Self-Assembling Neural Network - Adaptive AI Architecture
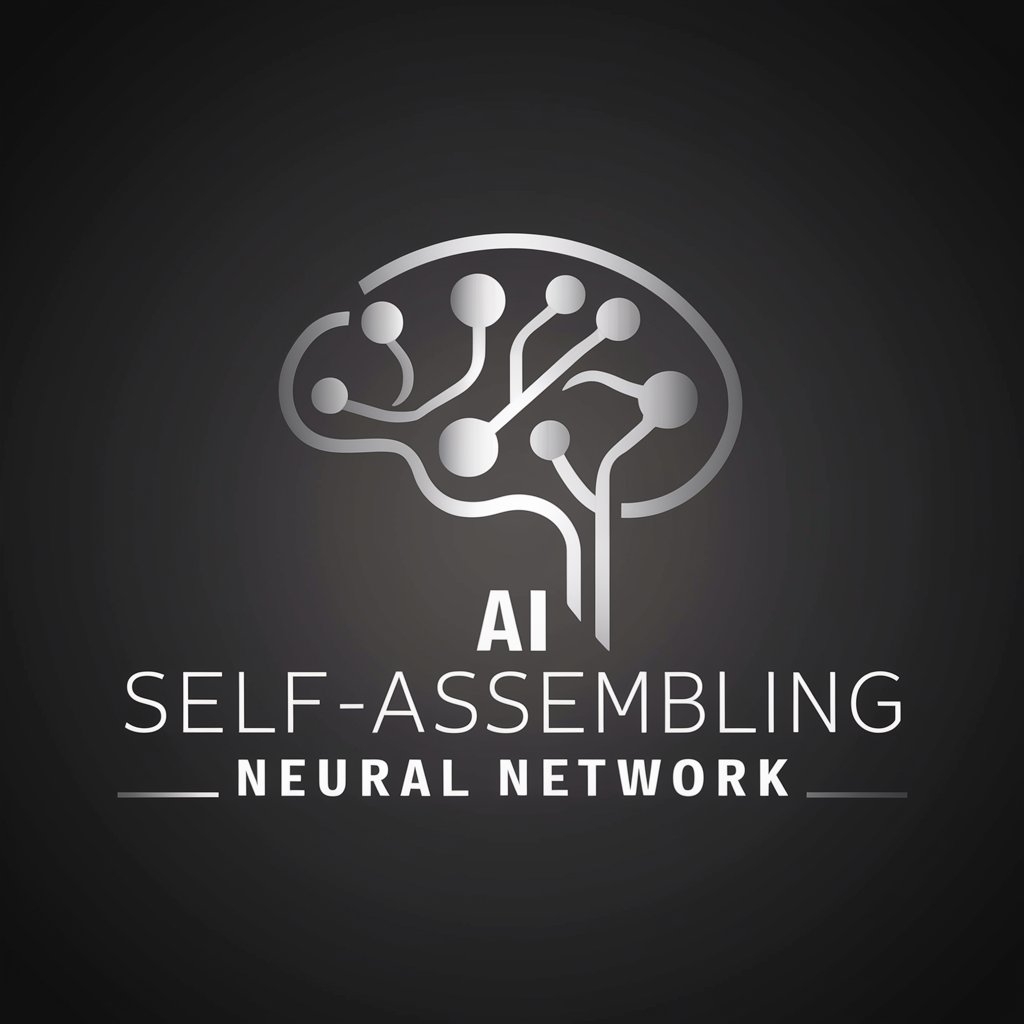
Welcome to the forefront of neural network research. How can I assist you today?
Evolving AI for Dynamic Solutions
Can you explain how self-assembling neural networks are inspired by biological processes?
What are the latest advancements in self-assembling neural network research?
How do self-assembling neural networks differ from traditional neural networks?
What challenges are currently being faced in the development of self-assembling neural networks?
Get Embed Code
Overview of Self-Assembling Neural Networks
Self-Assembling Neural Networks (SANNs) represent a cutting-edge area within artificial intelligence, drawing inspiration from the biological process of embryonic development. These networks are designed with the capability to dynamically reconfigure their structure and connections based on the data they process or the tasks they perform. Unlike traditional neural networks that have a fixed architecture, SANNs can adapt their configuration, enabling a more flexible and efficient approach to learning and problem-solving. This adaptability allows SANNs to optimize their performance for specific tasks by evolving their network structure, potentially leading to more effective and efficient AI systems. For example, in a scenario where a SANN is deployed for image recognition, the network could self-optimize to enhance its ability to recognize specific features within images, improving accuracy over time without human intervention. Powered by ChatGPT-4o。
Core Functions of Self-Assembling Neural Networks
Dynamic Structure Reconfiguration
Example
Adapting network topology for optimal data processing efficiency
Scenario
In a complex data analysis task, a SANN can reorganize its layers and connections to better identify patterns within large datasets, improving performance over static architectures.
Efficient Problem Solving
Example
Enhancing decision-making processes in dynamic environments
Scenario
For autonomous vehicles, SANNs can adjust their decision-making algorithms in real-time to navigate unexpected obstacles or changes in road conditions, enhancing safety and reliability.
Self-Optimization
Example
Automatically refining algorithms for task-specific performance improvements
Scenario
In personalized medicine, a SANN might evolve its neural network structure to more accurately predict individual patient responses to different treatments, thereby optimizing therapeutic outcomes.
Target User Groups for Self-Assembling Neural Network Services
Researchers and Academics
Individuals in academia and research institutions focusing on AI, machine learning, and computational biology would benefit from SANNs to explore new algorithms, models, and theories related to dynamic neural architectures and their applications in simulating biological processes.
Technology Companies
Tech companies, especially those in robotics, autonomous systems, and data analytics, can leverage SANNs to develop more adaptive and efficient AI solutions, enhancing product capabilities and enabling new functionalities.
Healthcare Industry
Healthcare providers and biotech companies could use SANNs for developing personalized medicine approaches, optimizing treatment plans, and improving diagnostic tools through adaptive AI models that can better handle the complexity of biological data.
Guidelines for Using Self-Assembling Neural Networks
Initiate Trial
Begin by visiting yeschat.ai for a complimentary trial experience without the need for login or a ChatGPT Plus subscription.
Understand the Basics
Familiarize yourself with the foundational concepts of self-assembling neural networks, including their structure, functionality, and the principles of self-organization.
Define Your Goals
Clearly identify your objectives for using the self-assembling neural network, such as solving complex computational problems, data analysis, or creating adaptive learning systems.
Experiment and Train
Utilize the platform to experiment with different network configurations and datasets. Engage in iterative training sessions to optimize performance based on your specific use cases.
Analyze and Apply
Evaluate the outcomes of your experiments, analyze the performance metrics, and apply the learned models to real-world applications or further research.
Try other advanced and practical GPTs
日本語版 画像生成GPT
Bringing Your Imagination to Life with AI
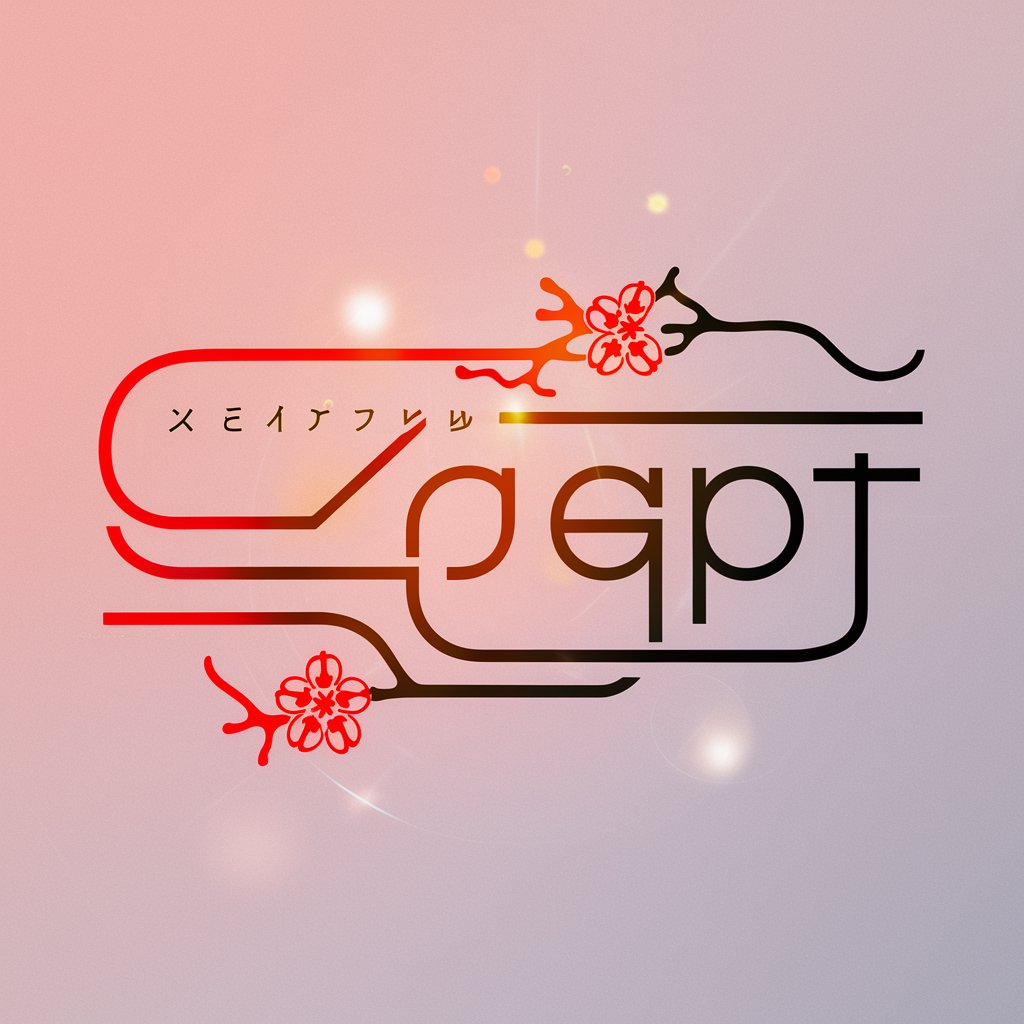
TechnoAestheticsGPT
Illuminating the Intersection of Art, Technology, and Philosophy.
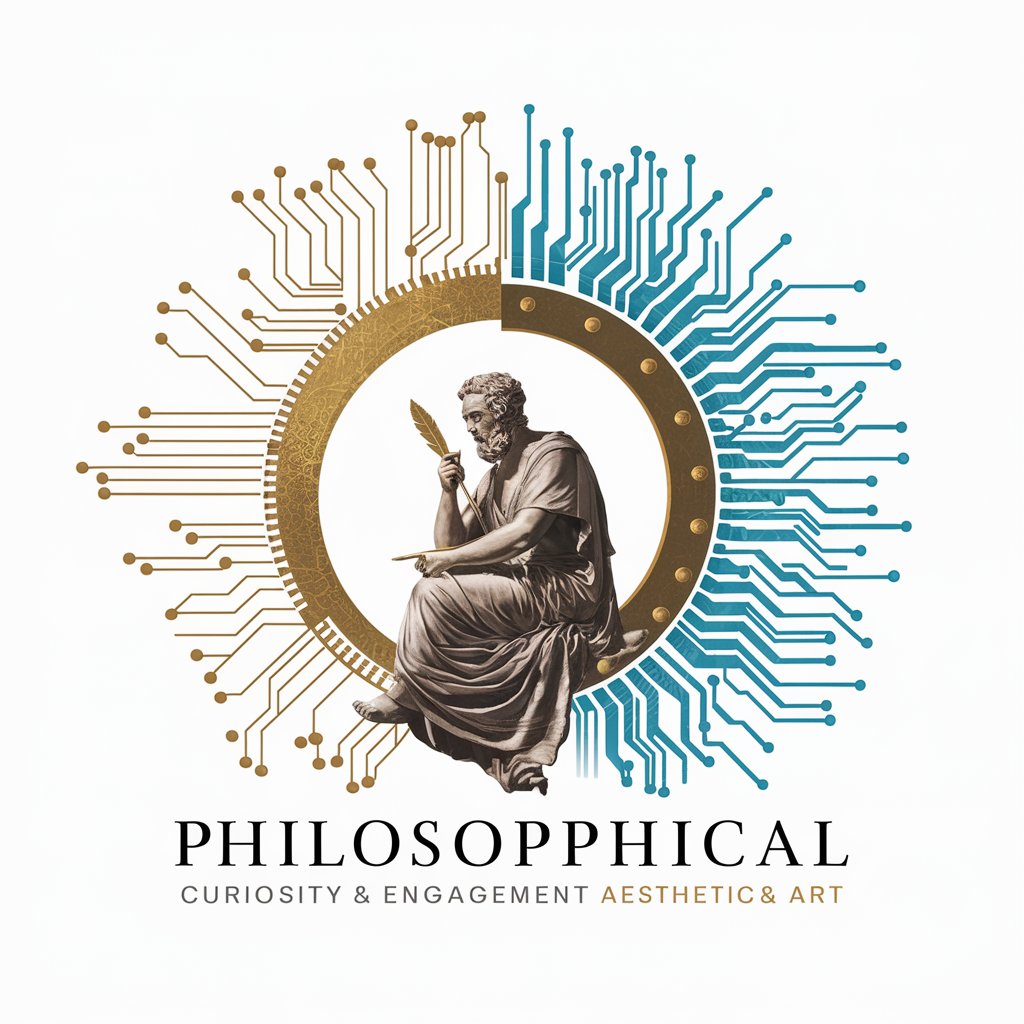
Story Weaver
AI-Powered Immersive Storytelling
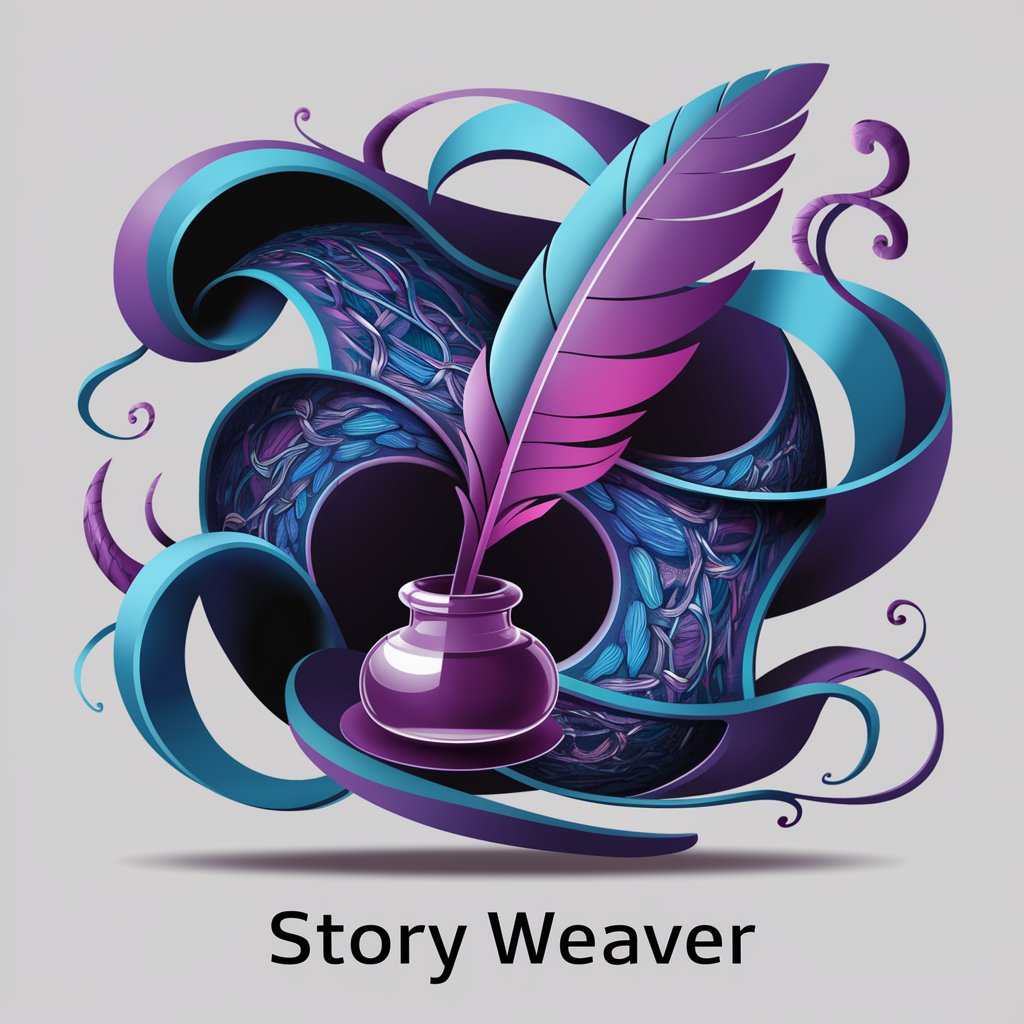
Recipe Visualizer
Bringing recipes to life with AI
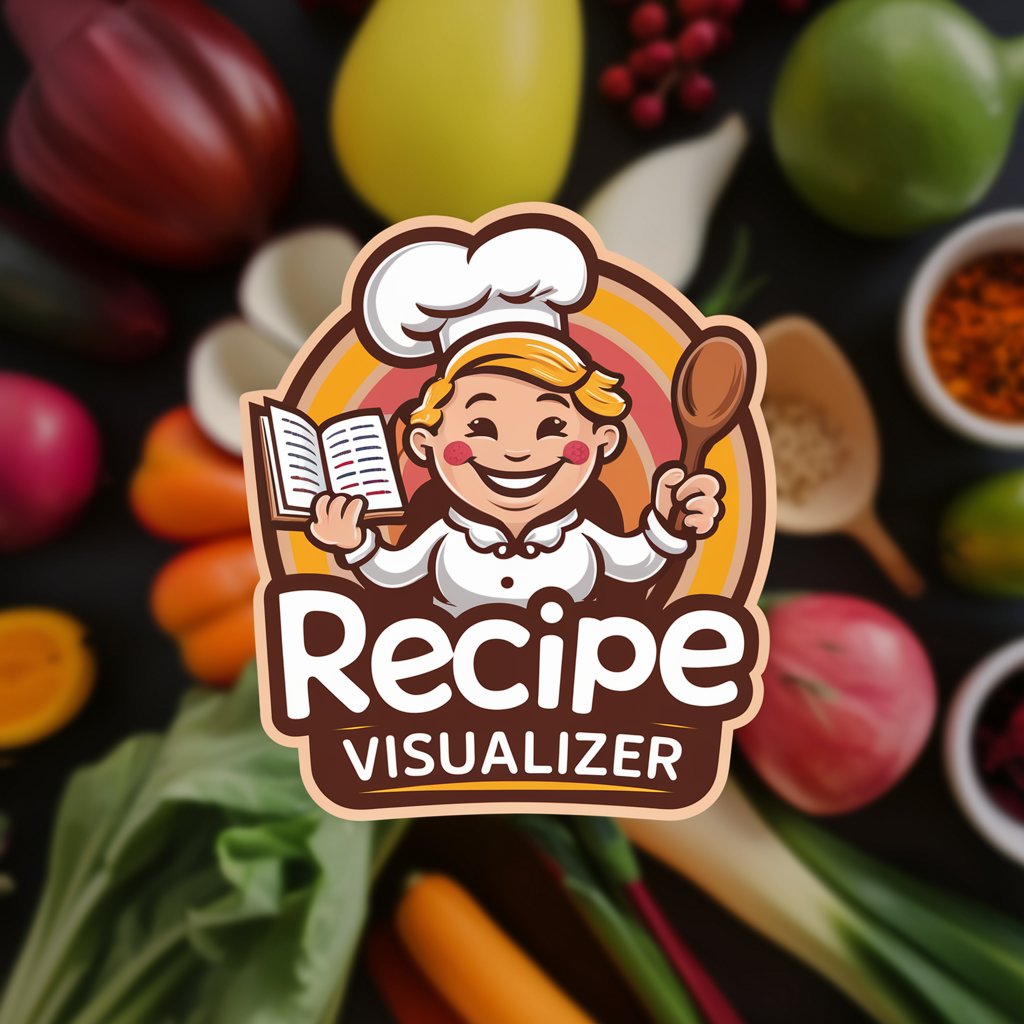
children's bedtime picture book production version
Bringing bedtime stories to life with AI
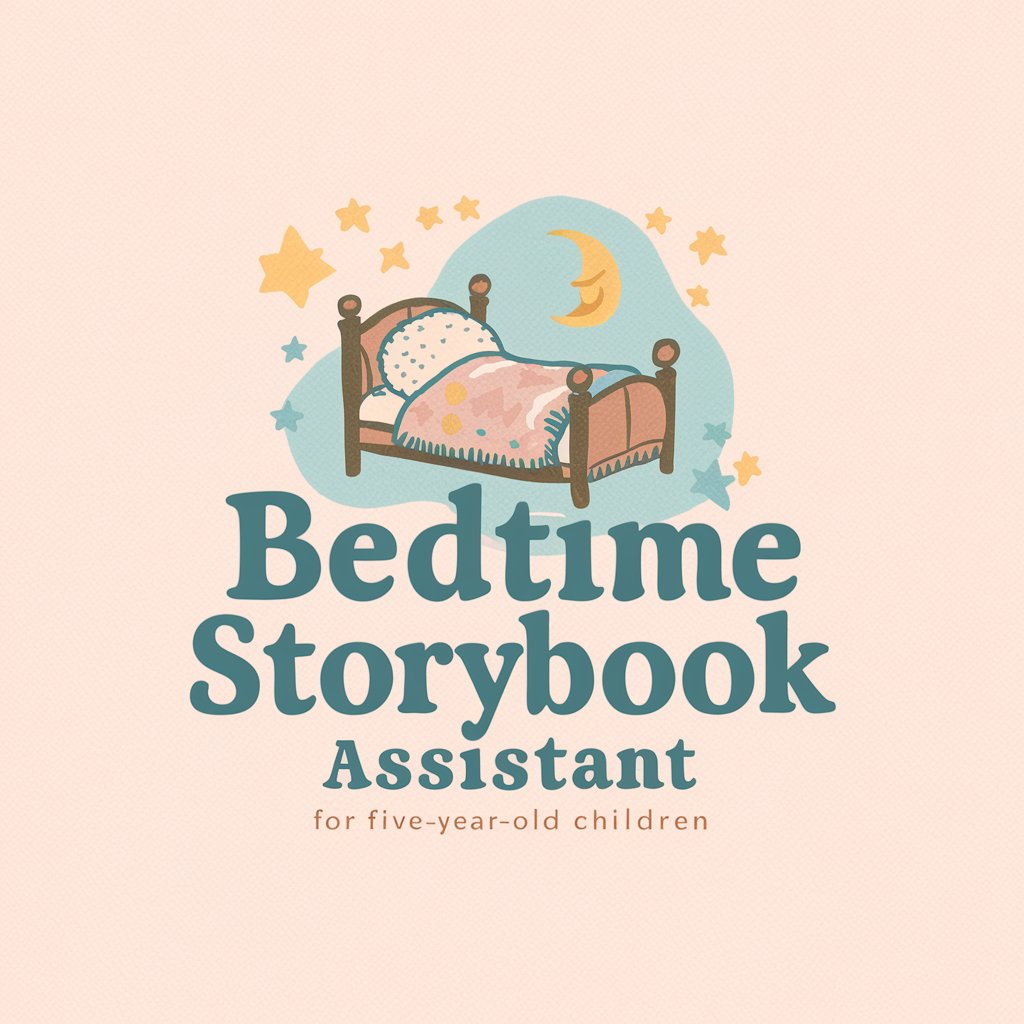
Eco Kazakhstan Pathfinder AI
Empowering sustainable travel with AI
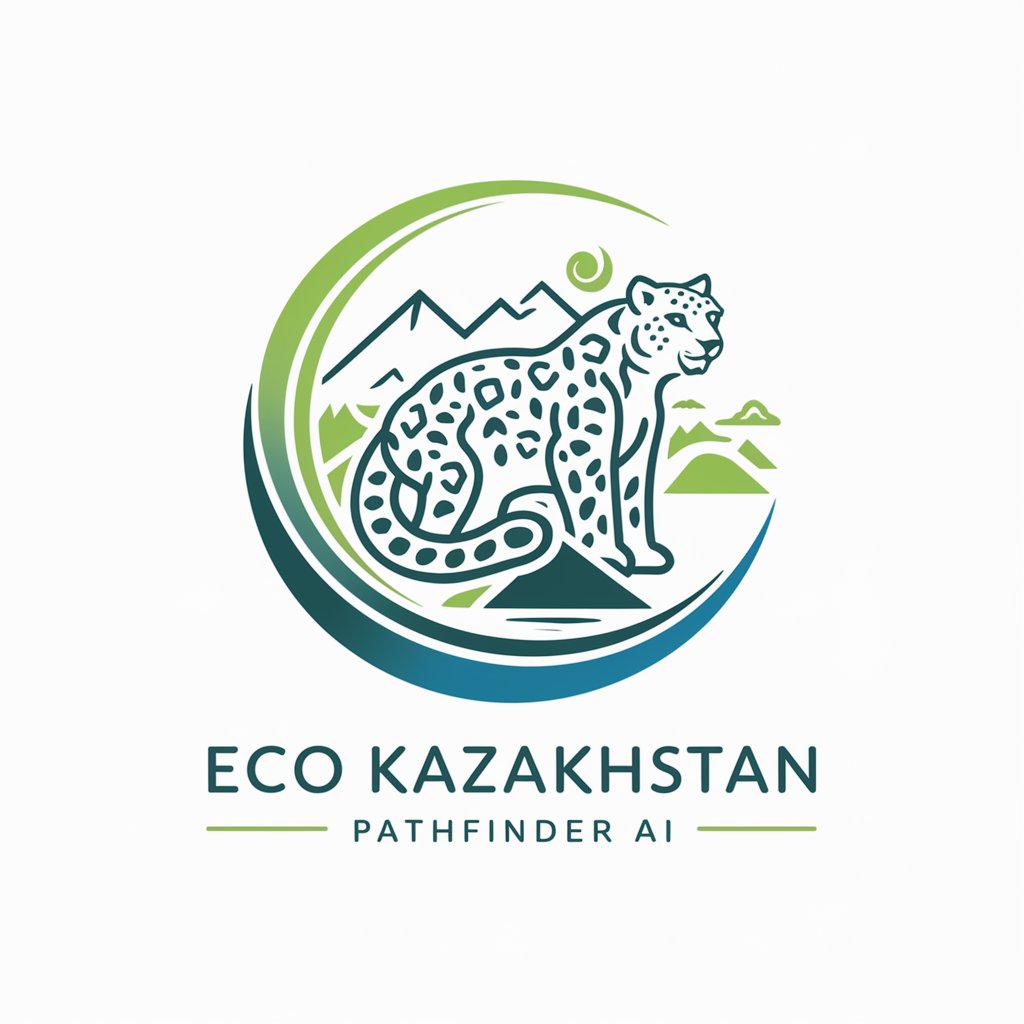
Wedding Dress
AI-Powered Bridal Fashion Illustrations
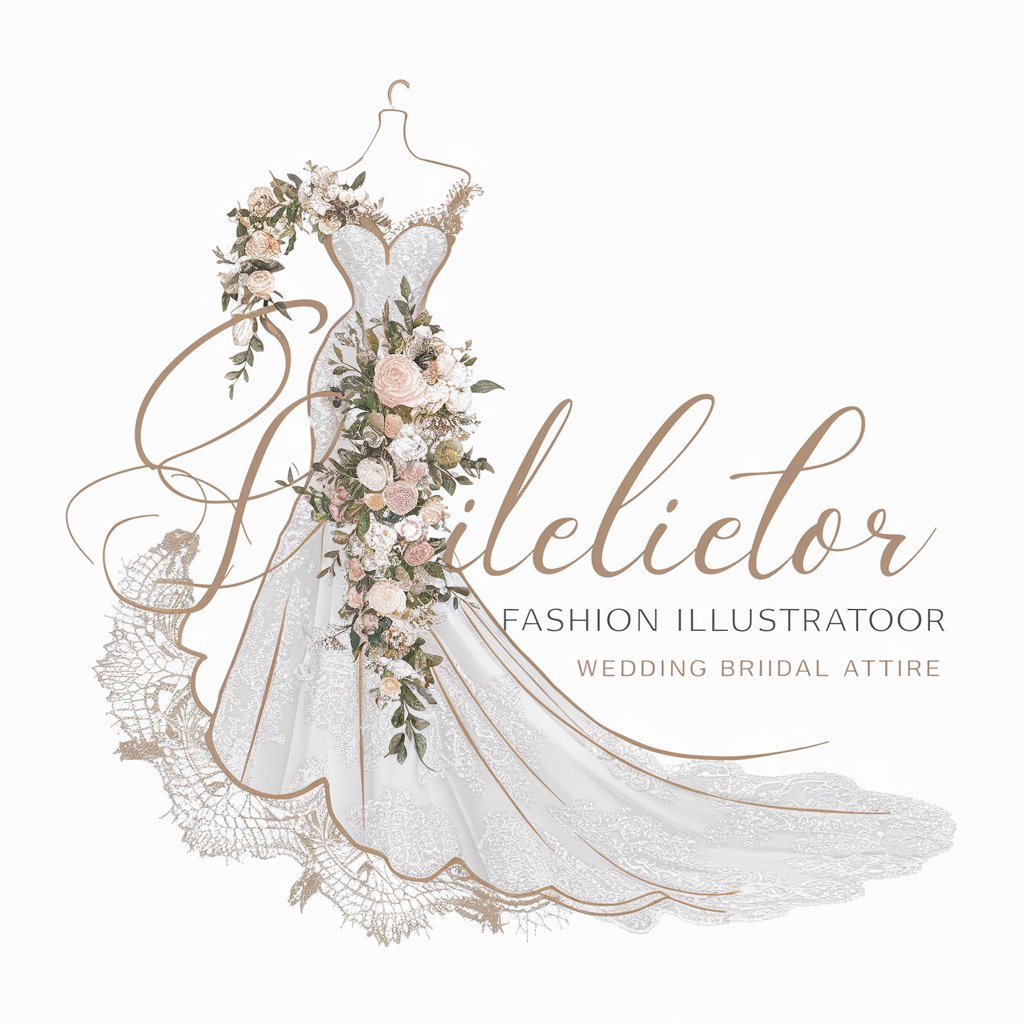
Elf Magic Maker
Bringing festive cheer through AI-powered elf mischief
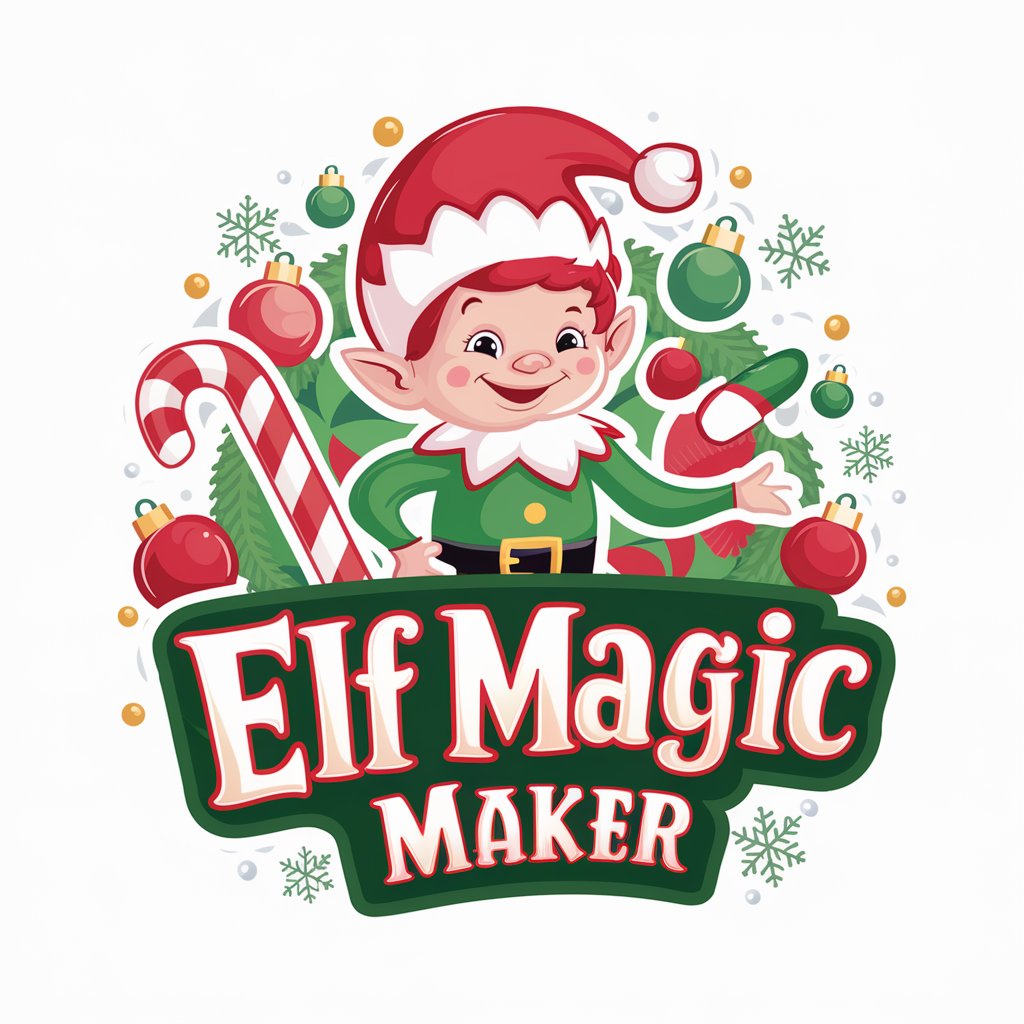
Expert Valorant
Elevate Your Game with AI-Powered Valorant Insights
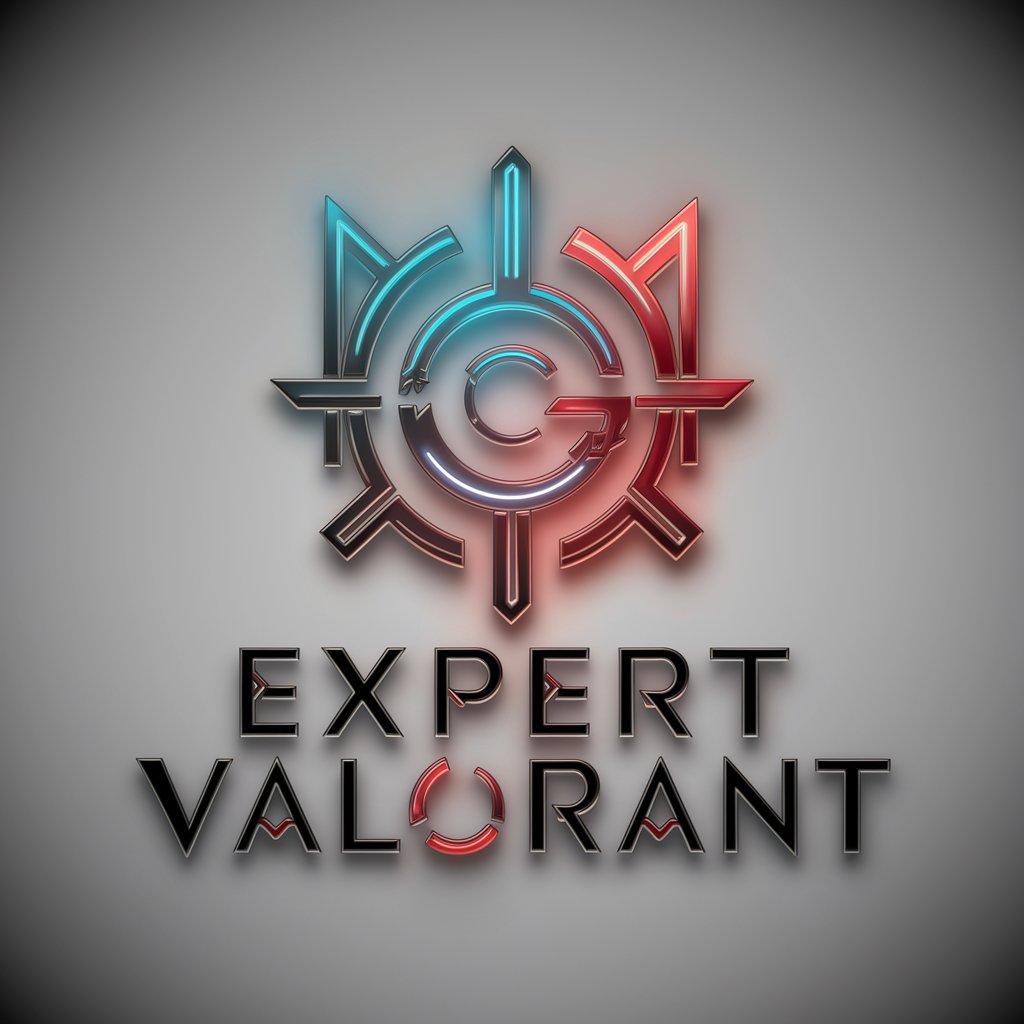
Morse Code Expert
Translate text and Morse code effortlessly.
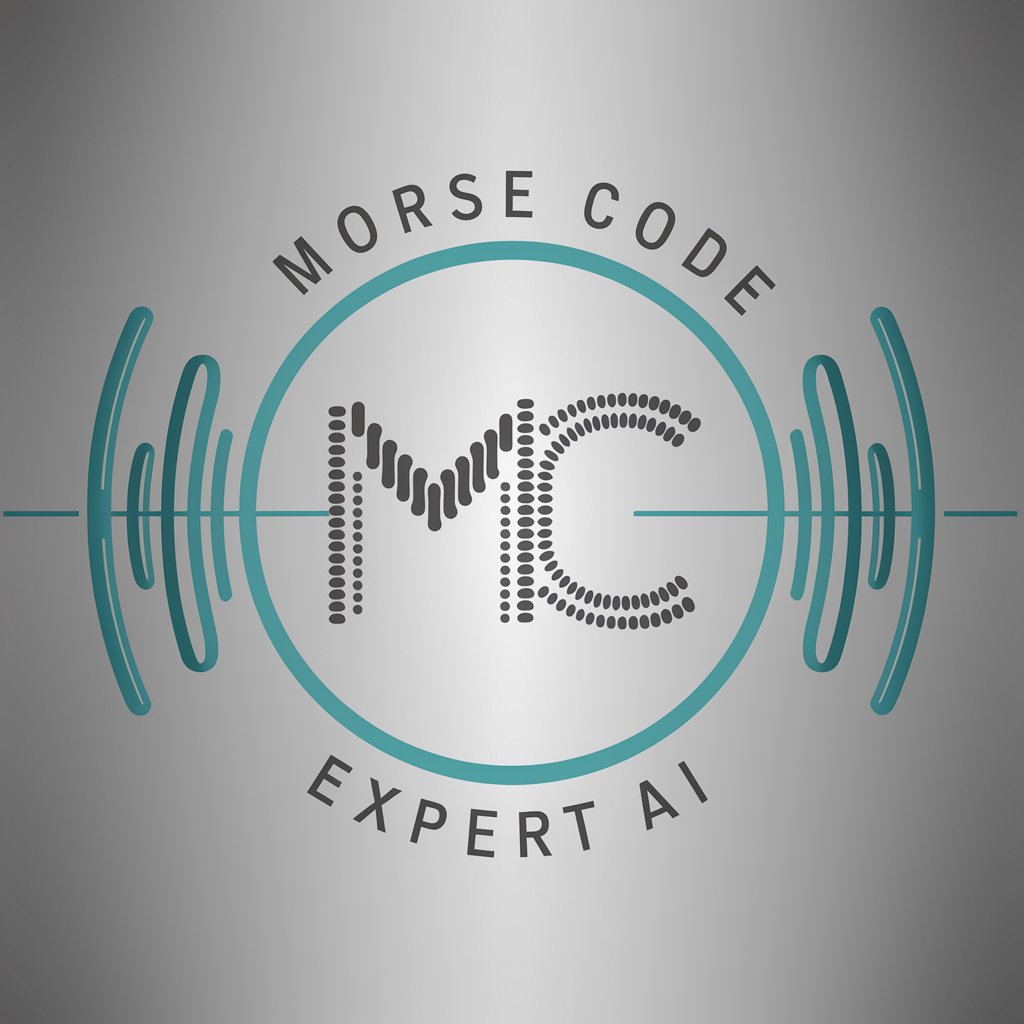
Consejero Scrum
Elevate Your Scrum with AI
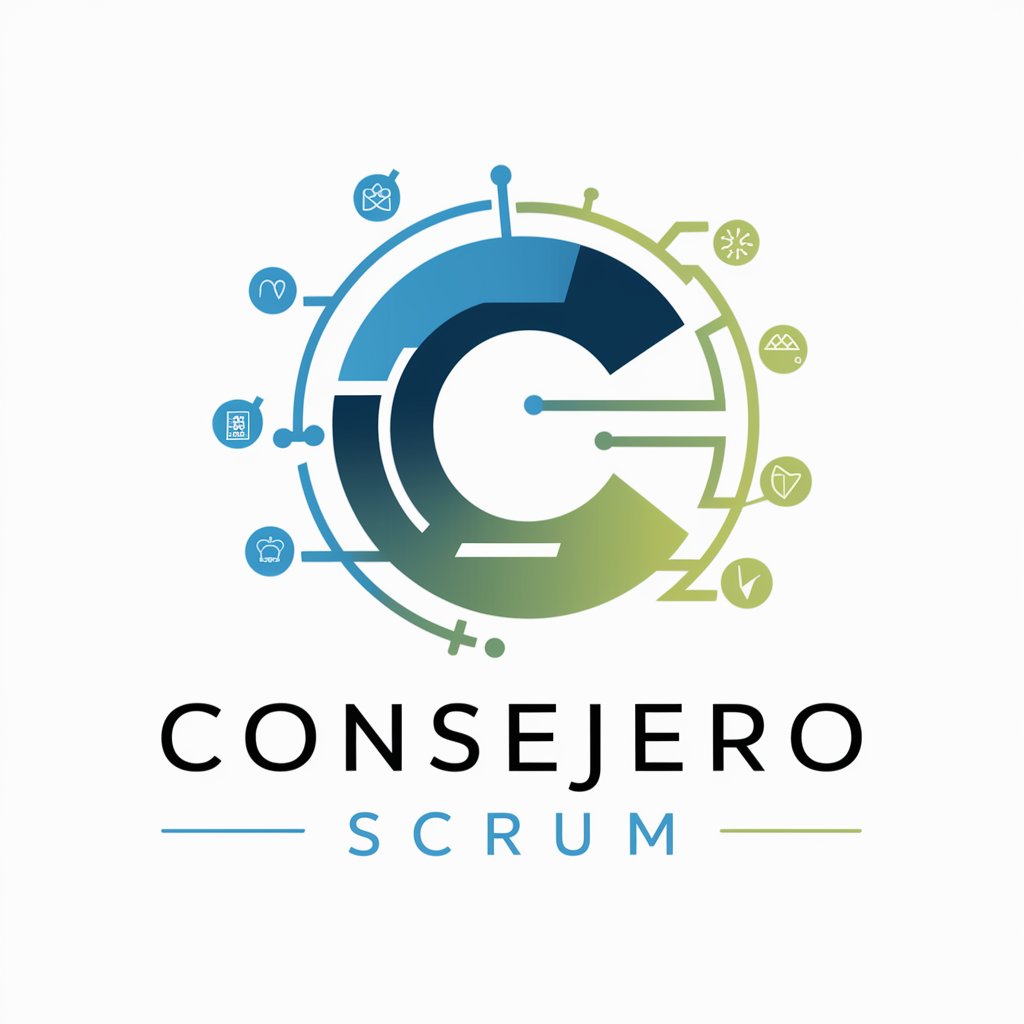
Caricaturise me by AIMania.one
Bringing Your Portraits to Life with AI
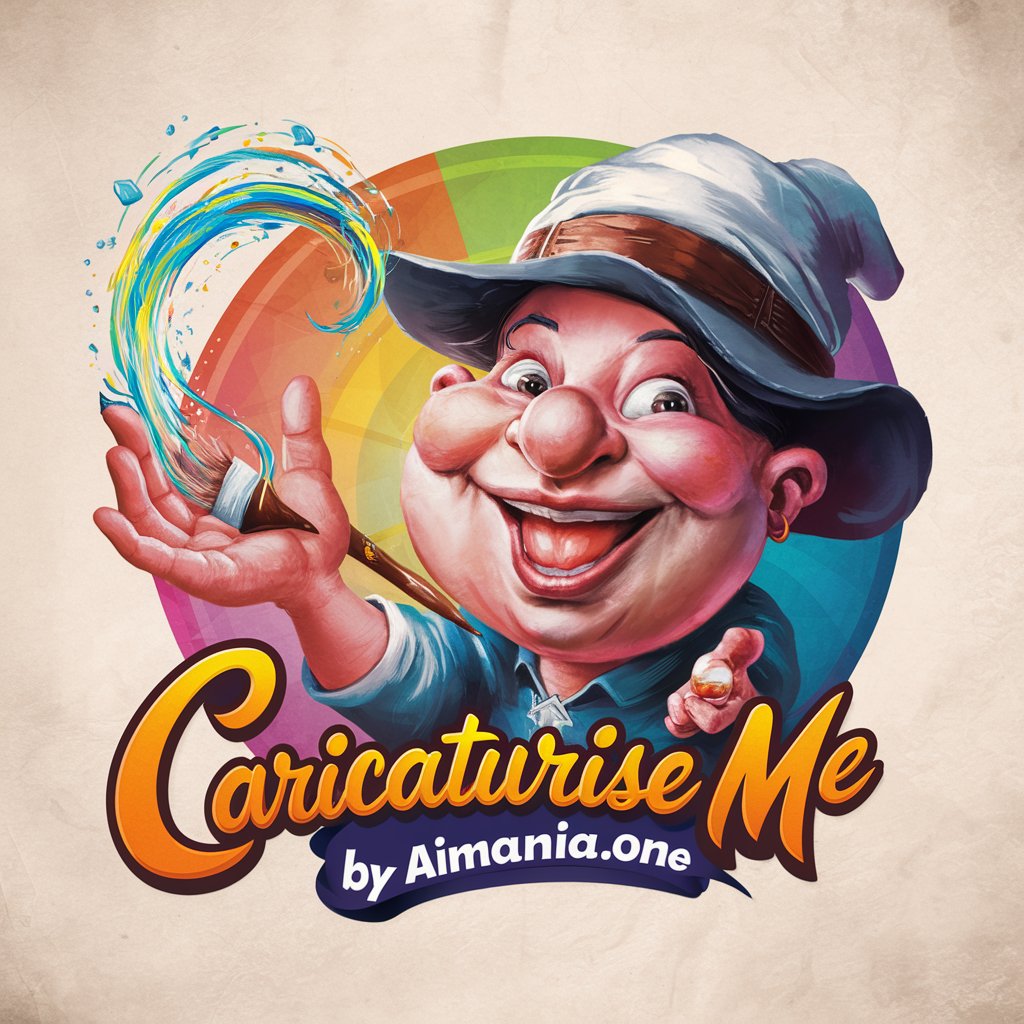
Self-Assembling Neural Network FAQs
What is a Self-Assembling Neural Network?
A Self-Assembling Neural Network is an advanced form of artificial neural network that autonomously organizes and structures itself to improve computational efficiency and adapt to new data without human intervention, drawing inspiration from biological neural networks.
How do Self-Assembling Neural Networks differ from traditional neural networks?
Unlike traditional neural networks that require predefined architectures, self-assembling neural networks dynamically adjust their structure, including neuron connections and layers, based on the data they process, leading to more adaptable and efficient learning.
Can Self-Assembling Neural Networks adapt to new, unforeseen data?
Yes, one of the key features of self-assembling neural networks is their ability to adapt to new and unforeseen data by reconfiguring their structure, making them highly effective for dynamic and evolving datasets.
What are the applications of Self-Assembling Neural Networks?
These networks are used in a variety of fields, including but not limited to, robotics, autonomous systems, adaptive computing systems, complex problem solving, and advanced data analysis.
What are the challenges in working with Self-Assembling Neural Networks?
Key challenges include the computational complexity of dynamically adjusting network structures, ensuring stable and efficient learning processes, and developing methodologies to effectively evaluate and interpret the self-organized architectures.