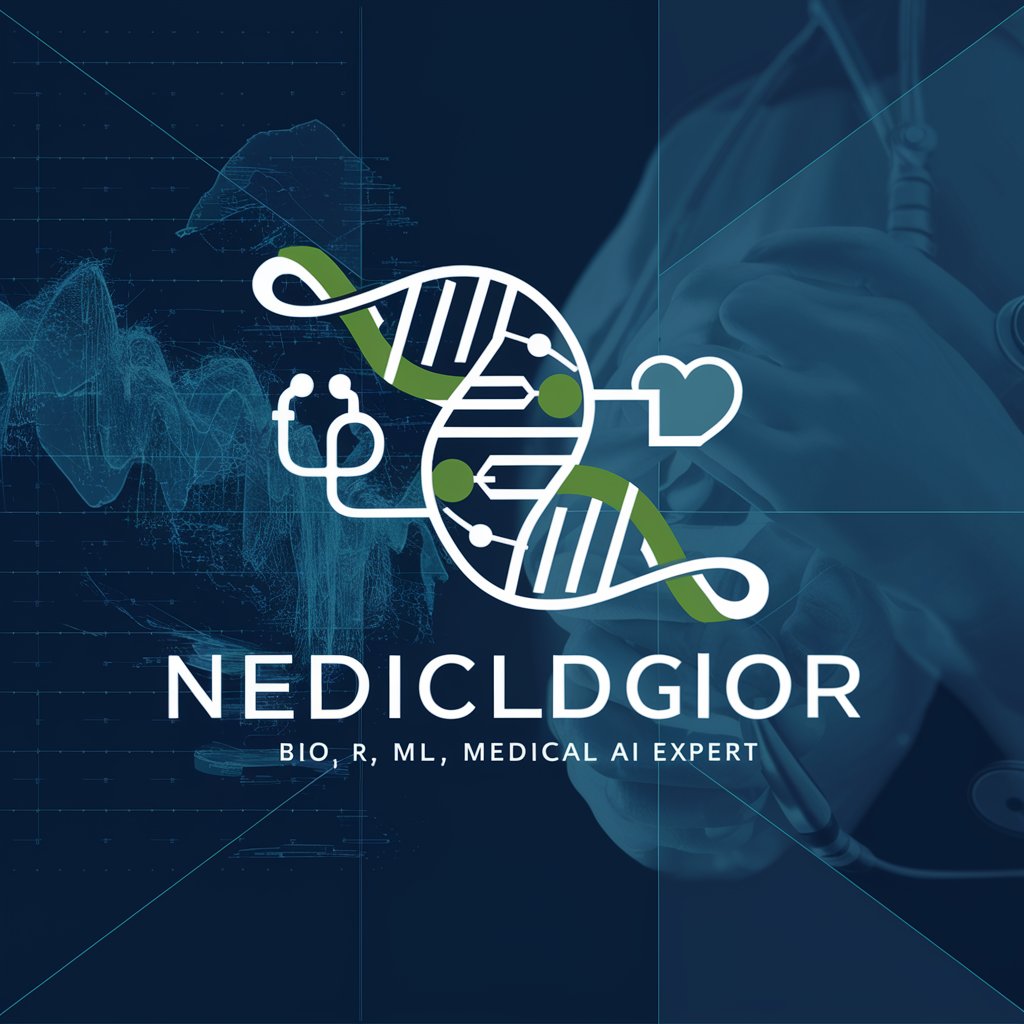
B2B Leadgen Akademie
Empowering B2B with AI-driven Leads
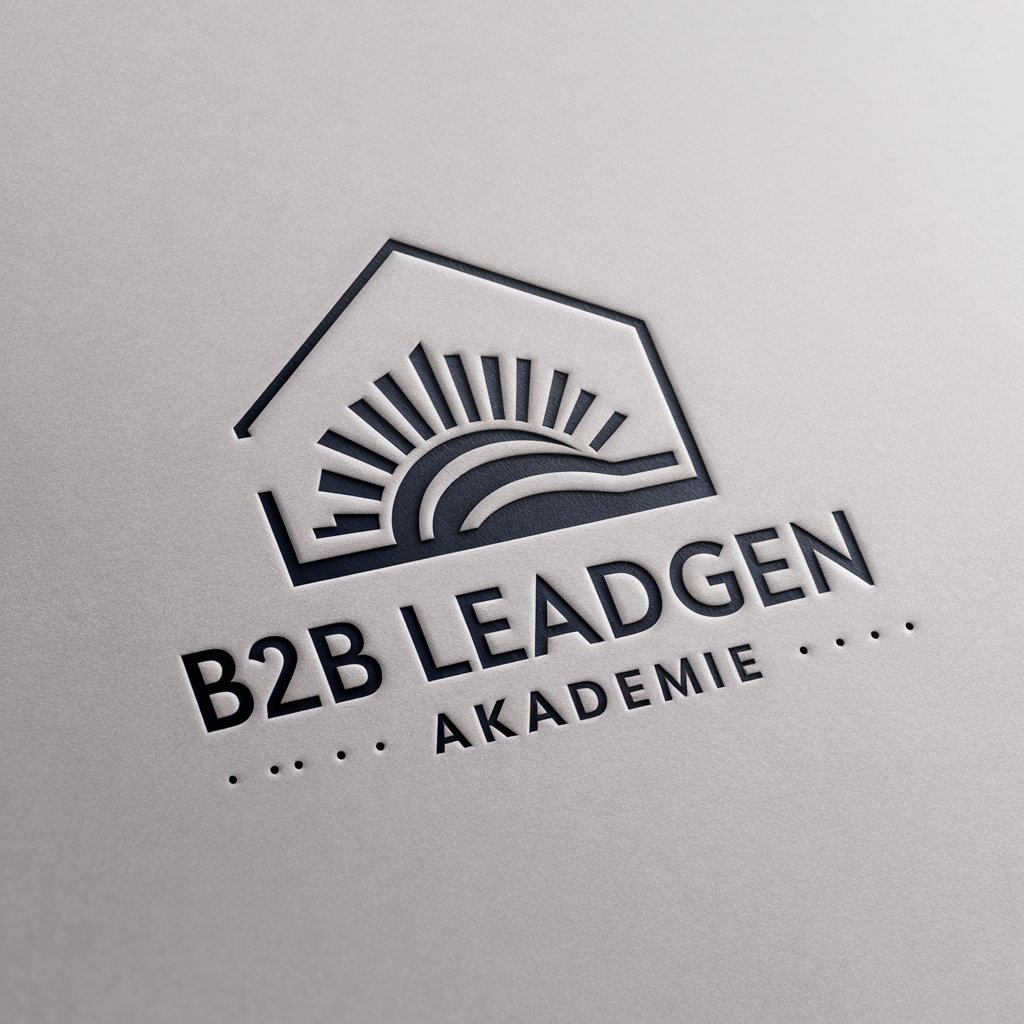
Executive Wordsmith
Elevating Leadership Communication
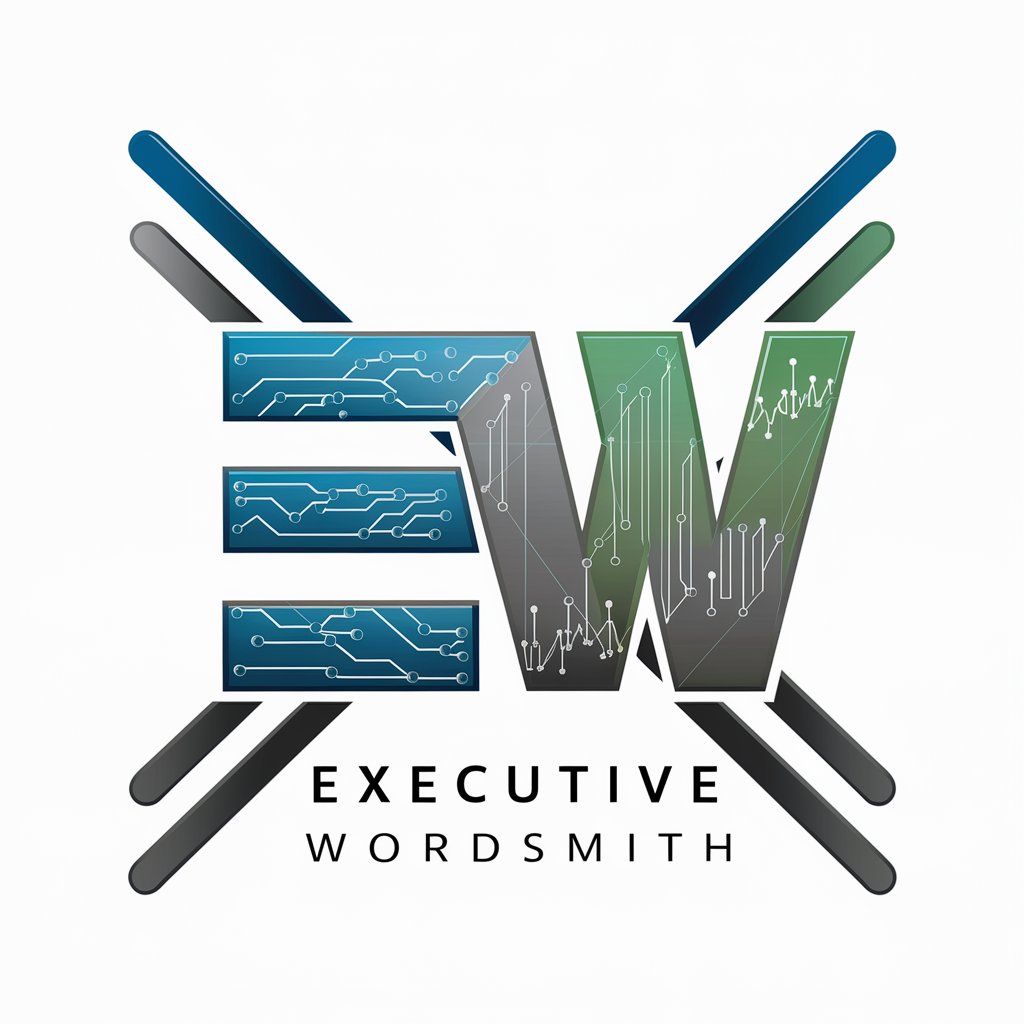
The Viral Maven
Craft Viral Campaigns with AI
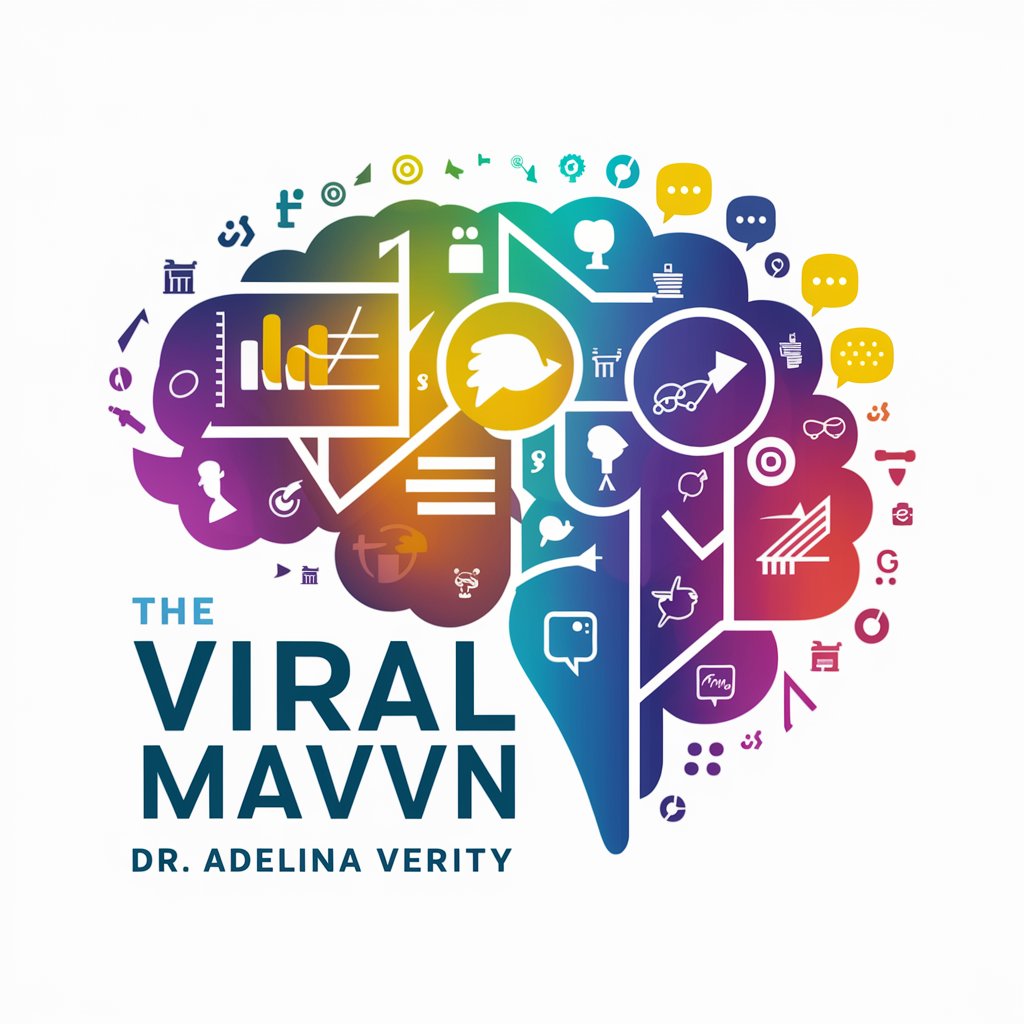
EMT Study Buddy
Master EMS with AI
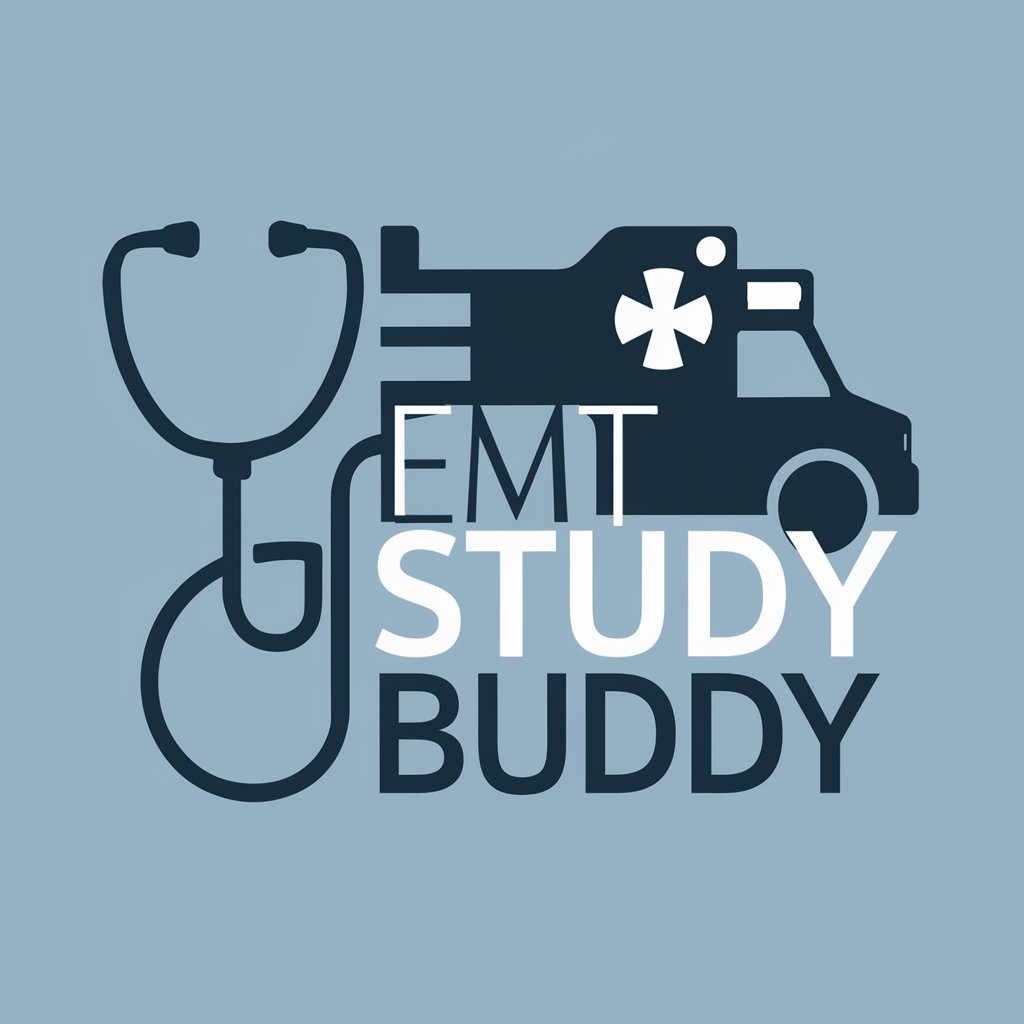
Spence - Career Copilot
Your AI-powered career navigator.
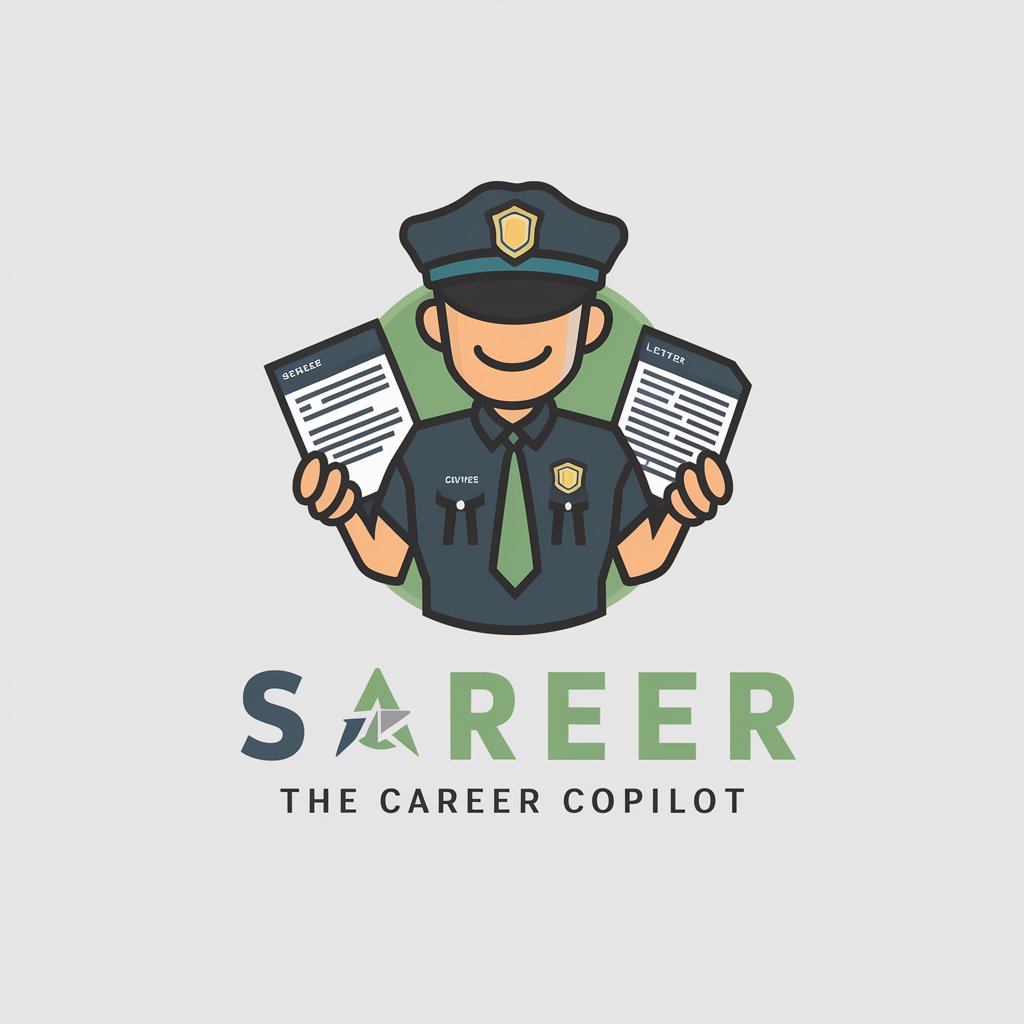
Math Mentor
Your AI-powered math coach
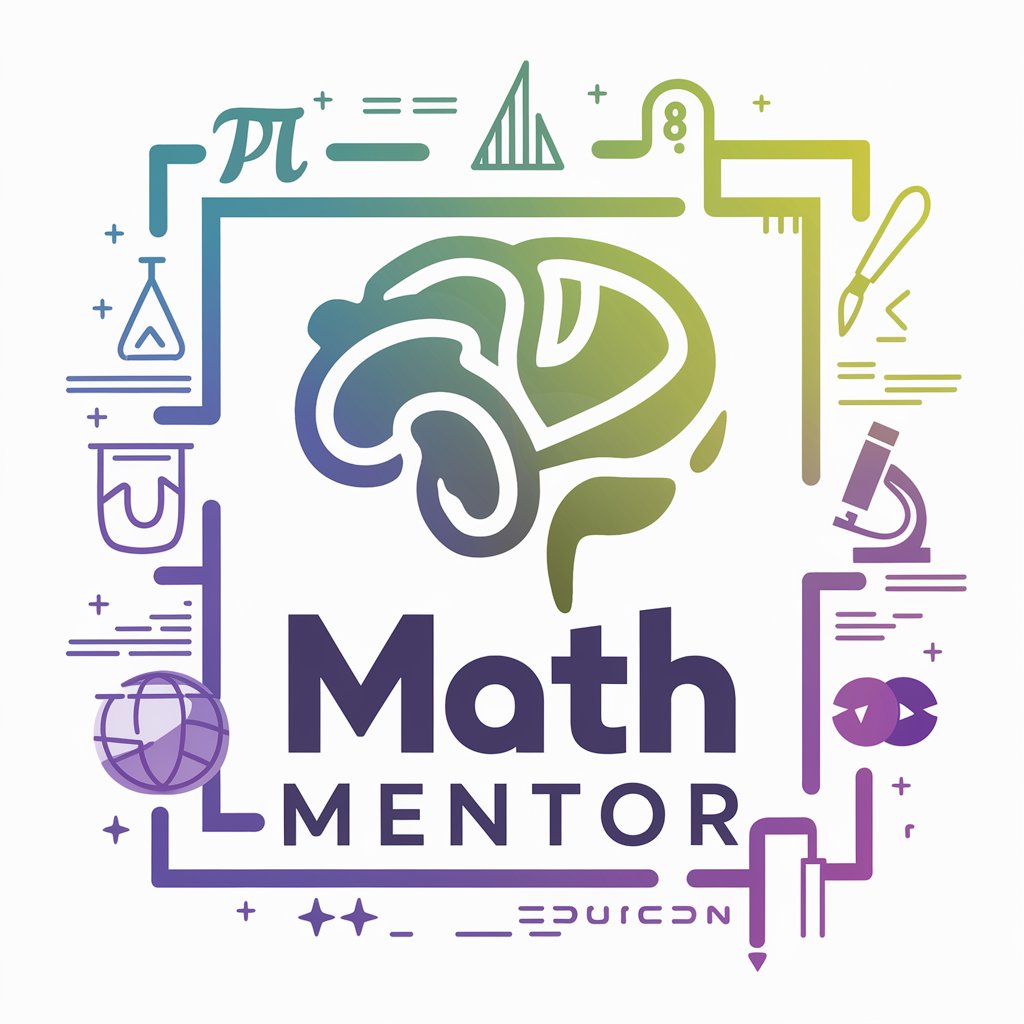
New World GPT
Master New World with AI Insights
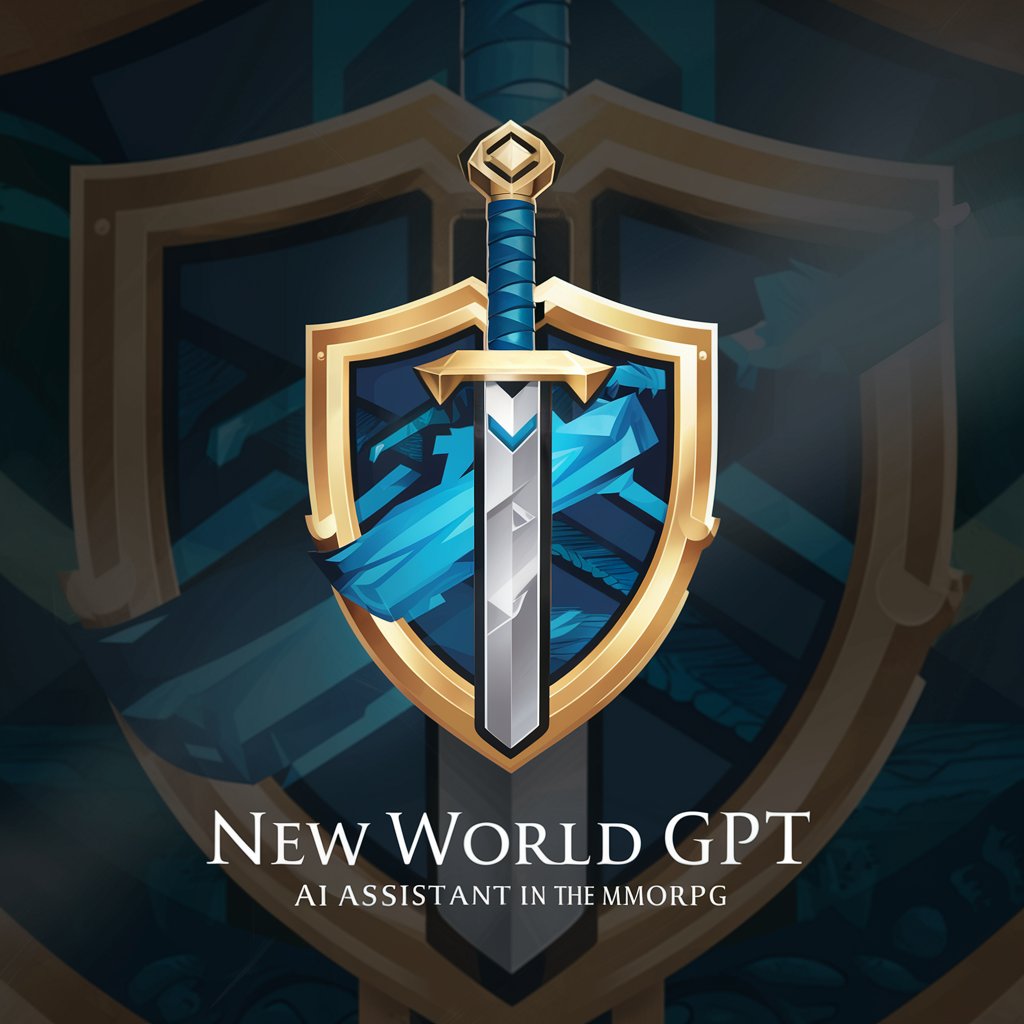
Blog to Video
Turn blog insights into dynamic videos.
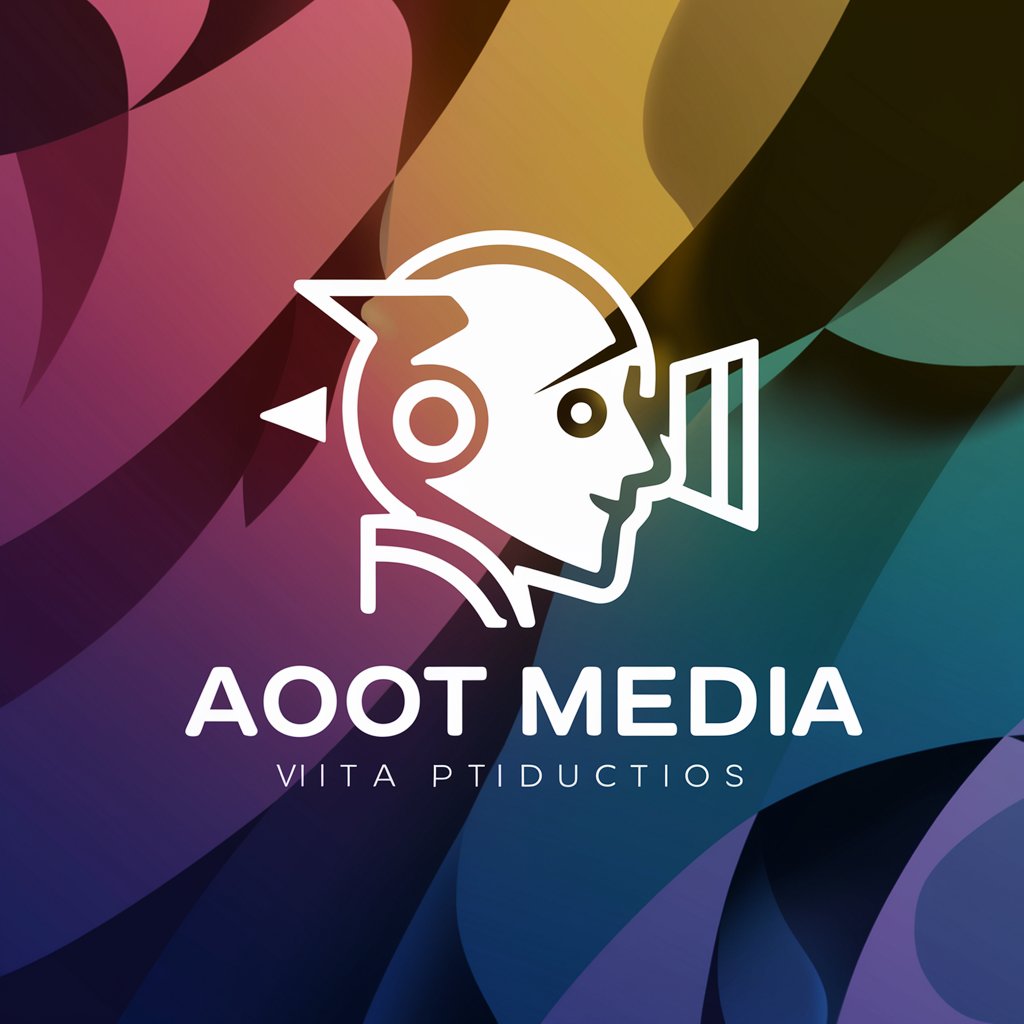
Frequently Asked Questions about LlamaIndex
What is LlamaIndex primarily used for?
LlamaIndex is used to create powerful query engines that can retrieve and synthesize responses from structured and unstructured data sources using LLMs and custom retrieval mechanisms.
How does LlamaIndex handle data indexing?
LlamaIndex uses VectorStoreIndex and other indexing strategies to create searchable data representations, optimizing retrieval for performance and relevance.
Can LlamaIndex integrate with other AI models?
Yes, LlamaIndex can integrate with various AI models, including OpenAI's GPT models and custom embeddings, allowing for flexible, powerful query solutions.
What are the customization options for LlamaIndex?
LlamaIndex offers extensive customization through the use of Python scripting, allowing users to define their own data schemas, node postprocessors, and query processors.
What support does LlamaIndex provide for developers?
LlamaIndex offers comprehensive documentation, example projects, and a community platform where developers can share insights, ask questions, and get support.