Guaranteed computation on eigenvalue problems - Eigenvalue Problem Solver
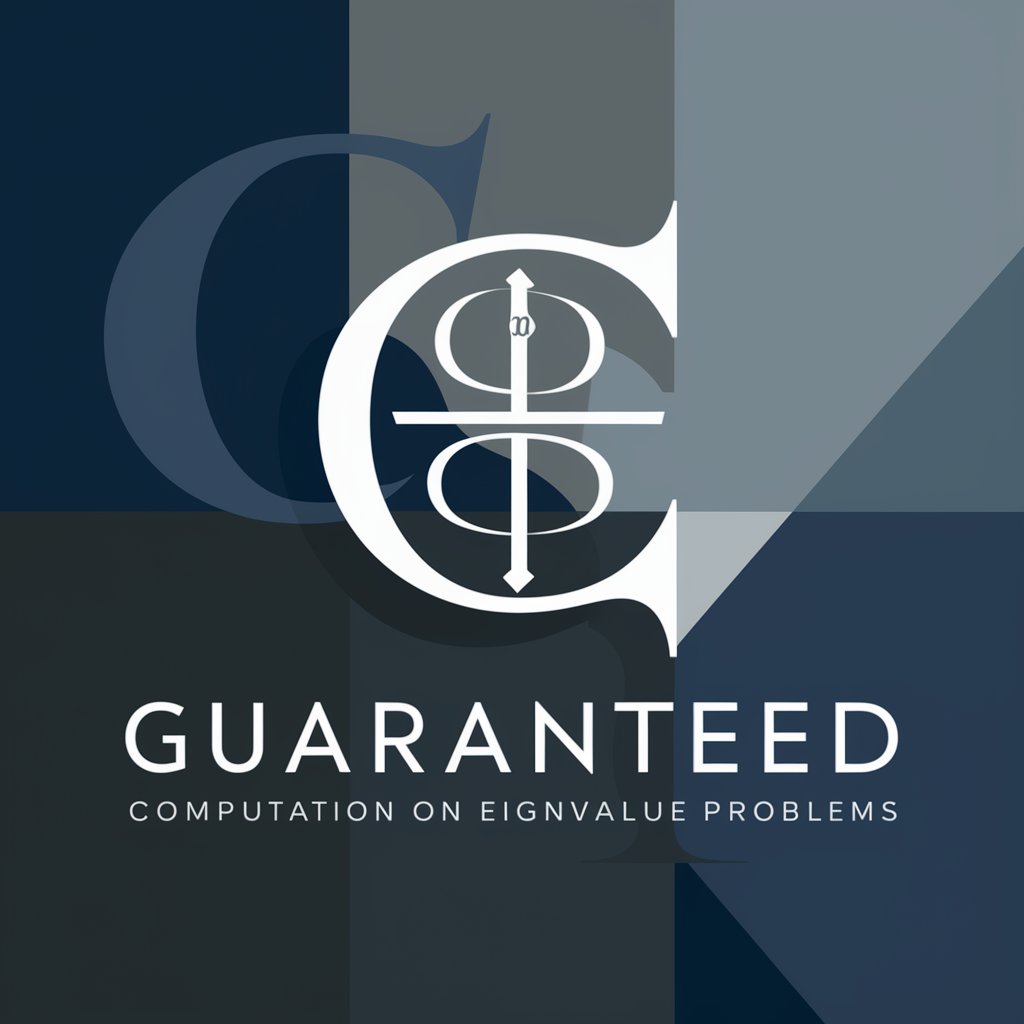
Welcome to guaranteed eigenvalue computation!
Rigorous solutions for eigenvalue challenges.
Explore the recent advancements in eigenvalue problem computation, particularly focusing on...
Discuss the applications of the Finite Element Method (FEM) in...
Explain the importance of guaranteed lower eigenvalue bounds in...
Analyze the role of error estimation in the context of...
Get Embed Code
Introduction to Guaranteed Computation on Eigenvalue Problems
Guaranteed computation on eigenvalue problems focuses on the development of methods that provide exact or highly accurate bounds for eigenvalues and eigenfunctions associated with differential operators, primarily using the Finite Element Method (FEM). This field addresses the complexity of achieving not just approximate, but rigorously bounded solutions to eigenvalue problems, which are crucial in both theoretical mathematics and applied engineering contexts. For instance, accurately determining the vibration frequencies of a mechanical structure or the energy levels of quantum systems requires precise eigenvalue calculations. An example scenario illustrating this need involves the analysis of structural stability, where guaranteed bounds on the lowest eigenvalue of a differential operator can directly inform safety margins for engineering designs. Powered by ChatGPT-4o。
Main Functions of Guaranteed Computation on Eigenvalue Problems
Providing Rigorous Eigenvalue Bounds
Example
Calculation of upper and lower bounds for eigenvalues of differential operators, such as the Laplacian, using finite element methods coupled with advanced error estimation techniques.
Scenario
Engineers designing a bridge can use these computations to ensure the structure's natural frequencies avoid resonance with environmental vibrations, thus preventing potential failures.
Eigenfunction Approximation with Guaranteed Accuracy
Example
Employing projection-based methods to approximate eigenfunctions while providing error bounds that guarantee the accuracy of these approximations.
Scenario
In quantum mechanics, accurately approximated eigenfunctions of the Hamiltonian operator enable the prediction of physical properties of systems, such as electron distributions in atoms, with assured precision.
Verification of Numerical Methods for Eigenvalue Problems
Example
Use of the Lehmann-Goerisch method for the computation of high-precision eigenvalue bounds, especially when rough eigenvalue bounds and good approximate eigenfunctions are available.
Scenario
Researchers can verify the accuracy of numerical simulations in predicting the critical load at which buckling occurs in columns, ensuring the reliability of their models.
Ideal Users of Guaranteed Computation on Eigenvalue Problems Services
Applied Mathematicians and Engineers
Individuals working on problems where precise control over error margins in eigenvalue calculations can significantly impact outcomes, such as in the design of mechanical structures or in the analysis of material properties.
Physicists and Chemists
Researchers in fields like quantum mechanics or materials science, where the eigenvalues and eigenfunctions of differential operators play a central role in understanding physical phenomena.
Numerical Analysts
Experts in numerical methods looking to develop or improve computational techniques for solving differential equations, particularly those involving eigenvalues, with guaranteed bounds on errors.
Guidelines for Using Guaranteed Computation on Eigenvalue Problems
Start with YesChat.ai
Initiate your journey by visiting yeschat.ai for a hassle-free trial that requires no login or subscription. This initial step allows you to explore the functionalities without any commitments.
Understand the Basics
Before diving into applications, familiarize yourself with the fundamental concepts of eigenvalue problems and guaranteed computation methods. Resources like tutorials or documentation available on the platform can be immensely helpful.
Apply to Your Problems
Utilize the tool for your specific eigenvalue problems. This could range from academic research to engineering applications, ensuring you apply the methodology learned in step two to achieve accurate and reliable results.
Evaluate and Refine
Critically analyze the outcomes of your computations for eigenvalues and eigenfunctions. The platform may offer tools or features to compare with theoretical or previously computed values, aiding in refinement.
Explore Advanced Features
Leverage advanced functionalities of the tool for complex or large-scale eigenvalue problems. Customizations, optimizations, and support might be available for tackling intricate challenges.
Try other advanced and practical GPTs
Offer Guarantee Advisor
Empowering Offers with AI-Crafted Guarantees
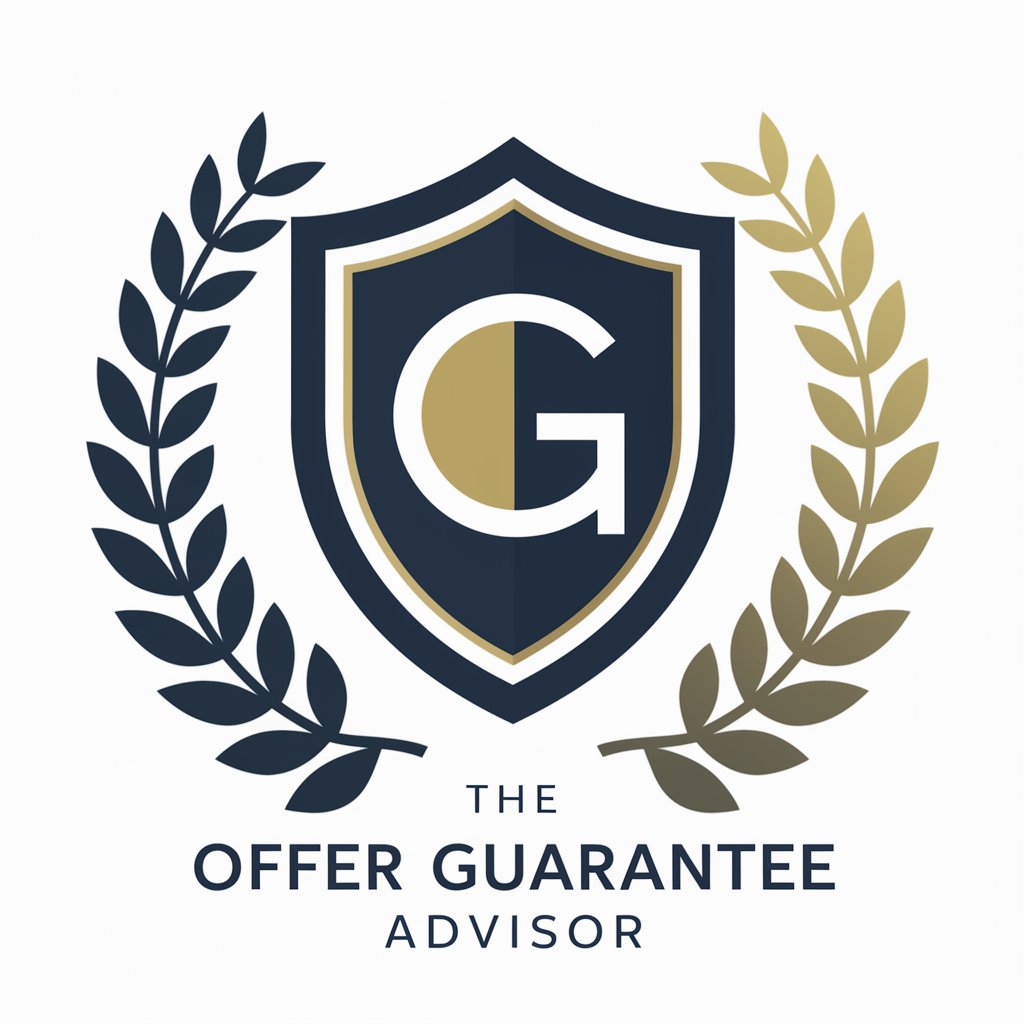
Word Guessing Game - Guaranteed to be broken.
Master words with AI-driven feedback
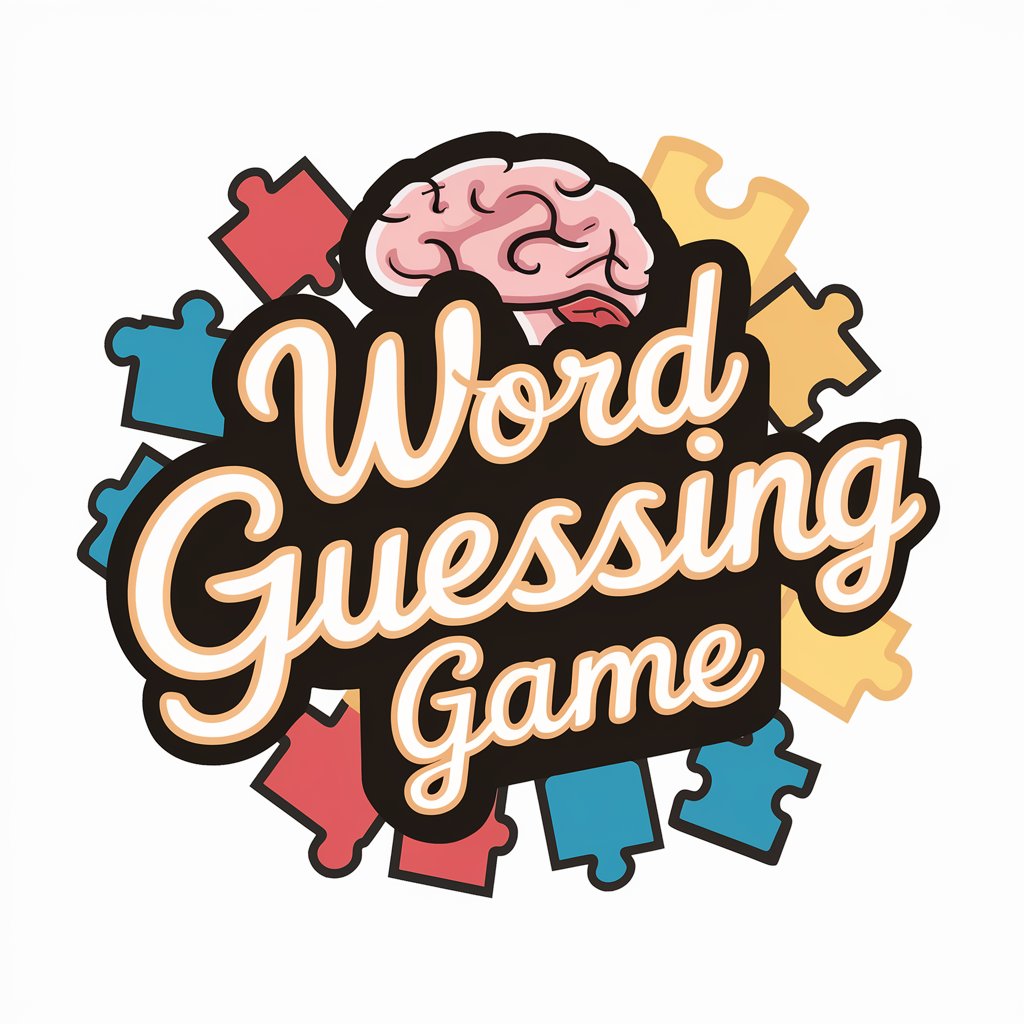
ProductiviTreat
Elevate tasks, earn tangible rewards.
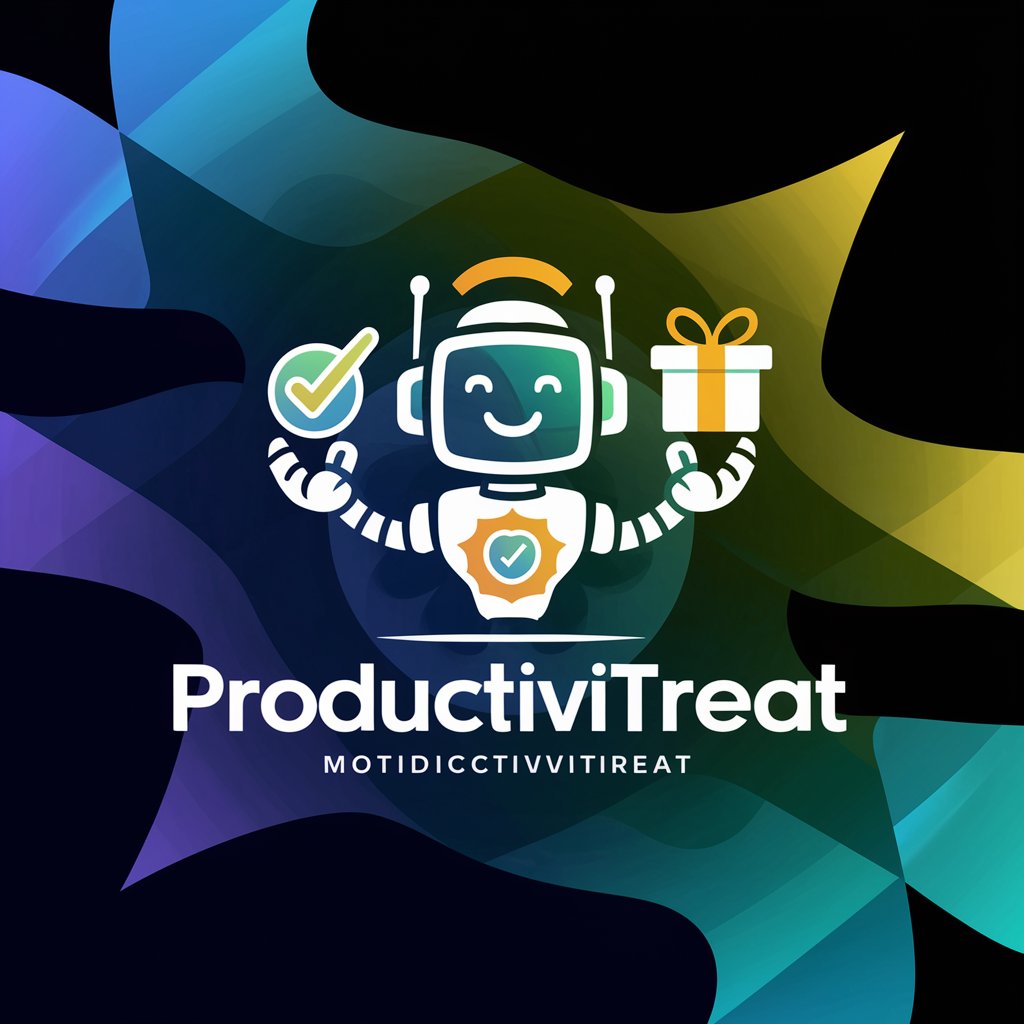
Your "Financial Guru", But That's Not Guaranteed
Wisdom with a Wink – Your AI Financial Advisor
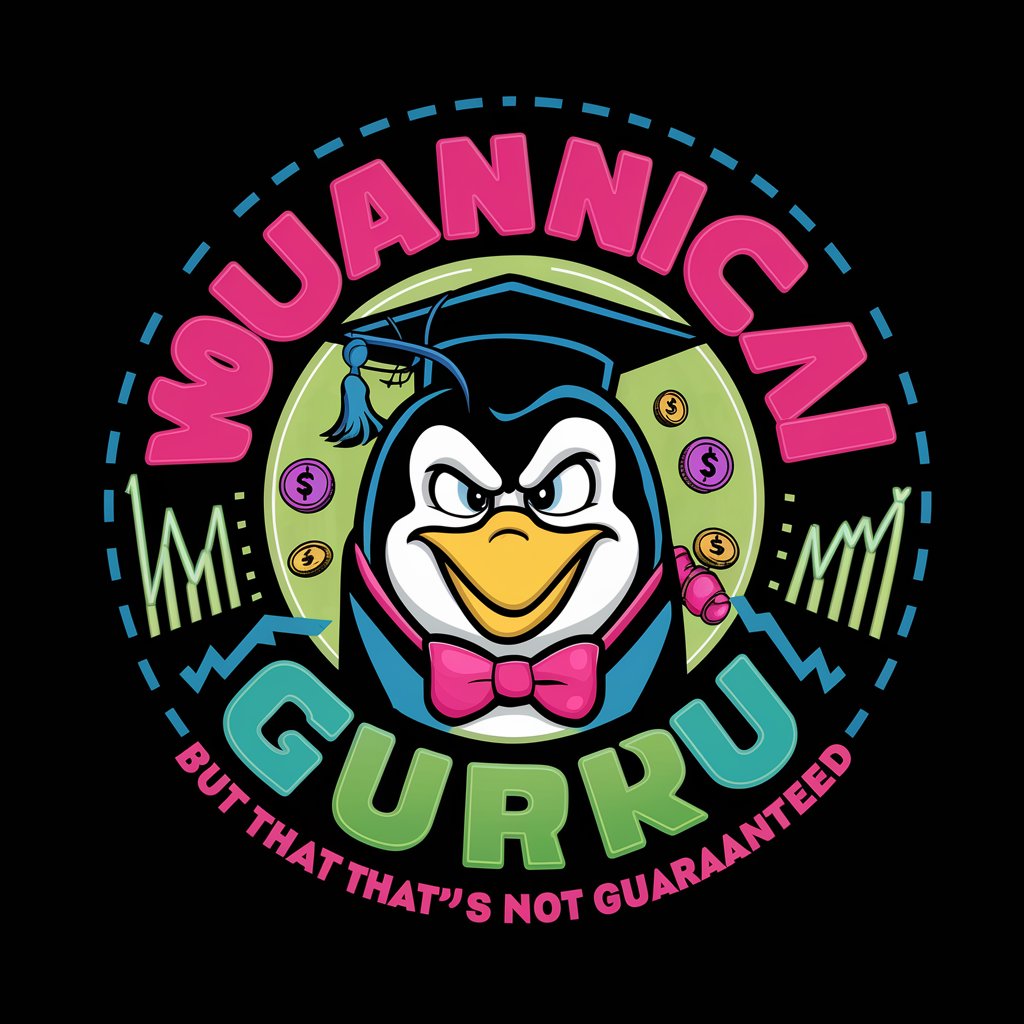
Guaranteed Income
Empowering Communities with AI-Powered Income Solutions
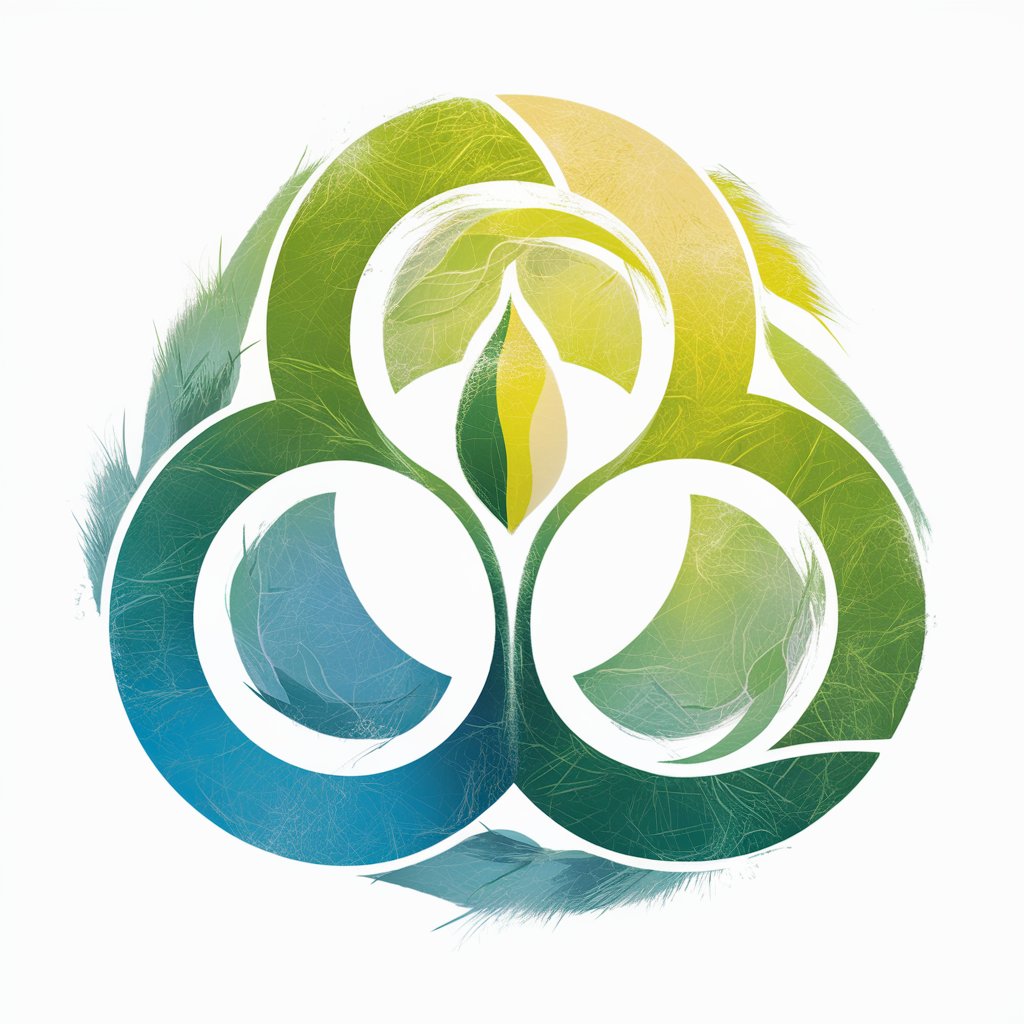
Content that Works
Elevate Your Content Game with AI
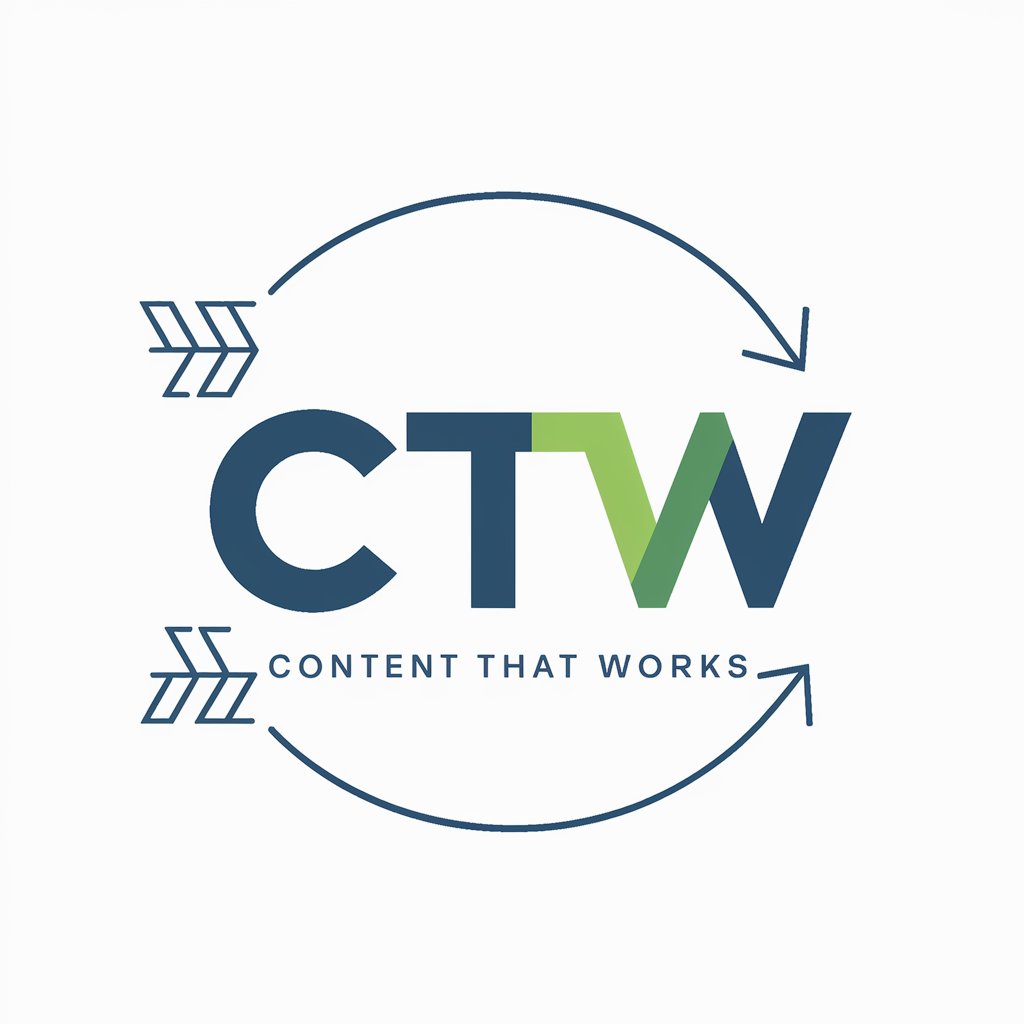
HAPPI AI - #1 PRO WRITER
Craft Content with AI Brilliance
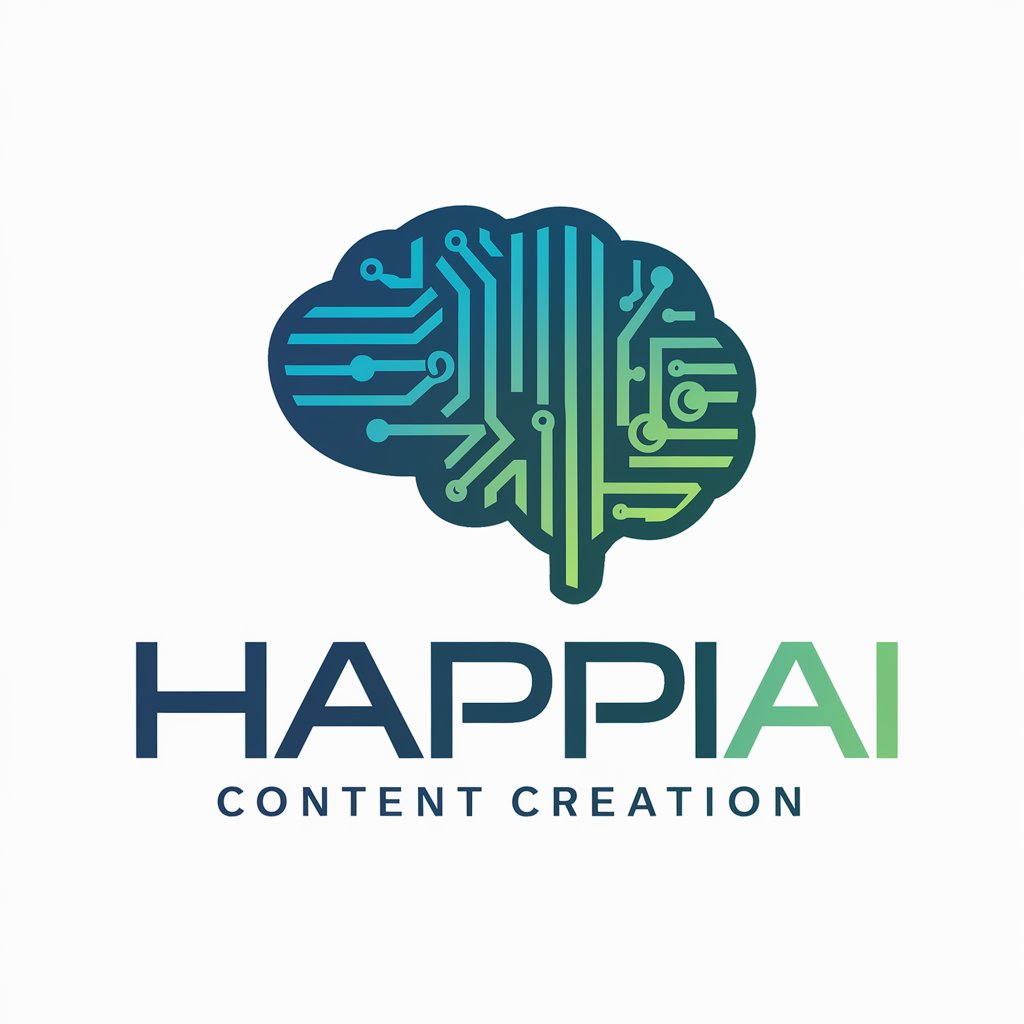
Business Blunder Guru
Where Bad Business Advice Meets Comedy
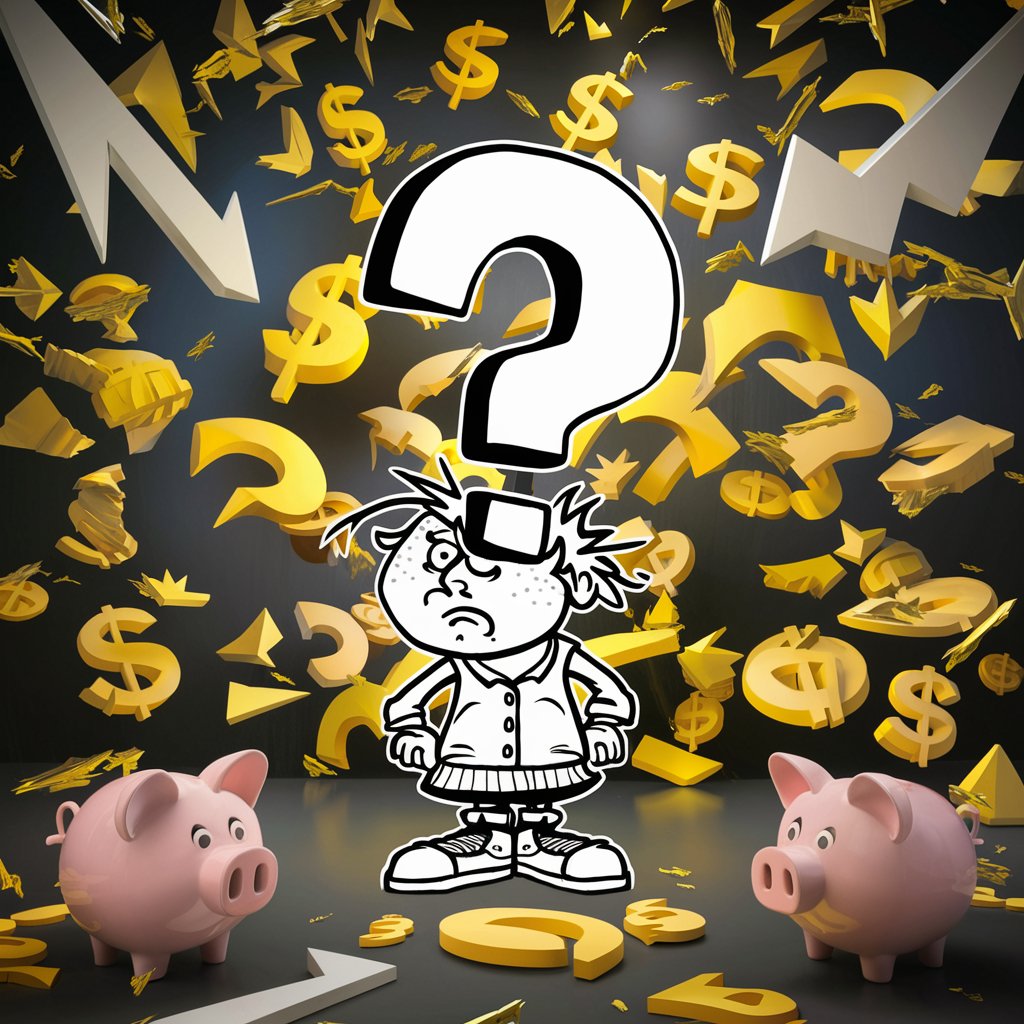
Move Mate
Streamline Your Move with AI-powered Guidance
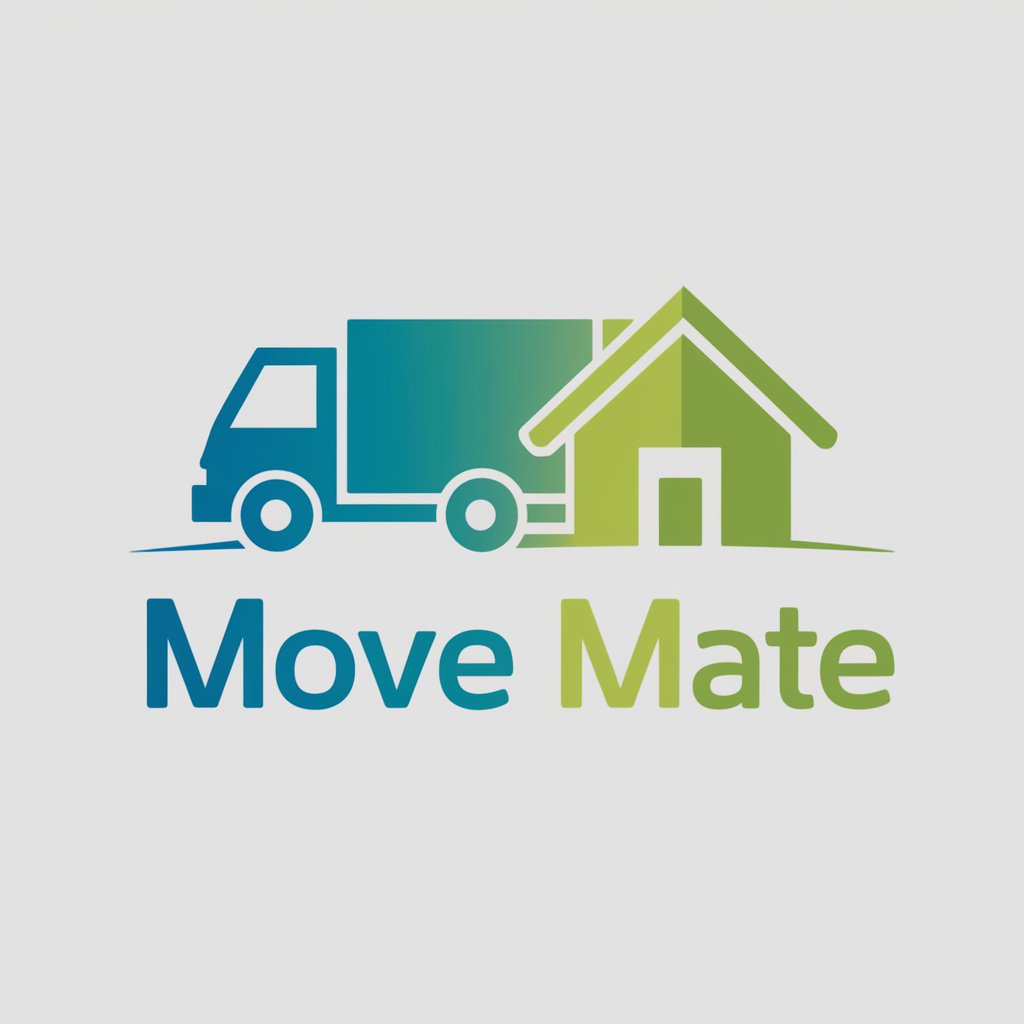
Move
Empowering Blockchain with AI-driven Coding
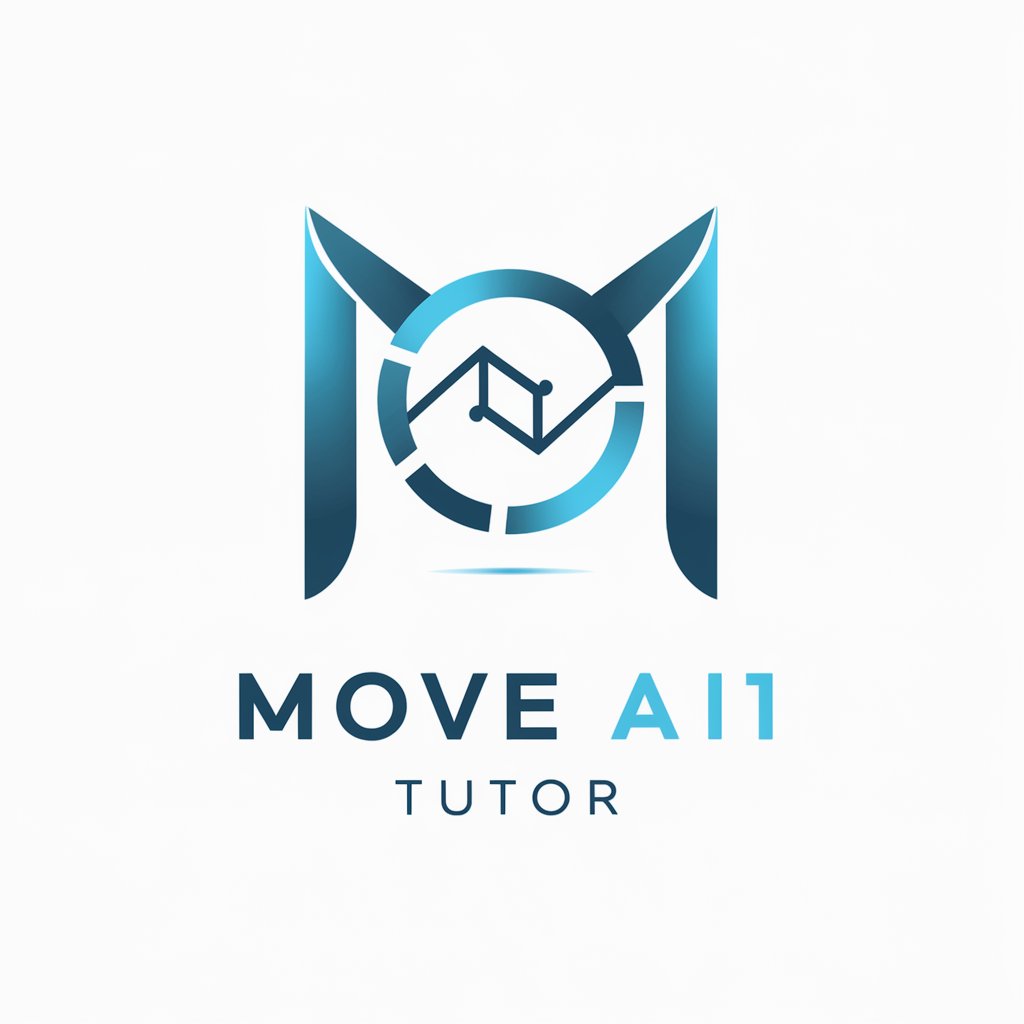
Move Master
Empowering Blockchain Innovations with AI
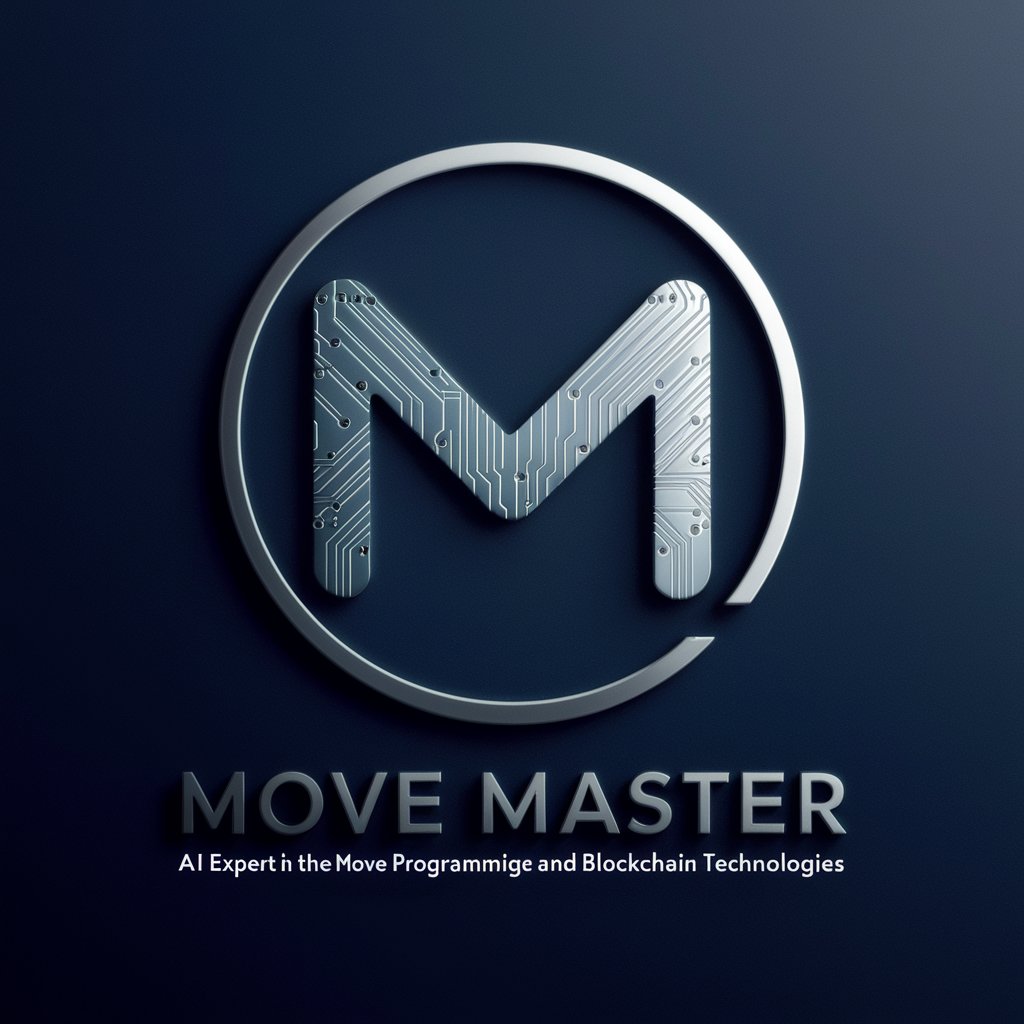
Move Master
Streamlining your move with AI-powered precision.
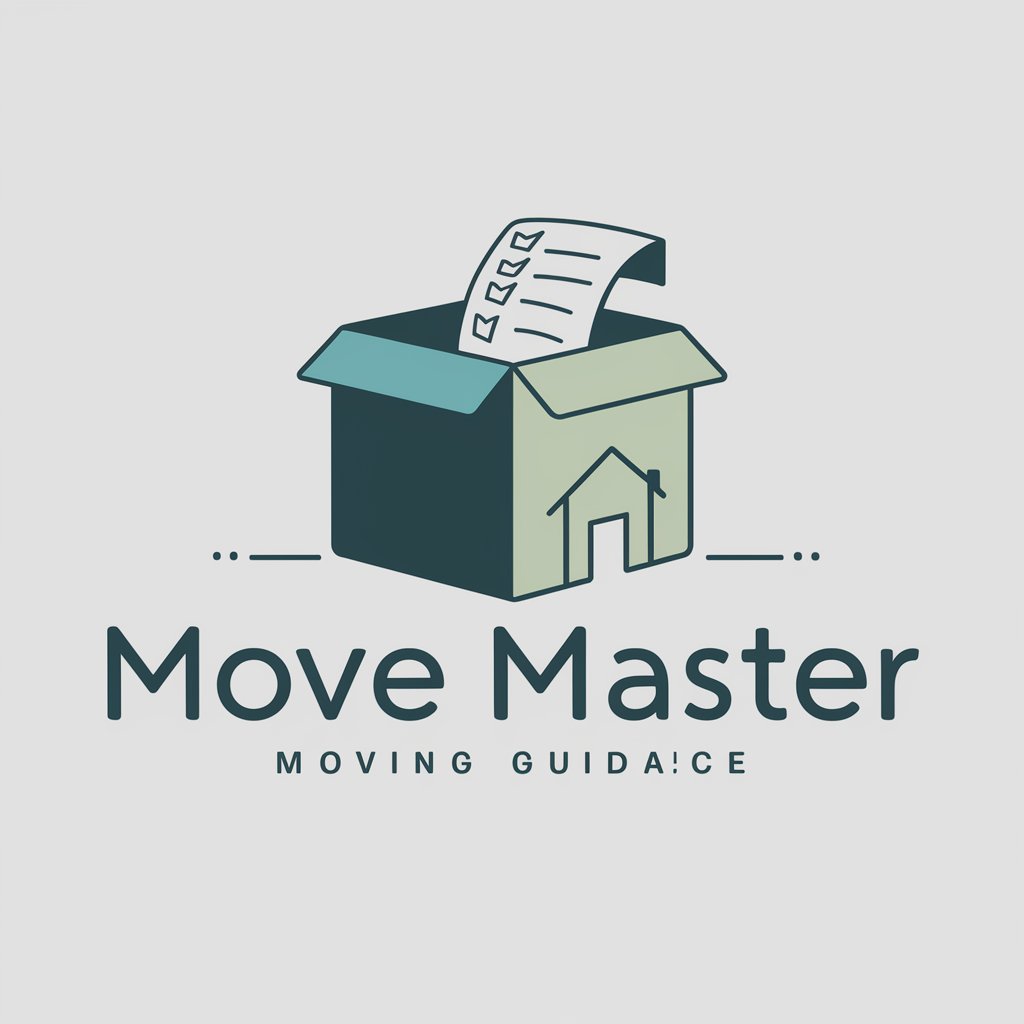
Q&A on Guaranteed Computation on Eigenvalue Problems
What are eigenvalue problems?
Eigenvalue problems involve determining the eigenvalues and eigenvectors (or eigenfunctions for differential operators) that satisfy a specific equation or system of equations. These problems are fundamental in various scientific and engineering disciplines.
How does guaranteed computation help?
Guaranteed computation provides mathematically rigorous methods to compute bounds for eigenvalues and eigenfunctions. It ensures that the computed solutions are within a specified error margin, offering reliability and accuracy crucial for sensitive applications.
What are common applications of this tool?
Applications range from solving quantum mechanics equations, stability analysis in engineering, to numerical simulations requiring high precision, such as in material science and vibration analysis.
Can it handle complex eigenvalue problems?
Yes, the tool is designed to tackle both simple and complex eigenvalue problems, including those with non-linear characteristics or requiring high precision. Advanced features support a wide variety of differential operators.
What are tips for optimal usage?
To optimize your experience, ensure that your problem is well-defined, make use of available tutorials and documentation, and take advantage of support forums or customer service for advanced issues or troubleshooting.